- Image/Video Storage, Retrieval
- Image/Video Processing
- Image/Video Coding
- Image Scanning, Display, and Printing
- Image Formation
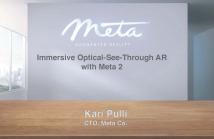
- Read more about Immersive Optical-See-Through Augmented Reality (Keynote Talk)
- Log in to post comments
Immersive Optical-See-Through Augmented Reality. Augmented Reality has been getting ready for the last 20 years, and is finally becoming real, powered by progress in enabling technologies such as graphics, vision, sensors, and displays. In this talk I’ll provide a personal retrospective on my journey, working on all those enablers, getting ready for the coming AR revolution. At Meta, we are working on immersive optical-see-through AR headset, as well as the full software stack. We’ll discuss the differences of optical vs.
- Categories:
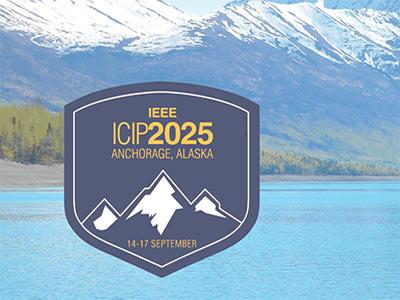
- Read more about Texture- and Shape-based Adversarial Attacks for Overhead Image Vehicle Detection
- Log in to post comments
Detecting vehicles in aerial images is difficult due to complex backgrounds, small object sizes, shadows, and occlusions. Although recent deep learning advancements have improved object detection, these models remain susceptible to adversarial attacks (AAs), challenging their reliability. Traditional AA strategies often ignore practical implementation constraints. Our work proposes realistic and practical constraints on texture (lowering resolution, limiting modified areas, and color ranges) and analyzes the impact of shape modifications on attack performance.
- Categories:
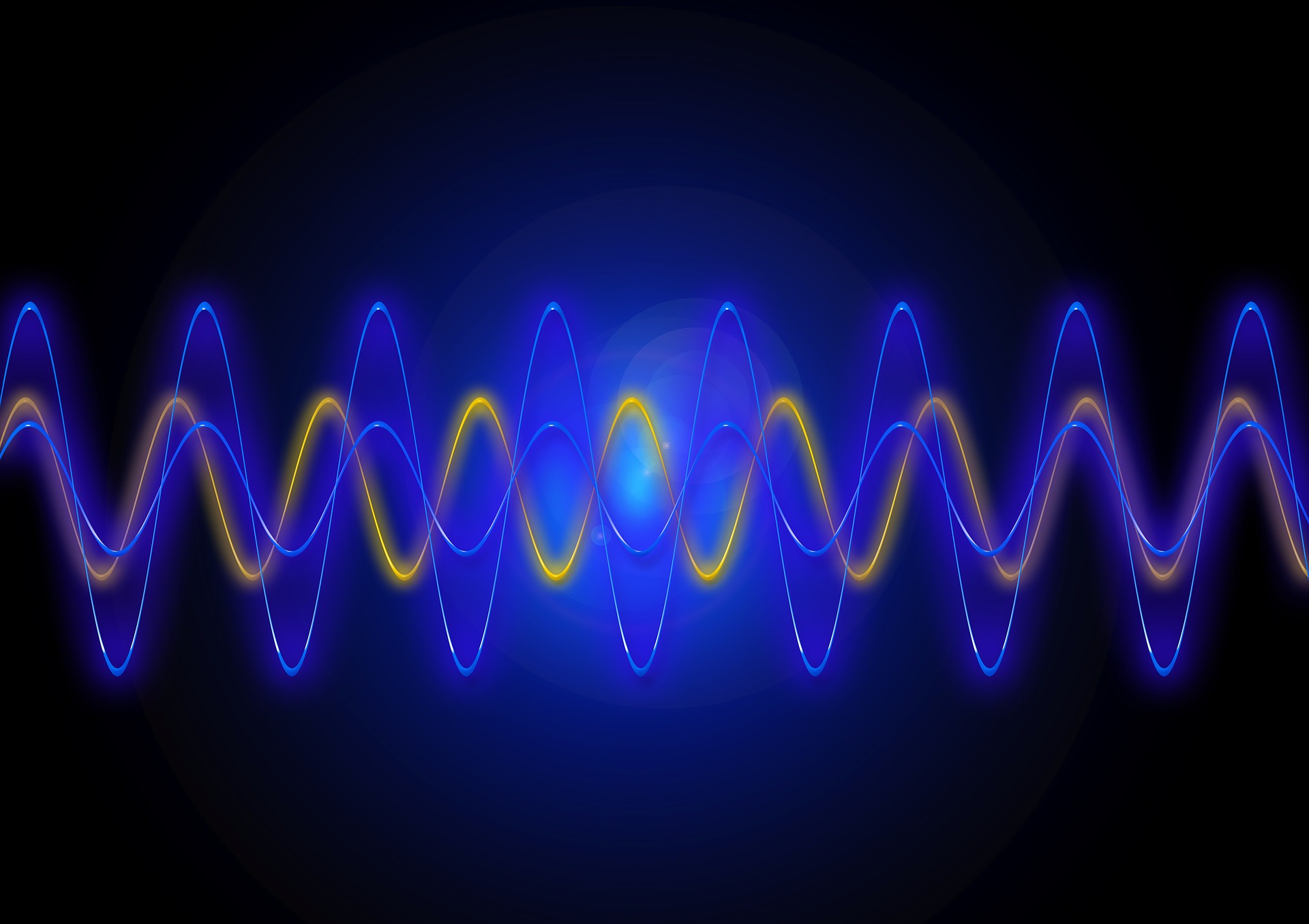
- Read more about Supplementary Material for Rebuttal
- 1 comment
- Log in to post comments
Supplementary material for rebuttal
- Categories:
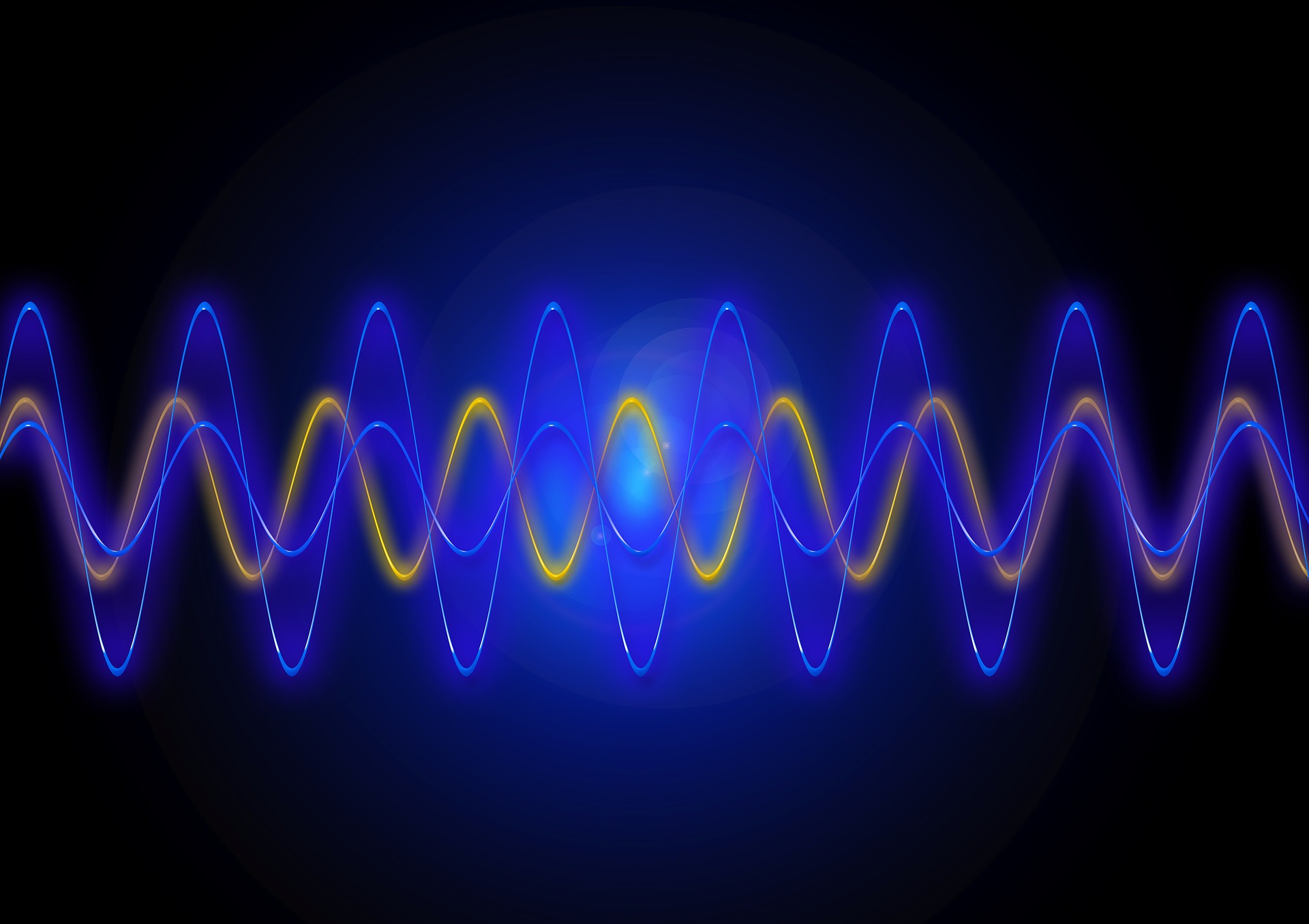
- Read more about ICIP2025_supp
- Log in to post comments
Supplementary materials
supp_main.pdf

- Categories:
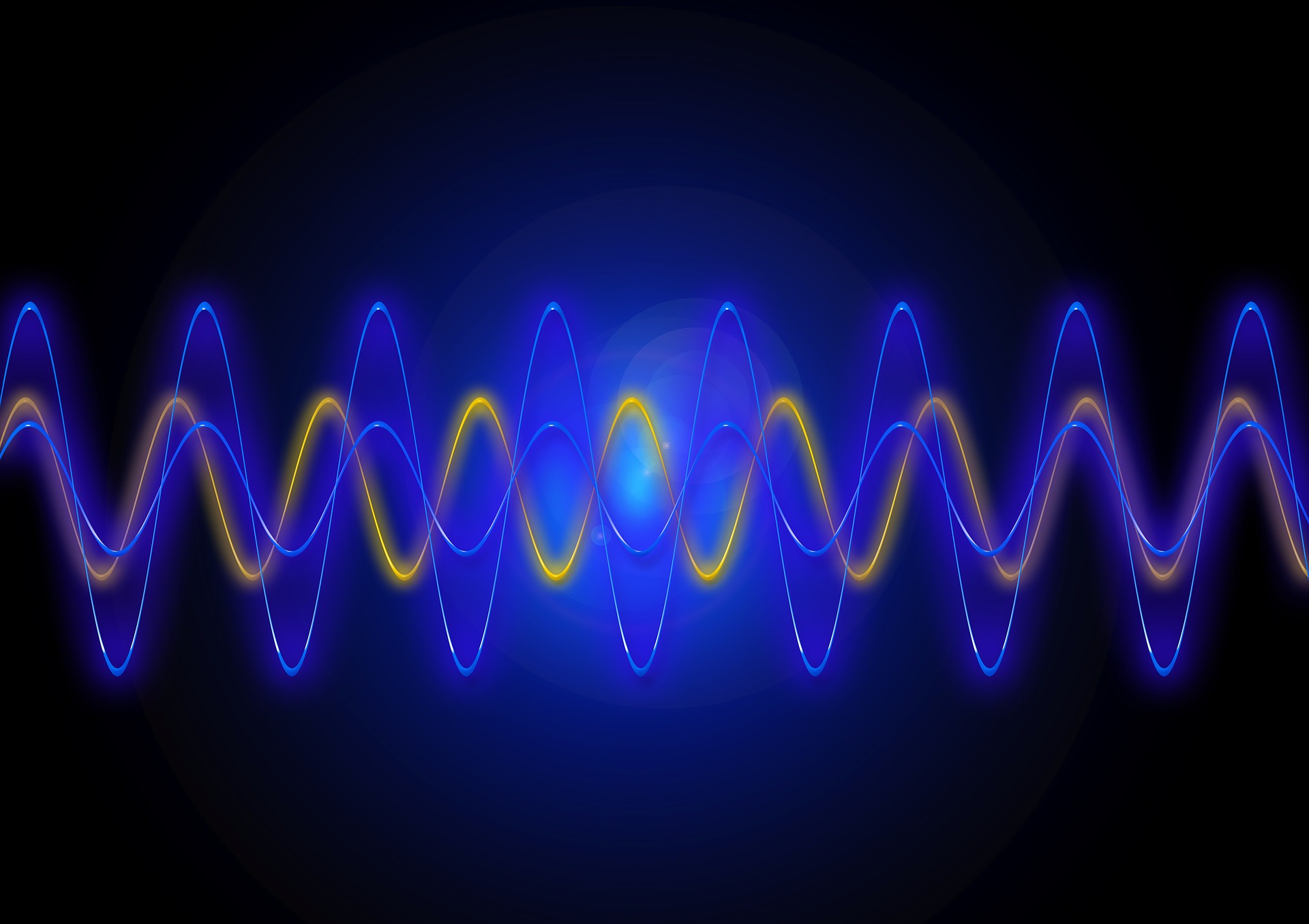
- Read more about ICIP2025_supp
- Log in to post comments
We address the challenge of local feature matching under large scale and rotation changes by focusing on keypoint positions.
First, we propose a novel module called similarity normalization (SN).
This module normalizes keypoint positions to remove a translation, rotation and scale difference between an image pair.
By performing positional encoding on these normalized positions, a network incorporated with SN can effectively avoid encoding largly different positions into descriptors from the two images.
supp_main.pdf

- Categories:
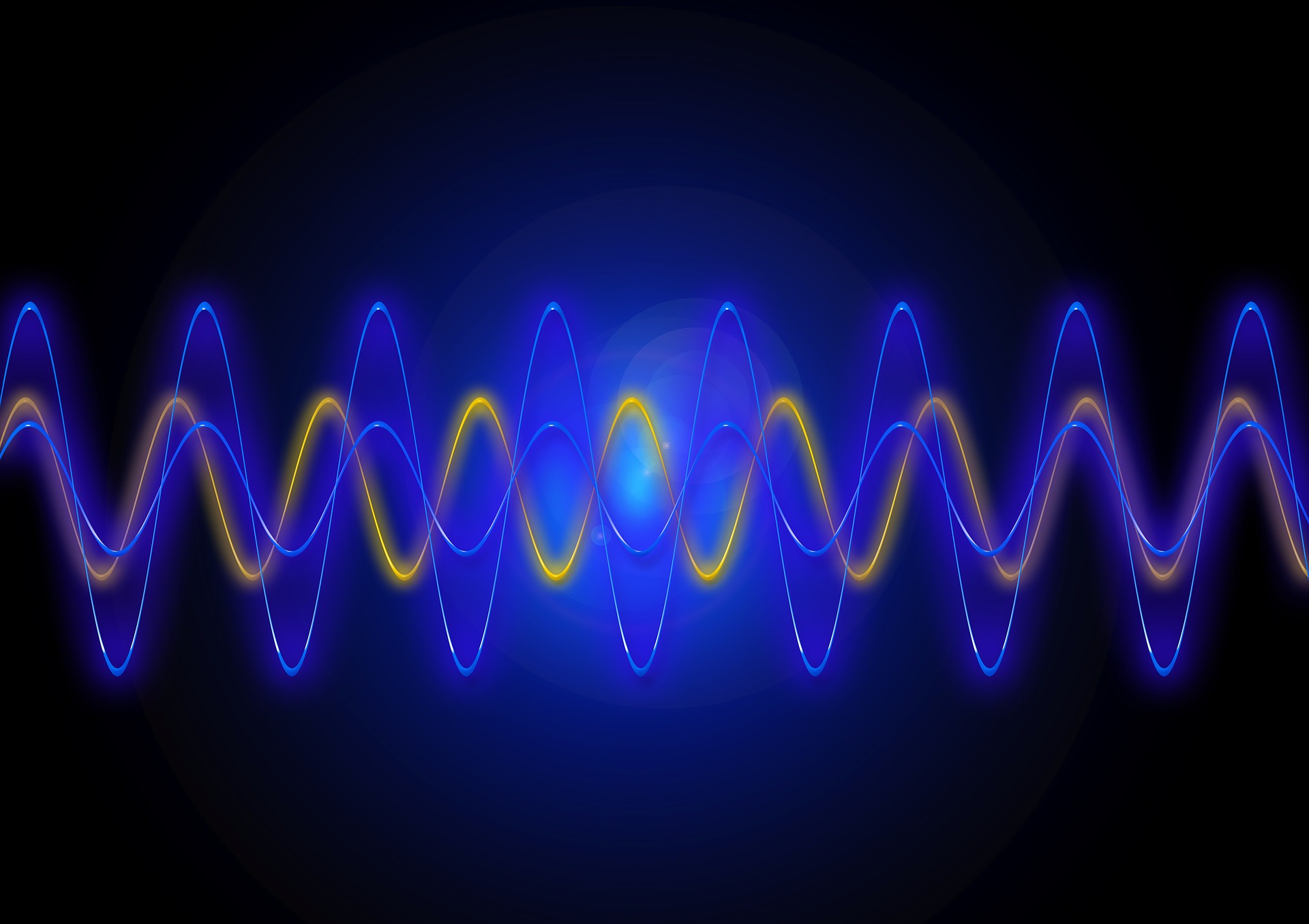
- Read more about RetCLIP Case Studies
- 1 comment
- Log in to post comments
In this work, we propose a retrieval-based method for improving open vocabulary panoptic segmentation.
- Categories:
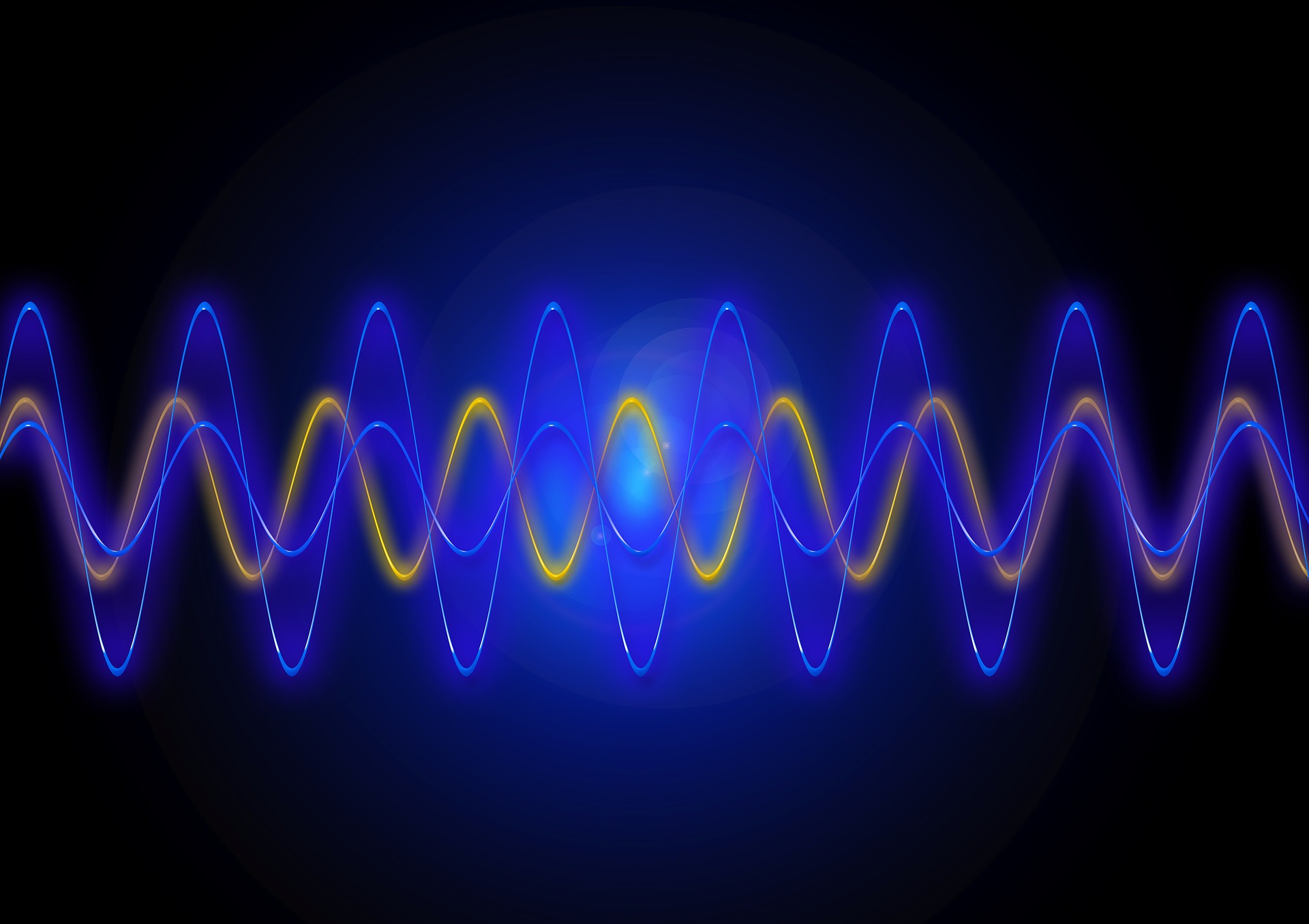
- Read more about Supplementary Material
- 1 comment
- Log in to post comments
Although many deepfake detection methods have been proposed to fight against severe misuse of generative AI, none provide detailed human-interpretable explanations beyond simple real/fake responses. This limitation makes it challenging for humans to assess the accuracy of detection results, especially when the models encounter unseen deepfakes. To address this issue, we propose a novel deepfake detector based on a large Vision-Language Model (VLM), capable of explaining manipulated facial regions.
- Categories:
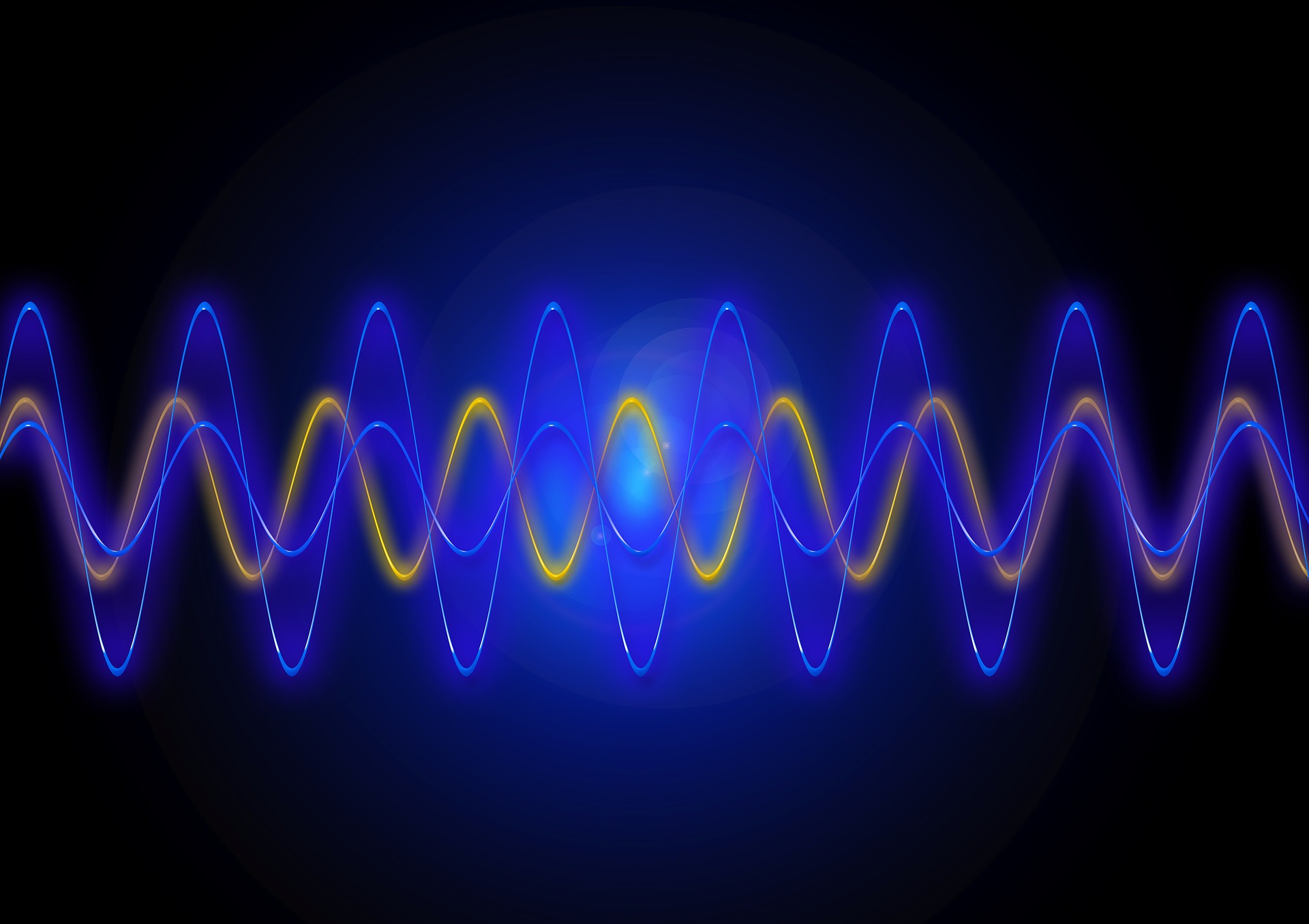
- Read more about Intriguing Equivalence Structures of the Embedding Space of Vision Transformers
- Log in to post comments
Pre-trained large foundation models play a central role in the recent surge of artificial intelligence, resulting in fine-tuned models with remarkable abilities when measured on benchmark datasets, standard exams, and applications. Due to their inherent complexity, these models are not well understood; in particular, the structures of the representation space are not well characterized despite their fundamental importance. In this paper,
- Categories:
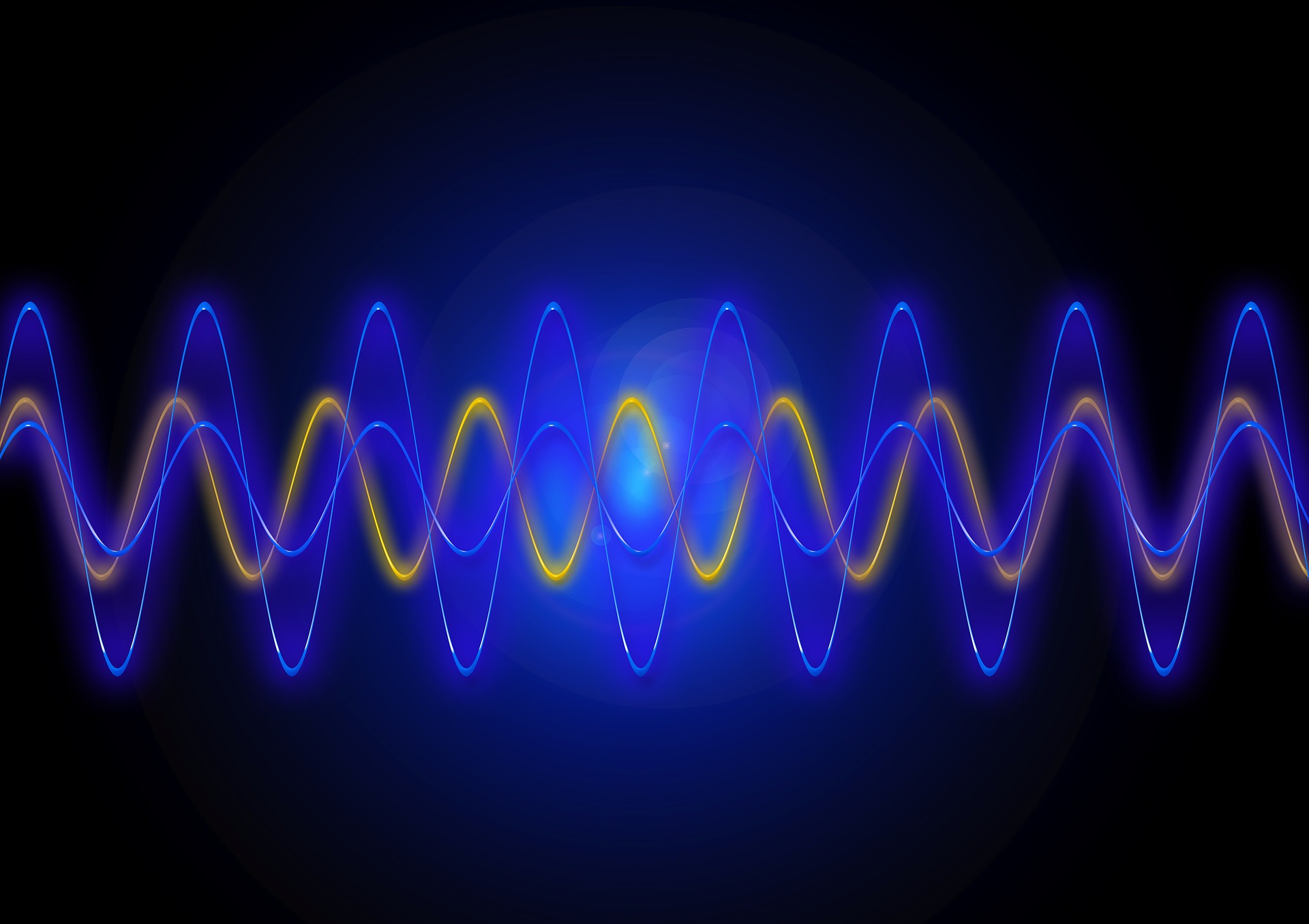
- Read more about supplementary material
- Log in to post comments
supplementary material
- Categories:
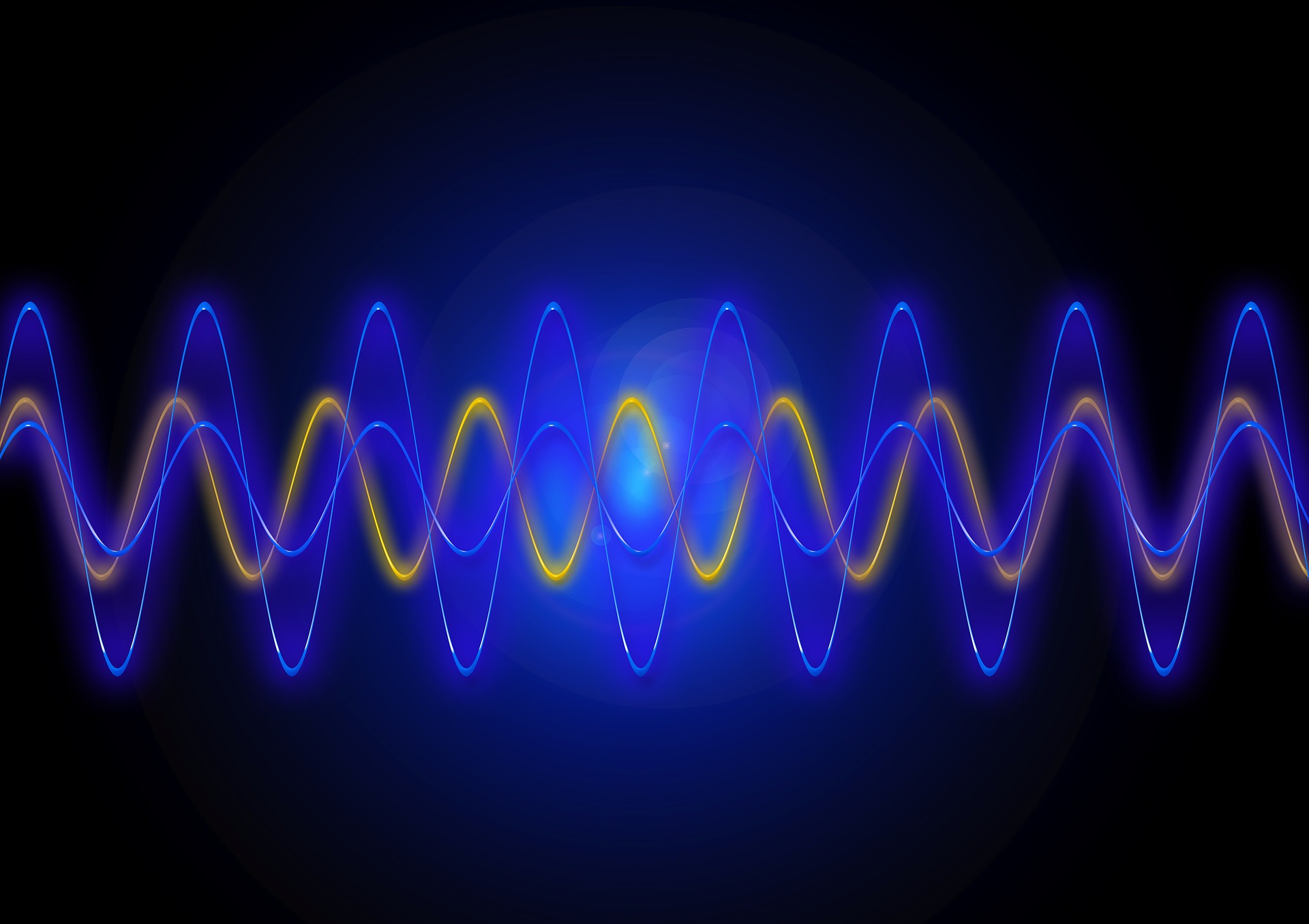
- Read more about ROLLOUT-GUIDED TOKEN PRUNING FOR EFFICIENT VIDEO UNDERSTANDING
- Log in to post comments
Supp for our ICIP submission: ROLLOUT-GUIDED TOKEN PRUNING FOR EFFICIENT VIDEO UNDERSTANDING
RGTP_supp.pdf

- Categories: