Documents
Poster
Dictionary learning algorithm for Multi-Subject fMRI analysis via temporal and spatial concatenation
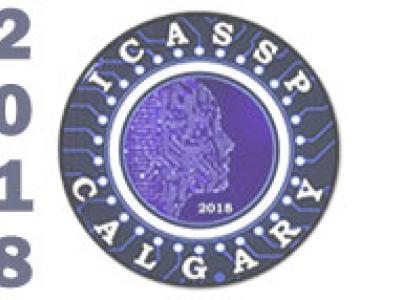
- Citation Author(s):
- Submitted by:
- Asif Iqbal
- Last updated:
- 14 April 2018 - 9:20pm
- Document Type:
- Poster
- Document Year:
- 2018
- Event:
- Presenters:
- Abd-Krim Seghouane
- Paper Code:
- 1964
- Categories:
- Keywords:
- Log in to post comments
In recent history, dictionary learning (DL) methods have been successfully used for analyzing multi-subject functional magnetic resonance imaging. These algorithms try to learn group-level spatial activation maps (SM) or voxel time courses (TC) from temporally or spatially concatenated fMRI datasets respectively. However, in multi-subject fMRI studies, we are interested in both group-level TCs as well as SMs. In this paper, we propose a DL algorithm which combines temporally and spatially concatenated fMRI datasets to learn not only the shared TC/SM pairs but also the subject-specific ones. We do this by separating group-level information and sub-specific information from each subject fMRI dataset. Performance of the proposed algorithm is illustrated using simulated as well as experimental task fMRI datasets.