Documents
Poster
DEEP MULTIMODAL LEARNING FOR EMOTION RECOGNITION IN SPOKEN LANGUAGE
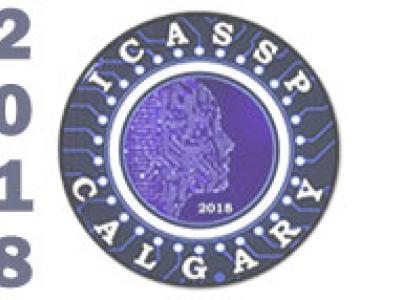
- Citation Author(s):
- Submitted by:
- Yue Gu
- Last updated:
- 13 April 2018 - 3:30pm
- Document Type:
- Poster
- Document Year:
- 2018
- Event:
- Presenters:
- Yue Gu
- Paper Code:
- 3738
- Categories:
- Log in to post comments
In this paper, we present a novel deep multimodal framework to predict human emotions based on sentence-level spoken language. Our architecture has two distinctive characteristics. First, it extracts the high-level features from both text and audio via a hybrid deep multimodal structure, which considers the spatial information from text, temporal information from audio, and high-level associations from low-level handcrafted features. Second, we fuse all features by using a three-layer deep neural network to learn the correlations across modalities and train the feature extraction and fusion modules together, allowing optimal global fine-tuning of the entire structure. We evaluated the proposed framework on the IEMOCAP dataset. Our result shows promising performance, achieving 60.4% in weighted accuracy for five emotion categories.