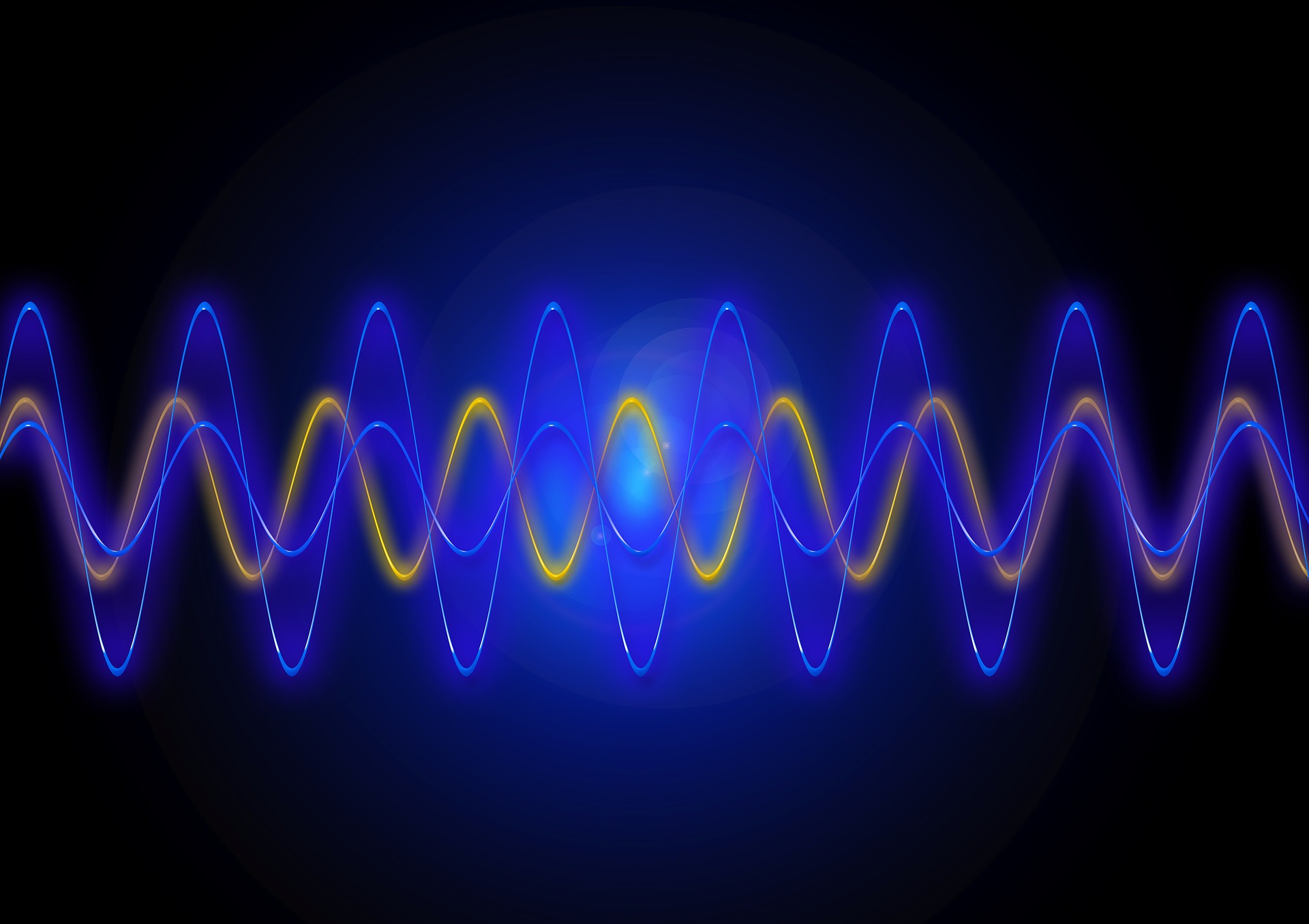
- Read more about APPENDIX
- Log in to post comments
This is supplementary material.
- Categories:
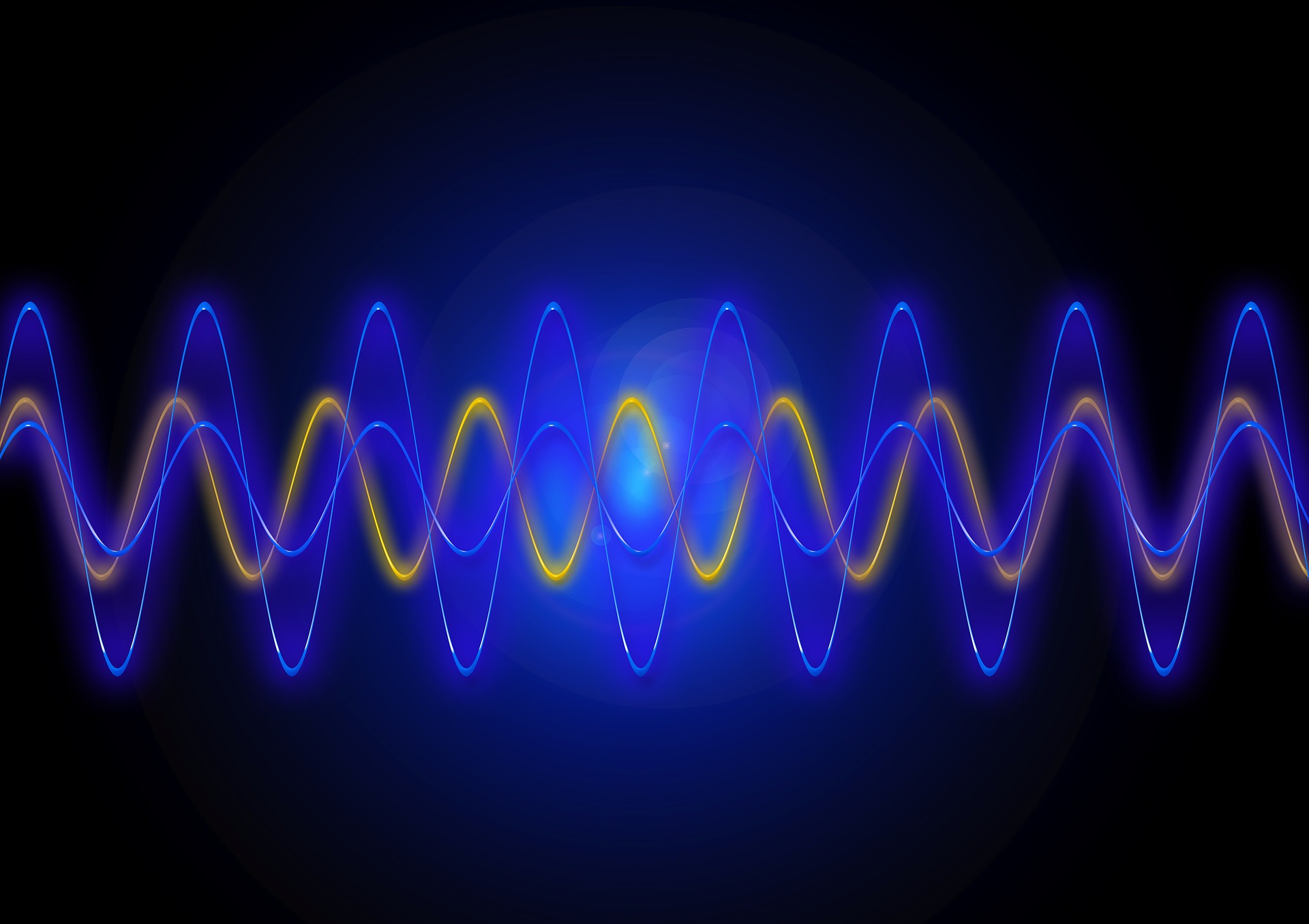
- Read more about SUPPLEMENTARY: DIFFUSION-BASED COMPRESSION QUALITY TRADEOFFS WITHOUT RETRAINING
- Log in to post comments
Learned image compression methods using a generative decoder can reconstruct images at significantly higher perceptual quality than new hand-crafted codecs or other learned methods. Recently, diffusion models have been integrated into the decoding process to further enhance image quality.
However, the diffusion process is sensitive to several hyper-parameters, such as the number of steps, which are typically hard-coded and expected to perform well across various images. When applied to a single image, these parameters are often suboptimal.
