Documents
Poster
Dictionary Learning-based Image Compression
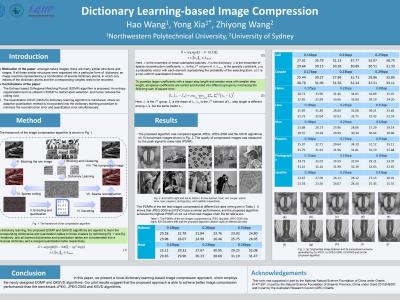
- Citation Author(s):
- Submitted by:
- Hao Wang
- Last updated:
- 17 September 2017 - 3:28pm
- Document Type:
- Poster
- Document Year:
- 2017
- Event:
- Paper Code:
- TP-PC.3
- Categories:
- Log in to post comments
Dictionary learning based image compression has attracted a lot of research efforts due to the inherent sparsity of image contents. Most algorithms in the literature, however, suffer from two drawbacks. First, the atoms selected for image patch reconstruction scatter over the entire dictionary, which leads to a high coding cost. Second, the sparse representation of image patches is performed independently from the quantization of sparse coefficients, which may result in a sub-optimal solution. In this paper, we propose the entropy based orthogonal matching pursuit (EOMP) algorithm and quantization KSVD (QKSVD) algorithm for dictionary learning-based image compression. An entropy regularization term is utilized in EOMP to restrict atom selection, and hence reduces the coding cost, and an adaptive quantization method is incorporated into the dictionary learning procedure in QKSVD to minimize the reconstruction error and quantization error simultaneously. Experimental results on 10 standard benchmark images demonstrate that our proposed approach achieves better performance than several state-of-the-art ones at low bit
rate, such as KSVD based compression approach, JPEG, and JPEG-2000.