Documents
Presentation Slides
DEEP LEARNING FOR FRAME ERROR PROBABILITY PREDICTION IN BICM-OFDM SYSTEMS
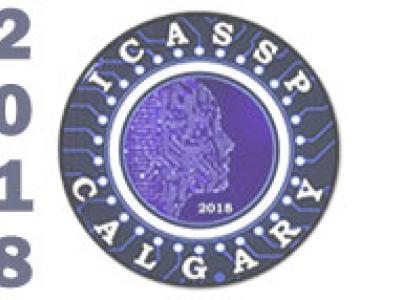
- Citation Author(s):
- Submitted by:
- Vidit Saxena
- Last updated:
- 19 April 2018 - 5:17pm
- Document Type:
- Presentation Slides
- Document Year:
- 2018
- Event:
- Presenters:
- Vidit Saxena
- Paper Code:
- 4286
- Categories:
- Log in to post comments
In the context of wireless communications, we propose a deep learning approach to learn the mapping from the instantaneous state of a frequency selective fading channel to the corresponding frame error probability (FEP) for an arbitrary set of transmission parameters. We propose an abstract model of a bit interleaved coded modulation (BICM) orthogonal frequency division multiplexing (OFDM) link chain and show that the maximum likelihood (ML) estimator of the model parameters estimates the true FEP distribution. Further, we exploit deep neural networks as a general purpose tool to implement our model and propose a training scheme for which, even while training with the binary frame error events (i.e., ACKs / NACKs), the network outputs converge to the FEP conditioned on the input channel state. We provide simulation results that demonstrate gains in the FEP prediction accuracy with our approach as compared to the traditional effective exponential SIR metric (EESM) approach for a range of channel code rates, and show that these gains can be exploited to increase the link throughput.