Documents
Poster
DEEP TRANSFER LEARNING FOR EEG-BASED BRAIN COMPUTER INTERFACE
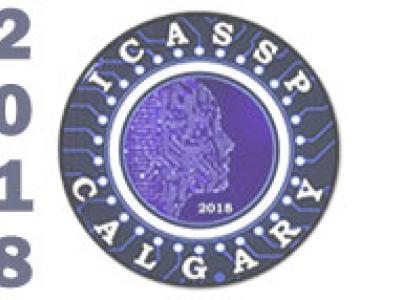
- Citation Author(s):
- Submitted by:
- chuanqi tan
- Last updated:
- 12 April 2018 - 11:40am
- Document Type:
- Poster
- Document Year:
- 2018
- Event:
- Presenters:
- Chuanqi Tan
- Paper Code:
- 1200
- Categories:
- Log in to post comments
The electroencephalography classifier is the most important component of brain-computer interface based systems. There are two major problems hindering the improvement of it. First, traditional methods do not fully exploit multimodal information. Second, large-scale annotated EEG datasets are almost impossible to acquire because biological data acquisition is challenging and quality annotation is costly. Herein, we propose a novel deep transfer learning approach to solve these two problems. First, we model cognitive events based on EEG data by characterizing the data using EEG optical flow, which is designed to preserve multimodal EEG information in a uniform representation. Second, we design a deep transfer learning framework which is suitable for transferring knowledge by joint training, which contains a adversarial network and a special loss function. The experiments demonstrate that our approach, when applied to EEG classification tasks, has many advantages, such as robustness and accuracy.