- Categories:
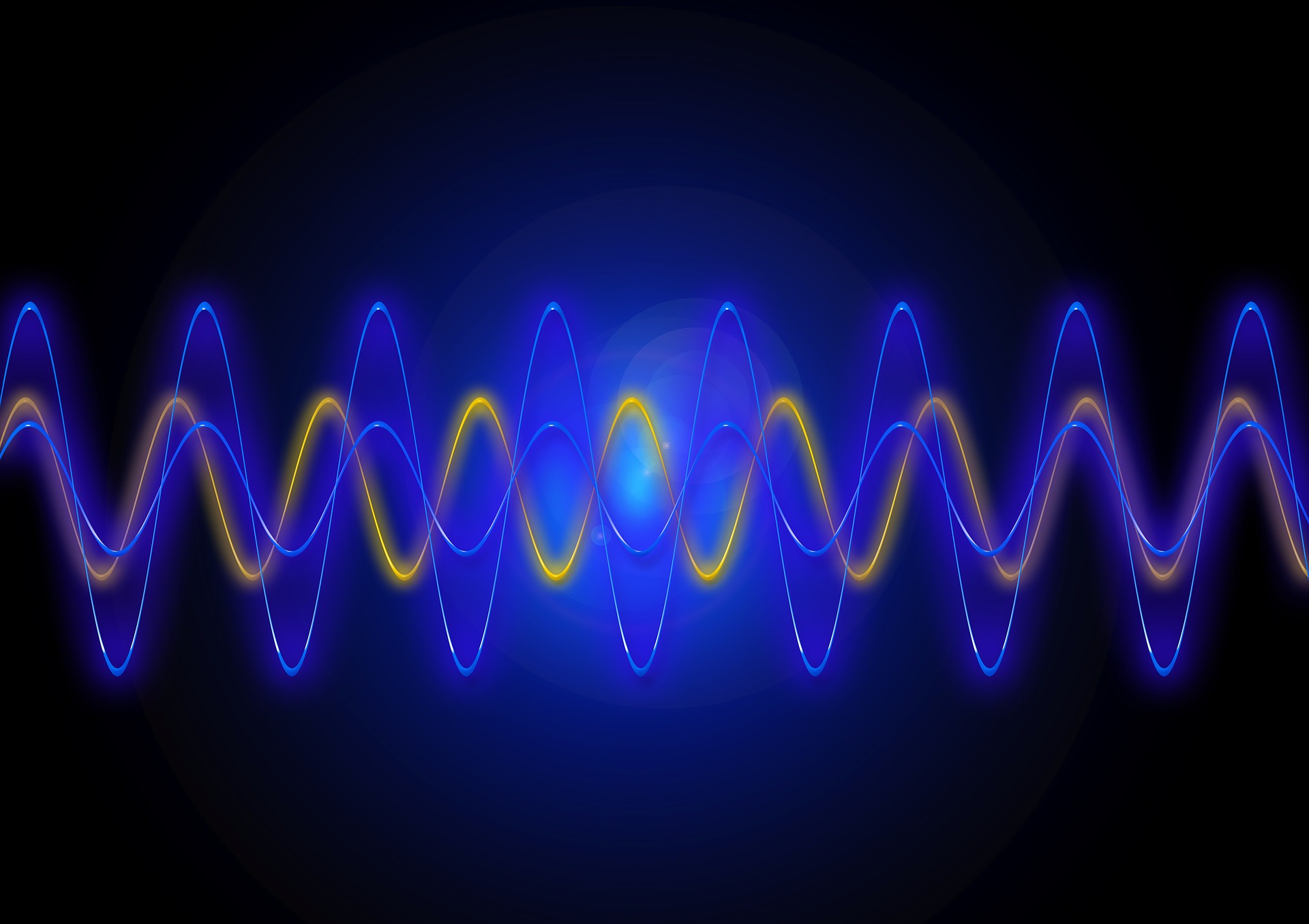
- Read more about JPEG AI Image Compression Artifacts
- Log in to post comments
This supplementary material accompanies the paper "JPEG AI Image Compression Artifacts: Detection Methods and Dataset", submitted to ICIP 2025. It presents a detailed evaluation of hyperparameter selection for the proposed artifact detection methods, demonstrating their impact on performance.
