Documents
Poster
DEPTH SUPER-RESOLUTION USING JOINT ADAPTIVE WEIGHTED LEAST SQUARES AND PATCHING GRADIENT
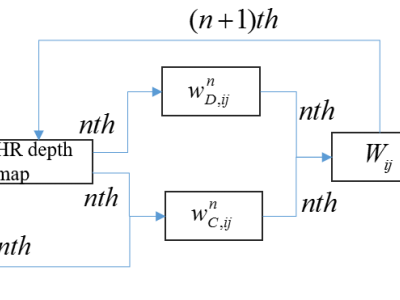
- Citation Author(s):
- Submitted by:
- Yuyuan Li
- Last updated:
- 20 April 2018 - 1:54am
- Document Type:
- Poster
- Document Year:
- 2018
- Event:
- Presenters:
- Yuyuan Li
- Paper Code:
- IVMSP-P2.4
- Categories:
- Keywords:
- Log in to post comments
This paper presents a flexible framework for the challenging task of color-guided depth upsampling. Some state-of-the-art approaches apply an aligned RGB image for depth recovery. Unfortunately, these kinds of methods may result in texture copying artifacts and edge blurring artifacts. To address these difficulties, we propose an adaptive weighted least squares framework of choosing different guidance weight for variant conditions flexibly. First of all, in the framework, we propose a joint adaptive color weighting scheme in which the depth maps and color images jointly choose a proper weight term for diverse cases. Then, a patch-based smoothness measuring approach called patching-gradient method (PGM) is proposed to distinguish the discontinuities and smooth areas. Our PGM is robust to dense noise and preserve weak edges effectively. Quantitative and qualitative experiments on noisy ToF-like datasets demonstrate our frameworks effectiveness on suppressing both texture copying artifacts and edge blurring artifacts.