Documents
Presentation Slides
Deep-URL
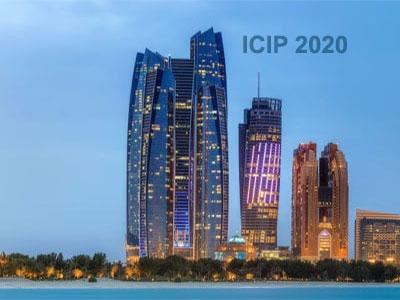
- Citation Author(s):
- Submitted by:
- Chirag Agarwal
- Last updated:
- 2 November 2020 - 10:54am
- Document Type:
- Presentation Slides
- Document Year:
- 2020
- Event:
- Presenters:
- Chirag Agarwal
- Paper Code:
- 1782
- Categories:
- Keywords:
- Log in to post comments
The lack of interpretability in current deep learning models causes serious concerns as they are extensively used for various life-critical applications. Hence, it is of paramount importance to develop interpretable deep learning models. In this paper, we consider the problem of blind deconvolution and propose a novel model-aware deep architecture that allows for the recovery of both the blur kernel and the sharp image from the blurred image. In particular, we propose the Deep Unfolded Richardson-Lucy (Deep-URL) framework -- an interpretable deep-learning architecture that can be seen as an amalgamation of classical estimation technique and deep neural network, and consequently leads to improved performance. Our numerical investigations demonstrate significant improvement compared to state-of-the-art algorithms.