- Categories:
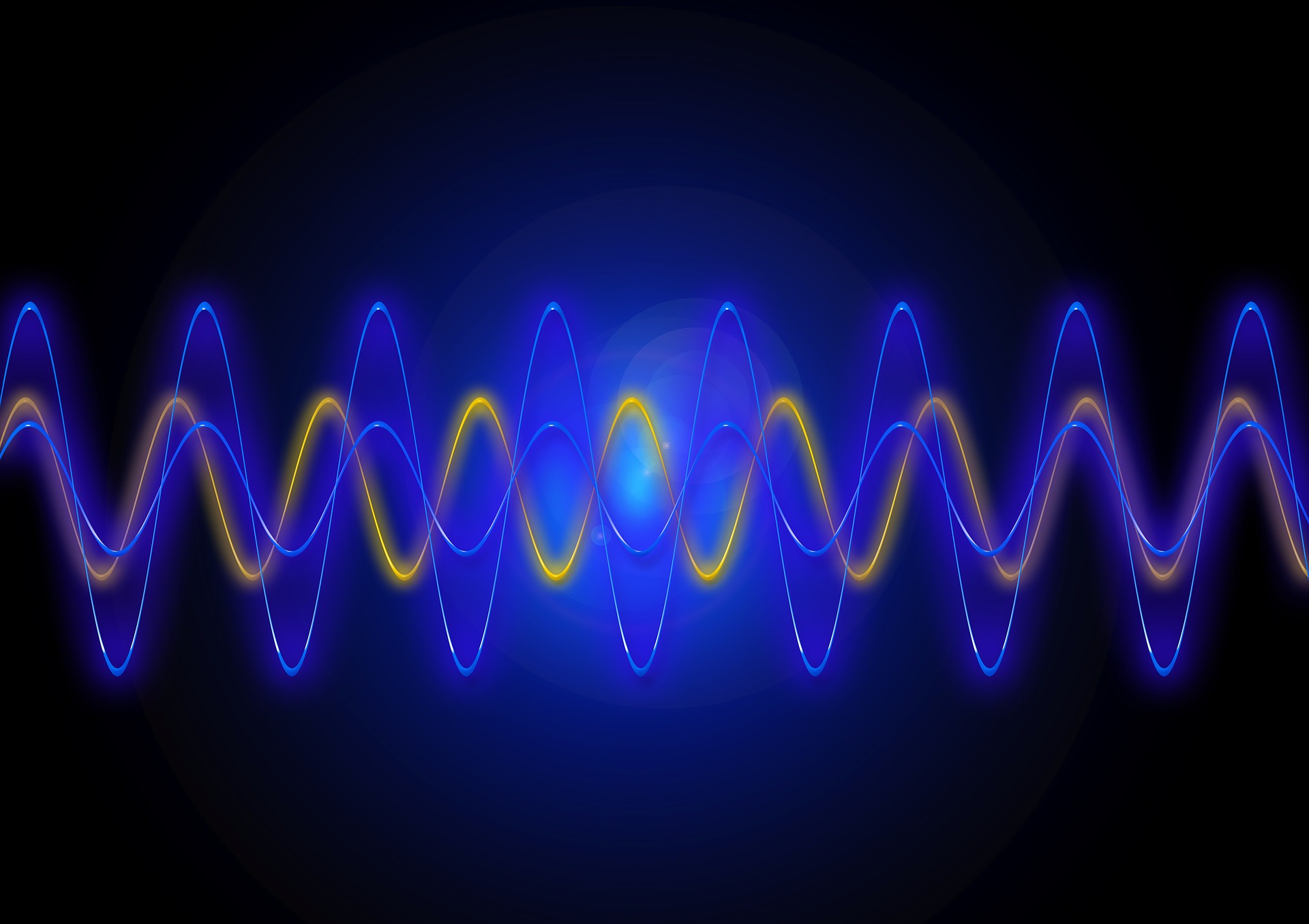
- Read more about Generalized Nested Latent Variable Models for Lossy Coding applied to Wind Turbine Scenarios
- Log in to post comments
Rate-distortion optimization through neural networks has accomplished competitive results in compression efficiency and image quality. This learning-based approach seeks to minimize the compromise between compression rate and reconstructed image quality by automatically extracting and retaining crucial information, while discarding less critical details. A successful technique consists in introducing a deep hyperprior that operates within a 2-level nested latent variable model, enhancing compression by capturing complex data dependencies.
- Categories:
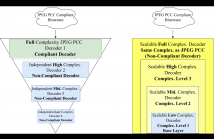
- Read more about Learning-based Point Cloud Decoding With Independent and Scalable Reduced Complexity
- 1 comment
- Log in to post comments
Point Clouds (PCs) have gained significant attention due to their usage in diverse application domains, notably virtual and augmented reality. While PCs excel in providing detailed 3D visualization, this typically requires millions of points which must be efficiently coded for real-world deployment, notably storage and streaming. Recently, learning-based coding solutions have been adopted, notably in the JPEG Pleno Point Coding (PCC) standard, which uses a coding model with millions of model parameters.
- Categories:
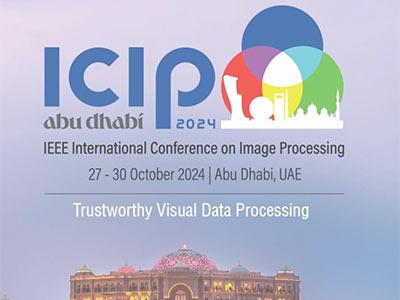
- Read more about Redefining Visual Quality: The Impact of Loss Functions on INR-Based Image Compression
- Log in to post comments
Implicit Neural Representations (INR) are a novel data representation technique which is gaining ground in the image compression field due to its simplicity and interesting results in terms of rate/distortion ratio.
- Categories:
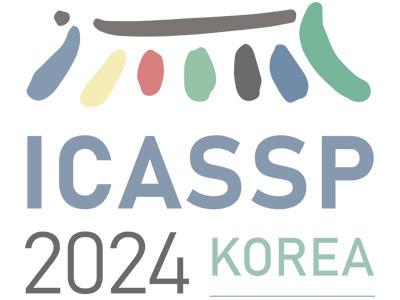
- Read more about Poster for the paper "Buffered Gaussian Modeling For Vectorized HD Map Construction"
- Log in to post comments
Vectorized high-definition (HD) map construction is an important and challenging task for autonomous driving. End-to-end models have been developed recently to enable online map construction. Existing works have difficulty in generating complex geometric shapes and lack comprehensive evaluation metrics. To tackle these challenges, we introduce buffered IoU as a novel metric for vectorized map construction, which is clearly defined and applicable to real-world situations. Inspired by methods of rotated object detection, we further propose a novel technique called Buffered Gaussian Modeling.
- Categories:

- Read more about MULTI-LEVEL CONTRASTIVE LEARNING FOR HYBRID CROSS-MODAL RETRIEVAL
- Log in to post comments
Hybrid image retrieval is a significant task for a wide range of applications. In this scenario, the hybrid query for searching images consists of a reference image and a text modifier. The reference image provides a vital visual context and displays some semantic details, while the text modifier specifies the modifications to the reference image. To address such hybrid cross-modal retrieval, we propose a multi-level contrastive learning (MLCL) method for combining the hybrid query features into a fused feature by cross-modal contrastive learning with multi-level semantic alignment.
- Categories:
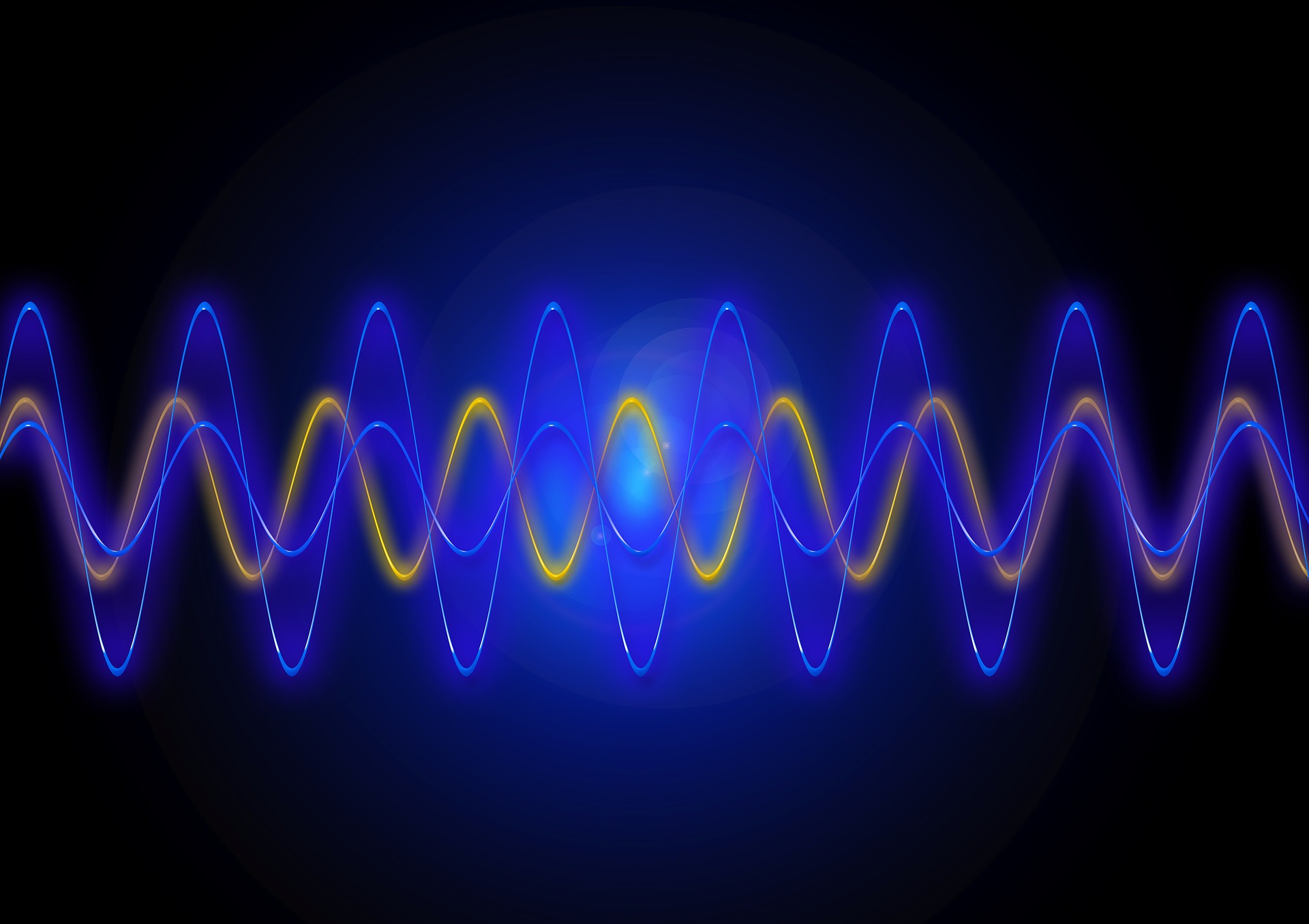
Versatile Video Coding (VVC) now supports Screen Content Coding (SCC) by integrating two efficient coding modes: Intra Block Copy (IBC) and Palette (PLT). However, the numerous
modes and the Quad-Tree Plus Multi-Type Tree (QTMT) structure inherent to VVC contribute to a very high coding complexity. To effectively reduce the computational complexity
of VVC SCC, we propose a fast Intra mode prediction algorithm for VVC SCC. More specifically, we first use the difference of minimum Sum of Absolute Transformed Differences
- Categories:
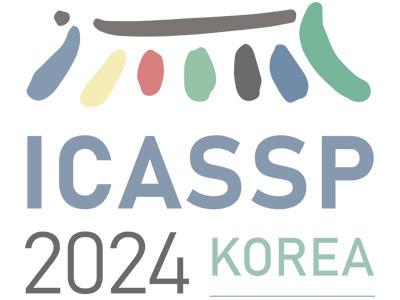
- Read more about Learned Video Compression with Spatial-Temporal Optimization
- Log in to post comments
Previous optical flow based video compression is gradually replaced by unsupervised deformable convolution (DCN) based method. This is mainly due to the fact that the motion vector (MV) estimated by the existing optical flow network
- Categories: