- Bioimaging and microscopy
- Bioinformatics
- Biomedical signal processing
- Medical image analysis
- Medical imaging
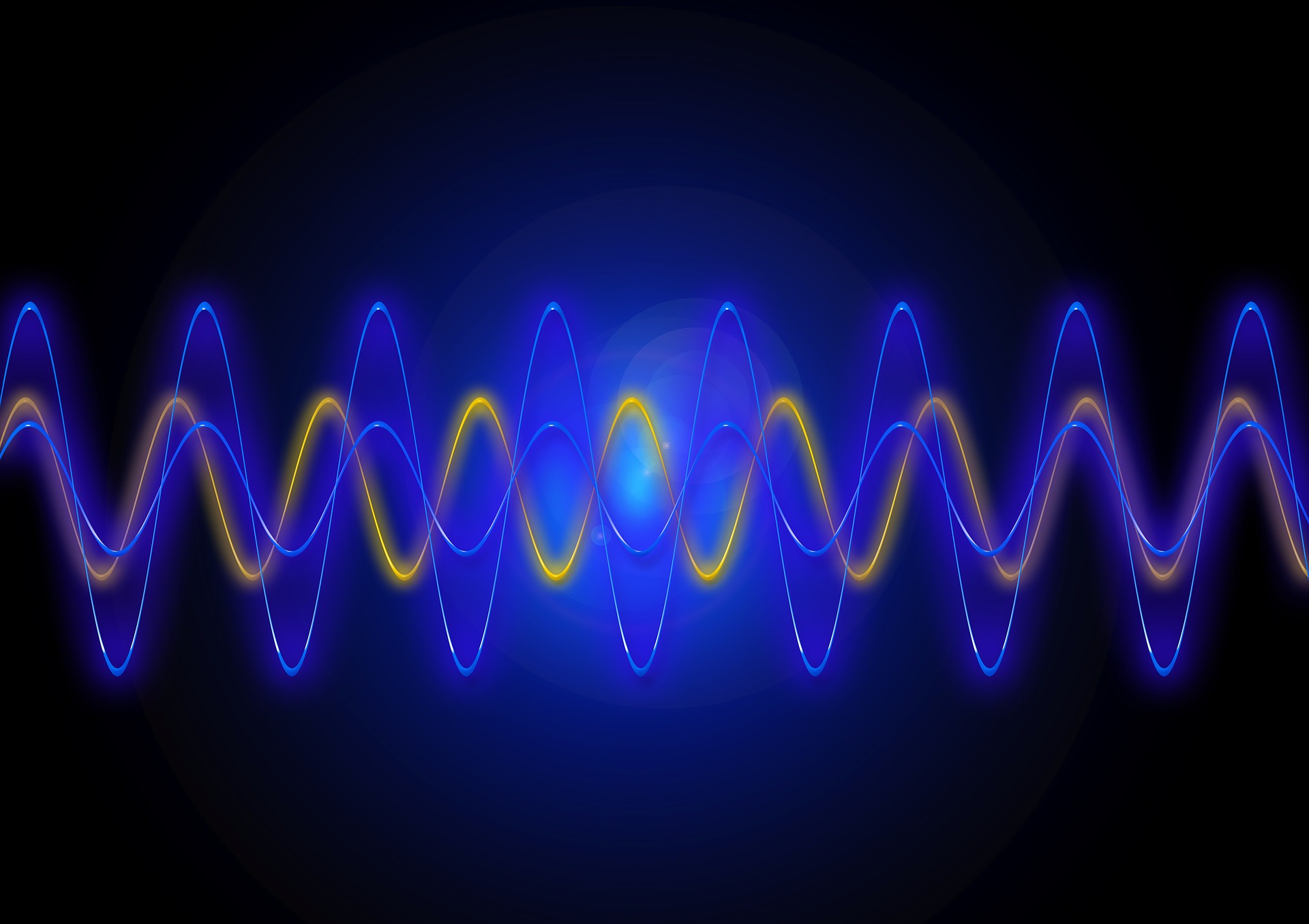
- Read more about Paper_8423_Xin_Zhu
- Log in to post comments
Electroencephalogram (EEG) data compression is necessary for wireless recording applications to reduce the amount of data that needs to be transmitted. In this paper, an asymmetrical sparse autoencoder with a discrete cosine transform (DCT) layer is proposed to compress EEG signals. The encoder module of the autoencoder has a combination of a fully connected linear layer and the DCT layer to reduce redundant data using hard-thresholding nonlinearity.
- Categories:
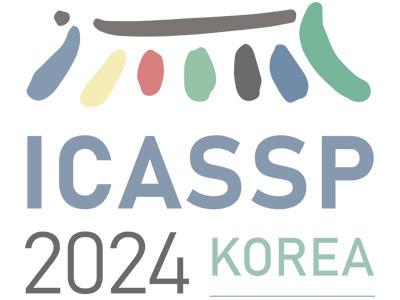
- Read more about ELECTROENCEPHALOGRAM SENSOR DATA COMPRESSION USING AN ASYMMETRICAL SPARSE AUTOENCODER WITH A DISCRETE COSINE TRANSFORM LAYER
- Log in to post comments
Electroencephalogram (EEG) data compression is necessary for wireless recording applications to reduce the amount of data that needs to be transmitted. In this paper, an asymmetrical sparse autoencoder with a discrete cosine transform (DCT) layer is proposed to compress EEG signals. The encoder module of the autoencoder has a combination of a fully connected linear layer and the DCT layer to reduce redundant data using hard-thresholding nonlinearity.
- Categories:
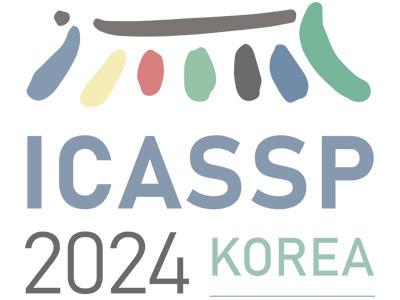
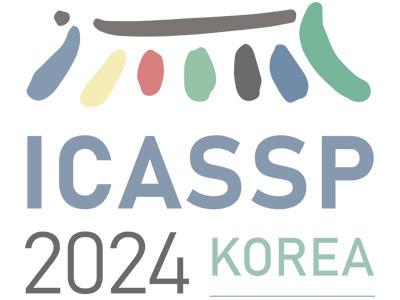
- Read more about CROSS-ATTENTION-GUIDED WAVENET FOR MEL SPECTROGRAM RECONSTRUCTION IN THE ICASSP 2024 AUDITORY EEG CHALLENGE slide
- Log in to post comments
upload slide
- Categories:
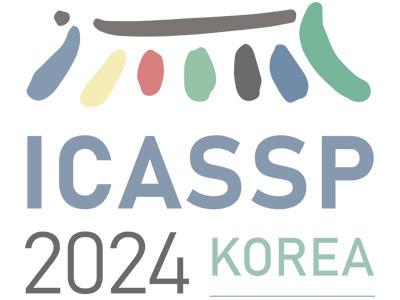
- Read more about SELF-SUPERVISED LEARNING FOR SLEEP STAGE CLASSIFICATION WITH TEMPORALAUGMENTATION AND FALSE NEGATIVE SUPPRESSION
- Log in to post comments
Self-supervised learning has been gaining attention in the field of sleep stage classification. It learns representations with unlabeled electroencephalography (EEG) signals, which alleviates the cost of labeling for specialists. However, most self-supervised approaches assume only the two augmented views from the same EEG sample is a positive pair, which suffers from the false negative problem. Therefore, we propose a new model named Temporal Augmentation and False Negative Suppression (TA-FNS) to solve the problem. Specifically, it first generates two augmented views for each EEG sample.
Poster.pdf

- Categories:
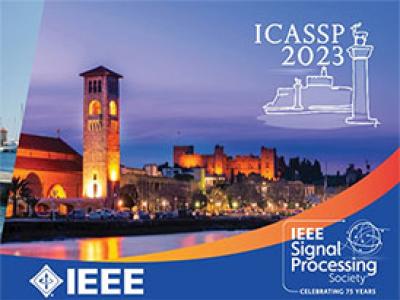
- Read more about BRAIN STRUCTURE-FUNCTION INTERACTION NETWORK FOR FLUID COGNITION PREDICTION
- Log in to post comments
Predicting fluid cognition via neuroimaging data is essential for understanding the neural mechanisms underlying various complex cognitions in the human brain. Both brain functional connectivity (FC) and structural connectivity (SC) provide distinct neural mechanisms for fluid cognition. In addition, interactions between SC and FC within distributed association regions are related to improvements in fluid cognition. However, existing learning-based methods that leverage both modality-specific embeddings and high-order interactions between the two modalities for prediction are scarce.
- Categories:
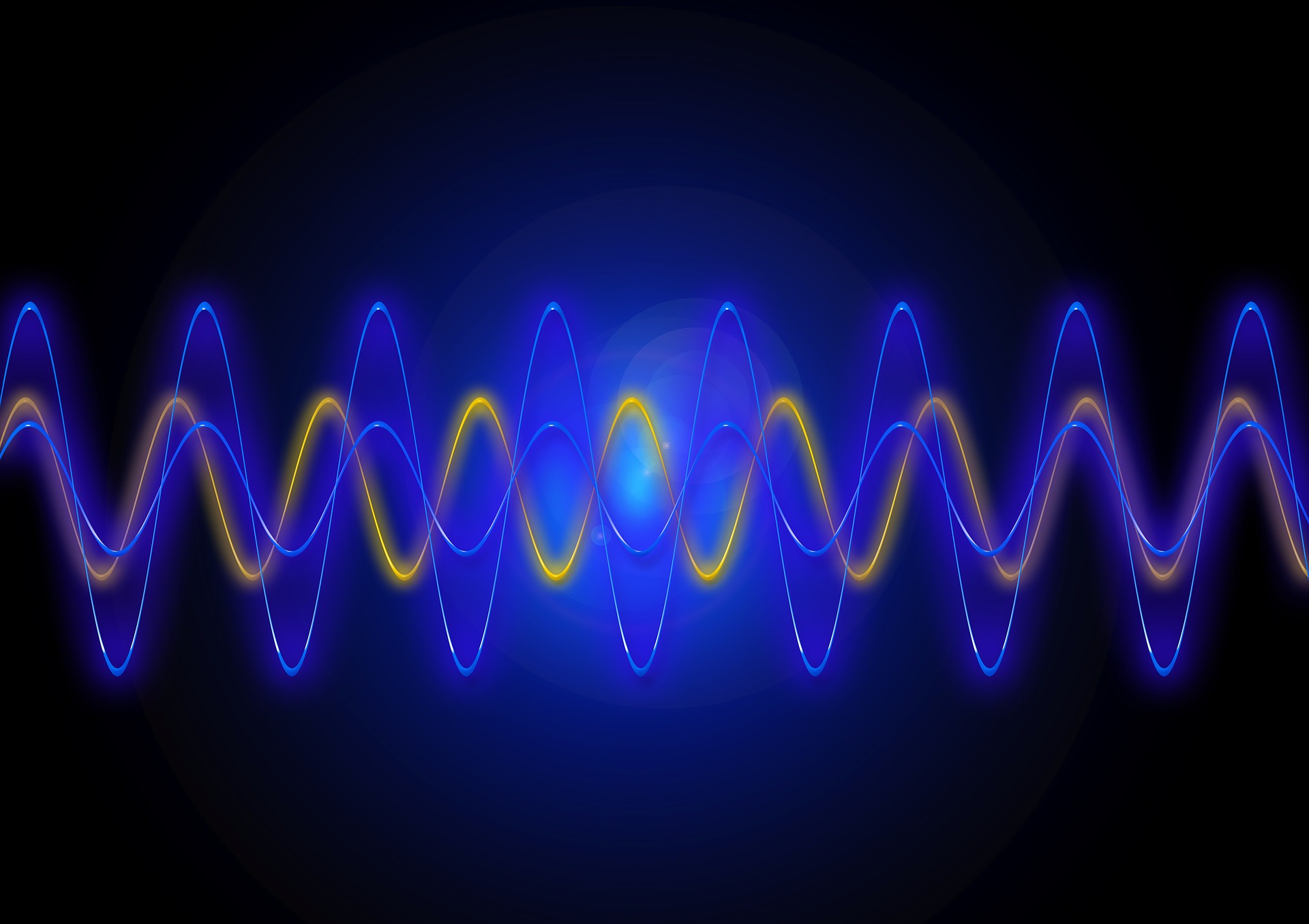
- Read more about HEALTHY AGING IS MARKED BY ENTROPY REDUCTION IN CORTICAL SPONTANEOUS ACTIVITY
- Log in to post comments
Entropy trajectories remain unclear for the aging process of human brain system. We employed the open data from an accelerated longitudinal cohort (PREVENT-AD) including 24 healthy aging participants followed by 4 years with 5 visits per participant to establish the brain entropy aging curves and distinguish with the age and cohort effects. This reveals that global cortical entropy decreased with aging while a significant cohort effect was detectable that people who were born earlier showed higher entropy.
- Categories:
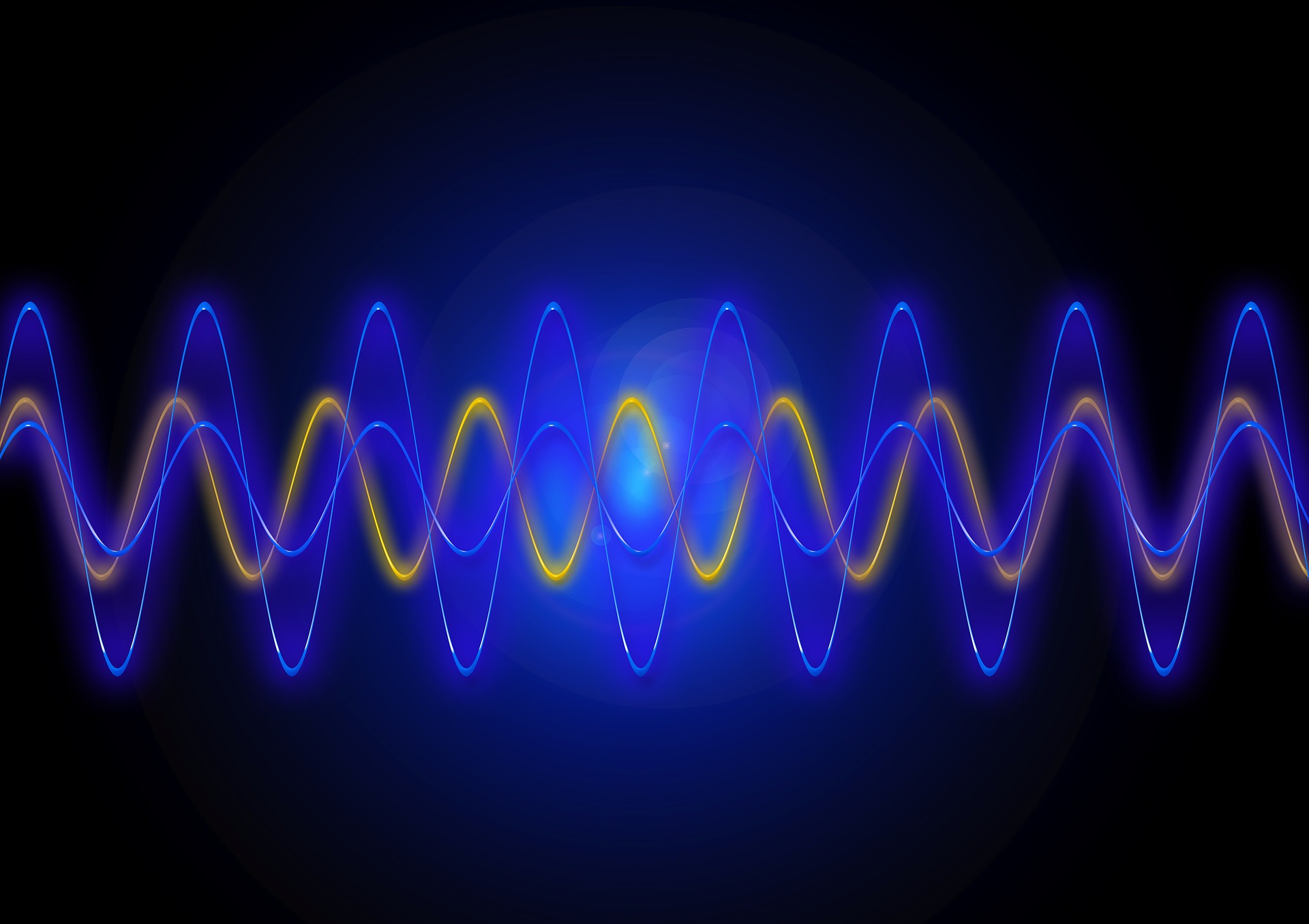
- Read more about CALSeg: Improving Calibration of Medical Image Segmentation with Variational Label Smoothing
- Log in to post comments
In practical medical image segmentation tasks, ensuring confidence calibration is crucial. However, medical image segmentation typically relies on hard labels (one-hot vectors), and
when minimizing the cross-entropy loss, the model’s softmax predictions are compelled to align with hard labels, resulting in over-confident predictions. To alleviate above problems, this study proposes a novel framework on calibration of medical image segmentation, called CALSeg. The Variational Label Smoothing (VLS) method is innovatively proposed, which
poster.pdf

- Categories:
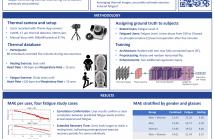
- Read more about Estimating exercise-induced fatigue from thermal facial images
- Log in to post comments
Exercise-induced fatigue resulting from physical activity can
be an early indicator of overtraining, illness, or other health
issues. In this article, we present an automated method for
estimating exercise-induced fatigue levels through the use of
thermal imaging and facial analysis techniques utilizing deep
learning models. Leveraging a novel dataset comprising over
400,000 thermal facial images of rested and fatigued users,
our results suggest that exercise-induced fatigue levels could
- Categories:
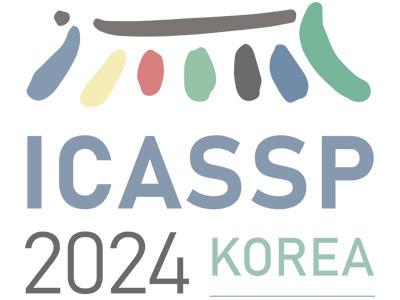
- Read more about UNCERTAINTY-GUIDED PERSON SEARCH MODEL WITH AUXILIARY SHALLOW FEATURE EXPLORATION
- Log in to post comments
Person search is a unified system aimed at jointly localizing and identifying a person of interest from a gallery of whole scene images. Due to the inherent properties of the person search, it faces significant challenges of large-scale variations, inaccurate detection boxes, and crowded scenes. To address these issues, we proposed an uncertainty-guided framework coupled with auxiliary shallow feature exploration, which includes a shallow feature fusion module and an uncertaintyguided module.
- Categories: