- Human Spoken Language Acquisition, Development and Learning (SLP-LADL)
- Language Modeling, for Speech and SLP (SLP-LANG)
- Machine Translation of Speech (SLP-SSMT)
- Speech Data Mining (SLP-DM)
- Speech Retrieval (SLP-IR)
- Spoken and Multimodal Dialog Systems and Applications (SLP-SMMD)
- Spoken language resources and annotation (SLP-REAN)
- Spoken Language Understanding (SLP-UNDE)
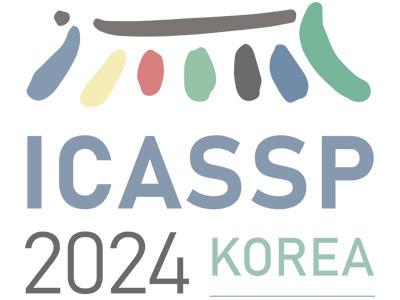
- Read more about Towards ASR robust spoken language understanding through in-context learning with word confusion networks
- Log in to post comments
In the realm of spoken language understanding (SLU), numerous natural language understanding (NLU) methodologies have been adapted by supplying large language models (LLMs) with transcribed speech instead of conventional written text. In real-world scenarios, prior to input into an LLM, an automated speech recognition (ASR) system generates an output transcript hypothesis, where inherent errors can degrade subsequent SLU tasks.
- Categories:
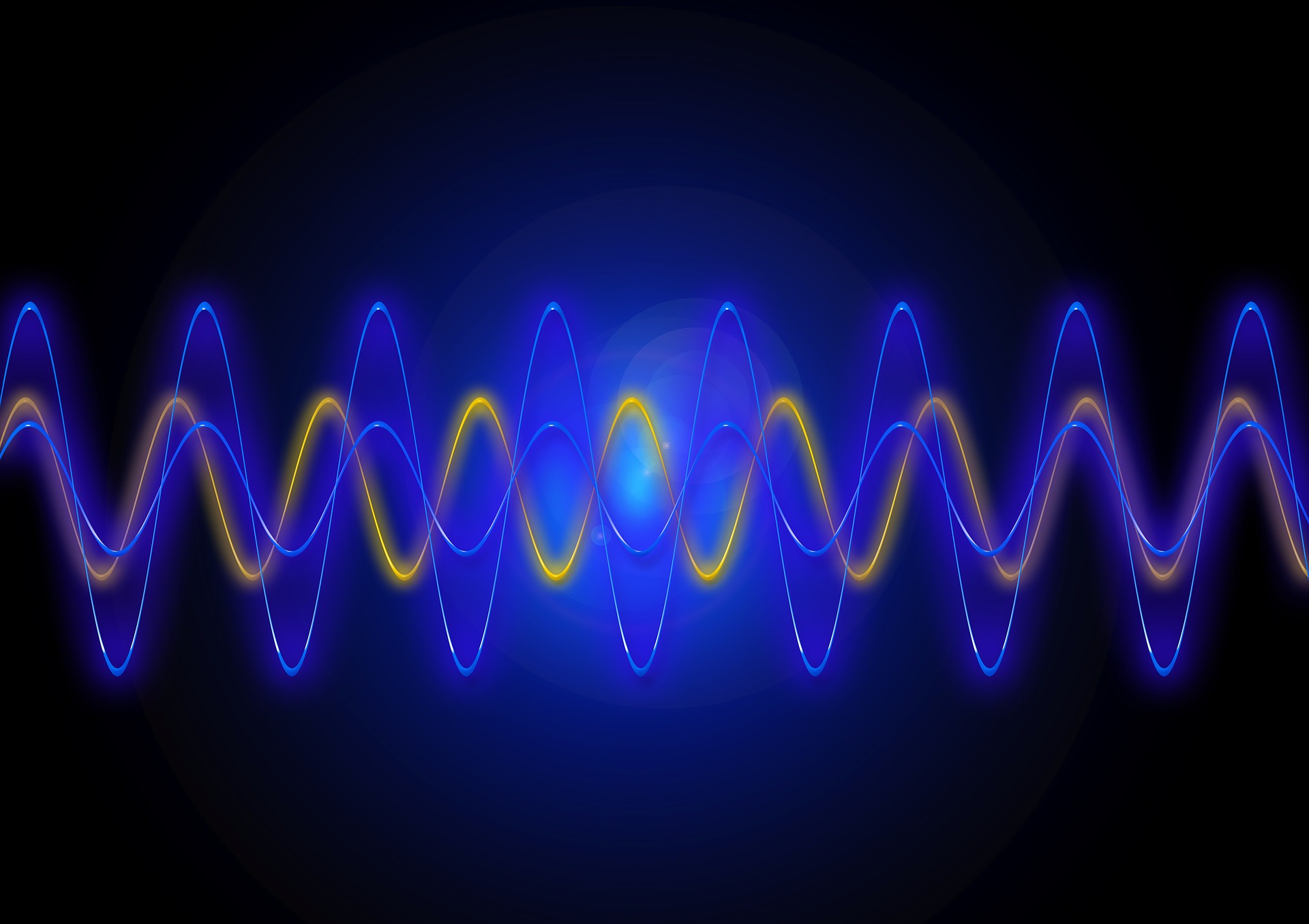
- Read more about AUGSUMM: TOWARDS GENERALIZABLE SPEECH SUMMARIZATION USING SYNTHETIC LABELS FROM LARGE LANGUAGE MODELS
- Log in to post comments
Abstractive speech summarization (SSUM) aims to generate humanlike summaries from speech. Given variations in information captured
and phrasing, recordings can be summarized in multiple ways. Therefore, it is more reasonable to consider a probabilistic distribution
of all potential summaries rather than a single summary. However, conventional SSUM models are mostly trained and evaluated
with a single ground-truth (GT) human-annotated deterministic summary for every recording. Generating multiple human references
- Categories:
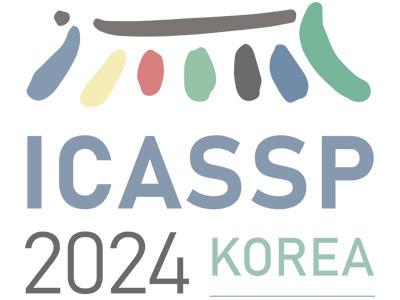
- Read more about END-TO-END SPEECH RECOGNITION CONTEXTUALIZATION WITH LARGE LANGUAGE MODELS
- Log in to post comments
In recent years, Large Language Models (LLMs) have garnered significant attention from the research community due to their exceptional performance and generalization capabilities. In this paper, we introduce a novel method for contextualizing speech recognition models incorporating LLMs. Our approach casts speech recognition as a mixed-modal language modeling task based on a pretrained LLM. We provide audio features, along with optional text tokens for context, to train the system to complete transcriptions in a decoderonly fashion.
- Categories:
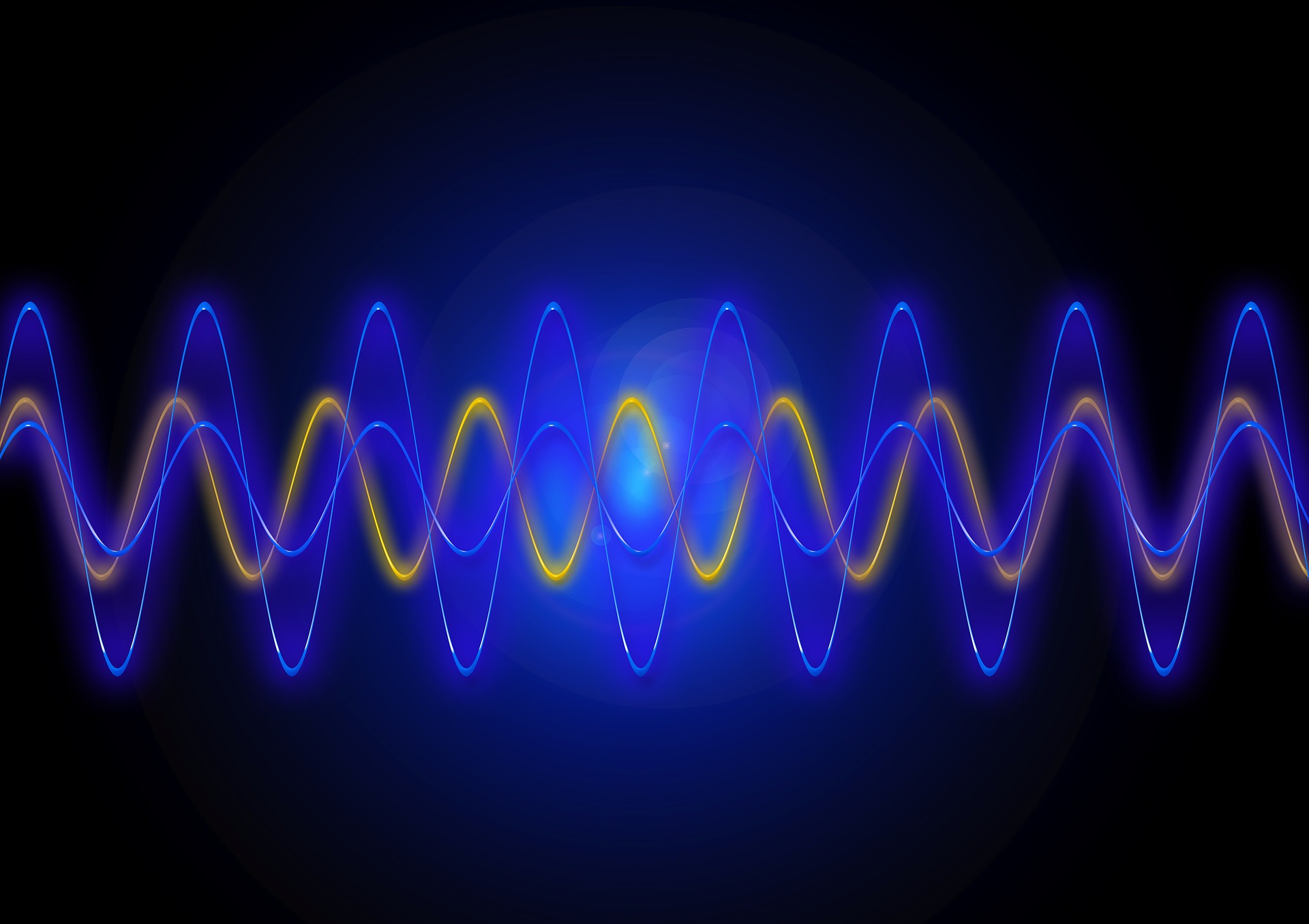
- Read more about Improving Medical Dialogue Generation with Abstract Meaning Representations
- Log in to post comments
Medical Dialogue Generation plays a critical role in telemedicine by facilitating the dissemination of medical expertise to patients. Existing studies focus on incorporating textual representations, which have limited their ability to represent text semantics, such as ignoring important medical entities.
- Categories:

- Read more about Self-supervised Speaker Verification with Adaptive Threshold and Hierarchical Training
- 1 comment
- Log in to post comments
This is a poster material of recent research accepted by IEEE ICASSP 2024.
Title: SELF-SUPERVISED SPEAKER VERIFICATION WITH ADAPTIVE THRESHOLD AND HIERARCHICAL TRAINING
For more inforamation, please check out the publication at IEEE Xplore:
https://ieeexplore.ieee.org/document/10448455
- Categories:
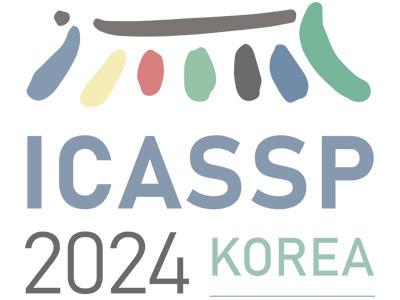
- Read more about Improving ASR Contextual Biasing using Guided Attention Loss
- Log in to post comments
In this paper, we propose a Guided Attention (GA) auxiliary training loss, which improves the effectiveness and robustness of automatic speech recognition (ASR) contextual biasing without introducing additional parameters. A common challenge in previous literature is that the word error rate (WER) reduction brought by contextual biasing diminishes as the number of bias phrases increases. To address this challenge, we employ a GA loss as an additional training objective besides the Transducer loss.
- Categories:
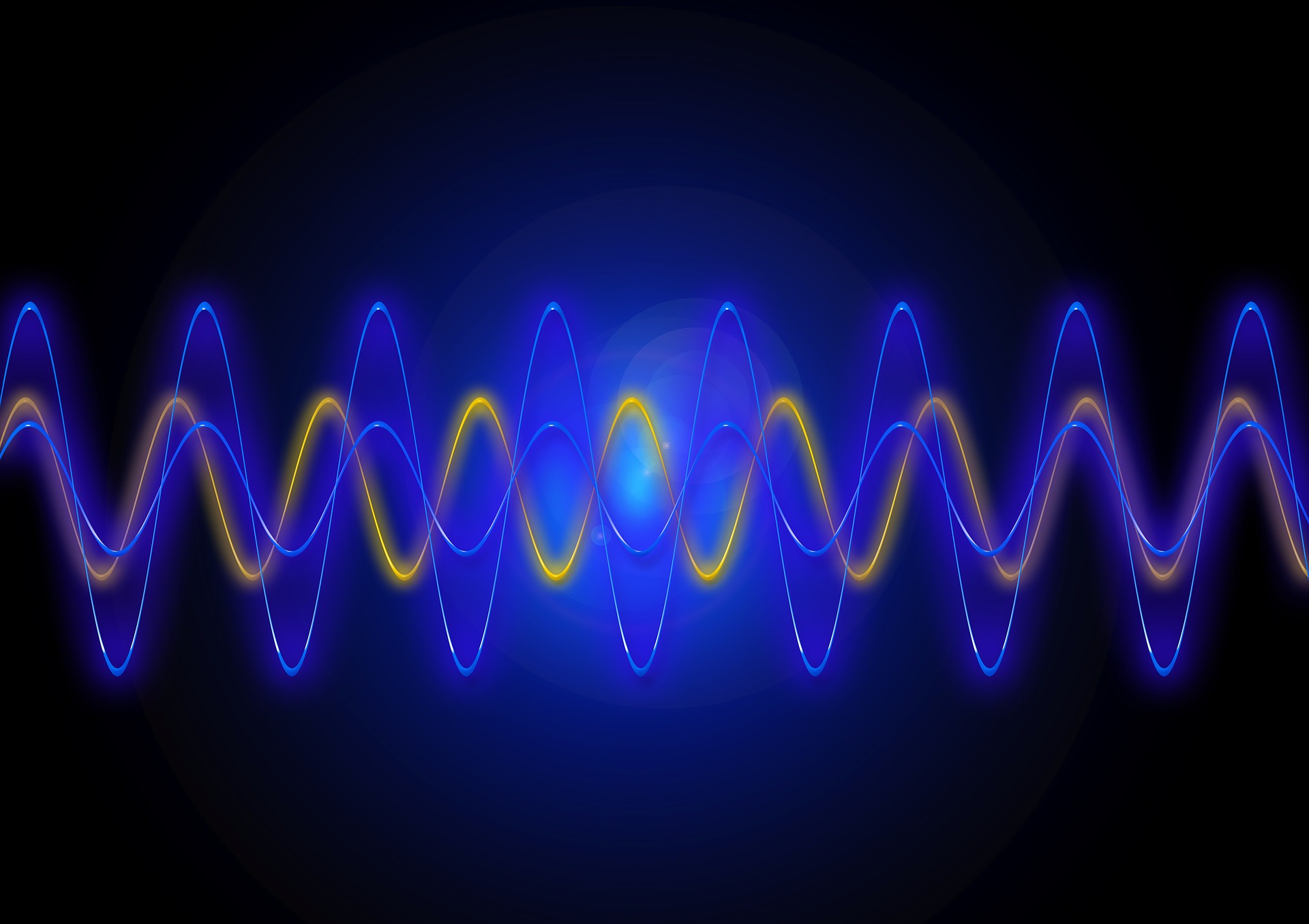
- Read more about CONCSS: CONTRASTIVE-BASED CONTEXT COMPREHENSION FOR DIALOGUE-APPROPRIATE PROSODY IN CONVERSATIONAL SPEECH SYNTHESIS
- 1 comment
- Log in to post comments
Conversational speech synthesis (CSS) incorporates historical dialogue as supplementary information with the aim of generating speech that has dialogue-appropriate prosody. While previous methods have already delved into enhancing context comprehension, context representation still lacks effective representation capabilities and context-sensitive discriminability. In this paper, we introduce a contrastive learning-based CSS framework, CONCSS.
- Categories:
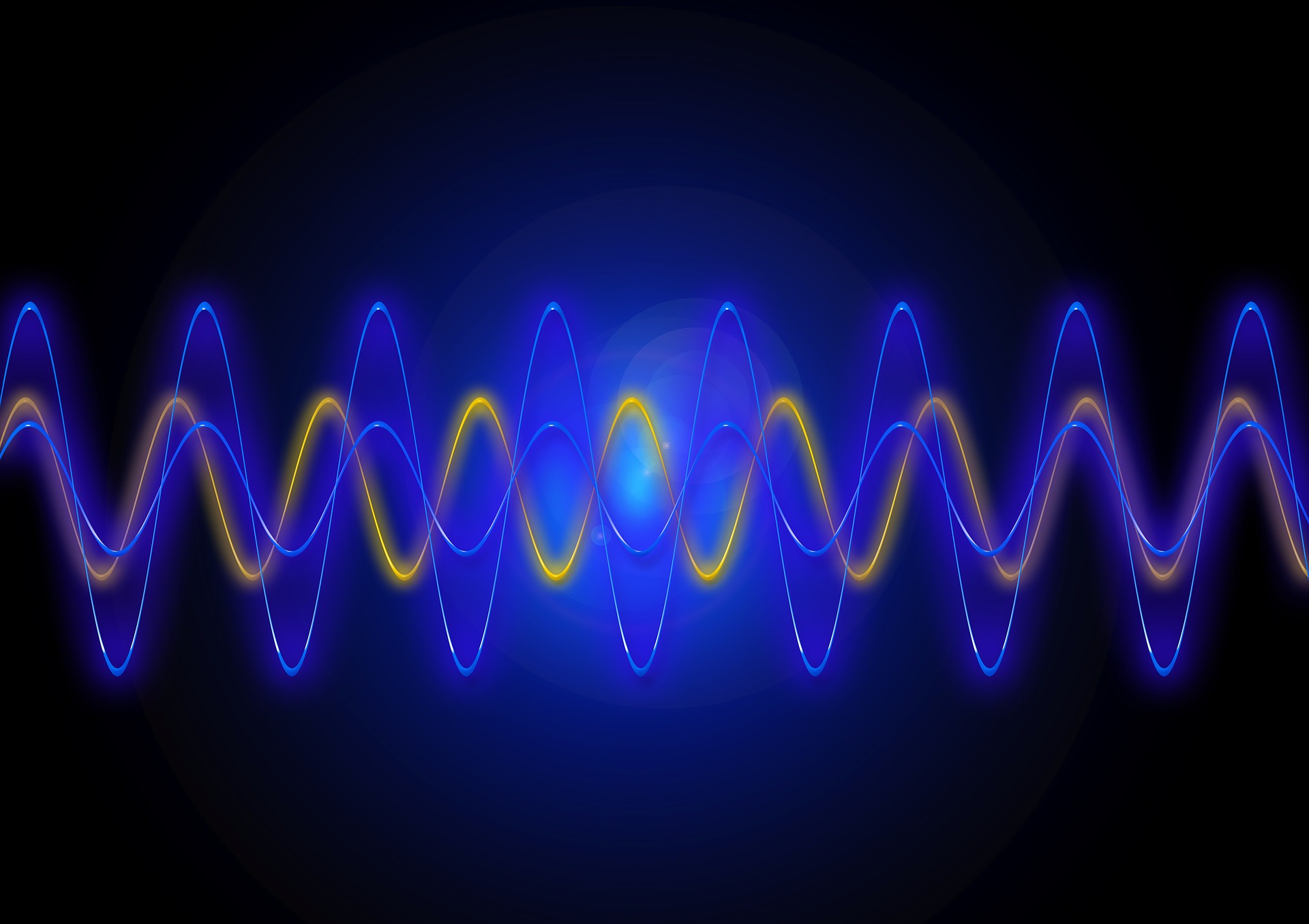
- Read more about TOWARDS CONTROLLED TABLE-TO-TEXT GENERATION WITH SCIENTIFIC REASONING
- Log in to post comments
The sheer volume of scientific experimental results and complex technical statements, often presented in tabular formats, presents a formidable barrier to individuals acquiring preferred information. The realms of scientific reasoning and content generation that adhere to user preferences encounter distinct challenges. In this work, we present a new task for generating fluent and logical descriptions that match user preferences over scientific tabular data, aiming to automate scientific document analysis.
- Categories:
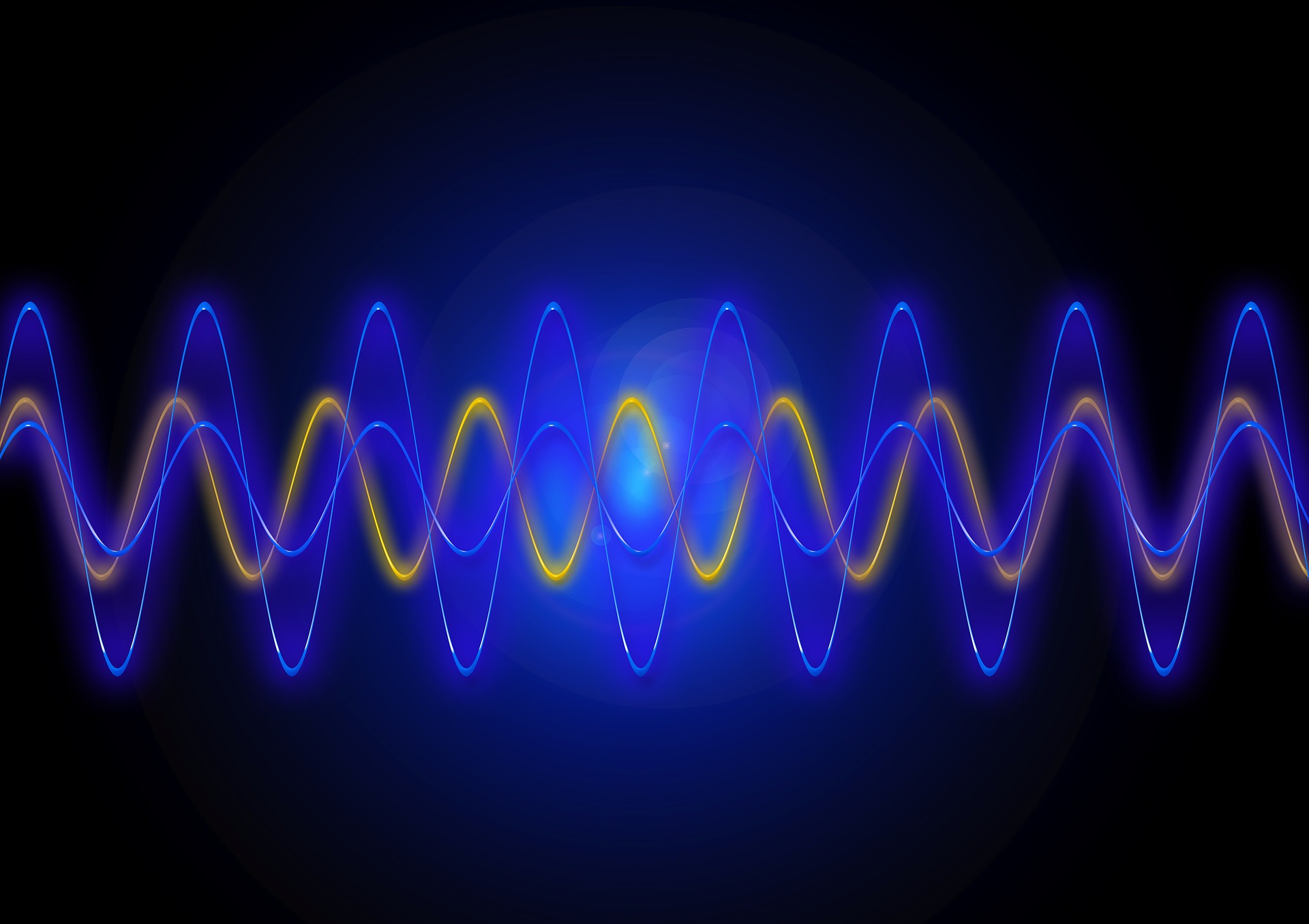
- Read more about SPEECH COLLAGE: CODE-SWITCHED AUDIO GENERATION BY COLLAGING MONOLINGUAL CORPORA
- Log in to post comments
Designing effective automatic speech recognition (ASR) systems for Code-Switching (CS) often depends on the availability of the tran- scribed CS resources. To address data scarcity, this paper introduces Speech Collage, a method that synthesizes CS data from mono- lingual corpora by splicing audio segments. We further improve the smoothness quality of audio generation using an overlap-add approach. We investigate the impact of generated data on speech recognition in two scenarios: using in-domain CS text and a zero- shot approach with synthesized CS text.
- Categories:
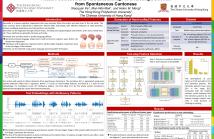
- Read more about Feature Selection and Text Embedding For Detecting Dementia from Spontaneous Cantonese
- Log in to post comments
Dementia is a severe cognitive impairment that affects the health of older adults and creates a burden on their families and caretakers. This paper analyzes diverse hand-crafted features extracted from spoken languages and selects the most discriminative ones for dementia detection. Recently, the performance of dementia detection has been significantly improved by utilizing Transformer-based models that automatically capture the structural and linguistic properties of spoken languages. We investigate Transformer-based features and propose an end-to-end system for dementia detection.
ICASSP2023.pdf

- Categories: