Documents
Poster
DISCOVERING MALICIOUS SIGNATURES IN SOFTWARE FROM STRUCTURAL INTERACTIONS
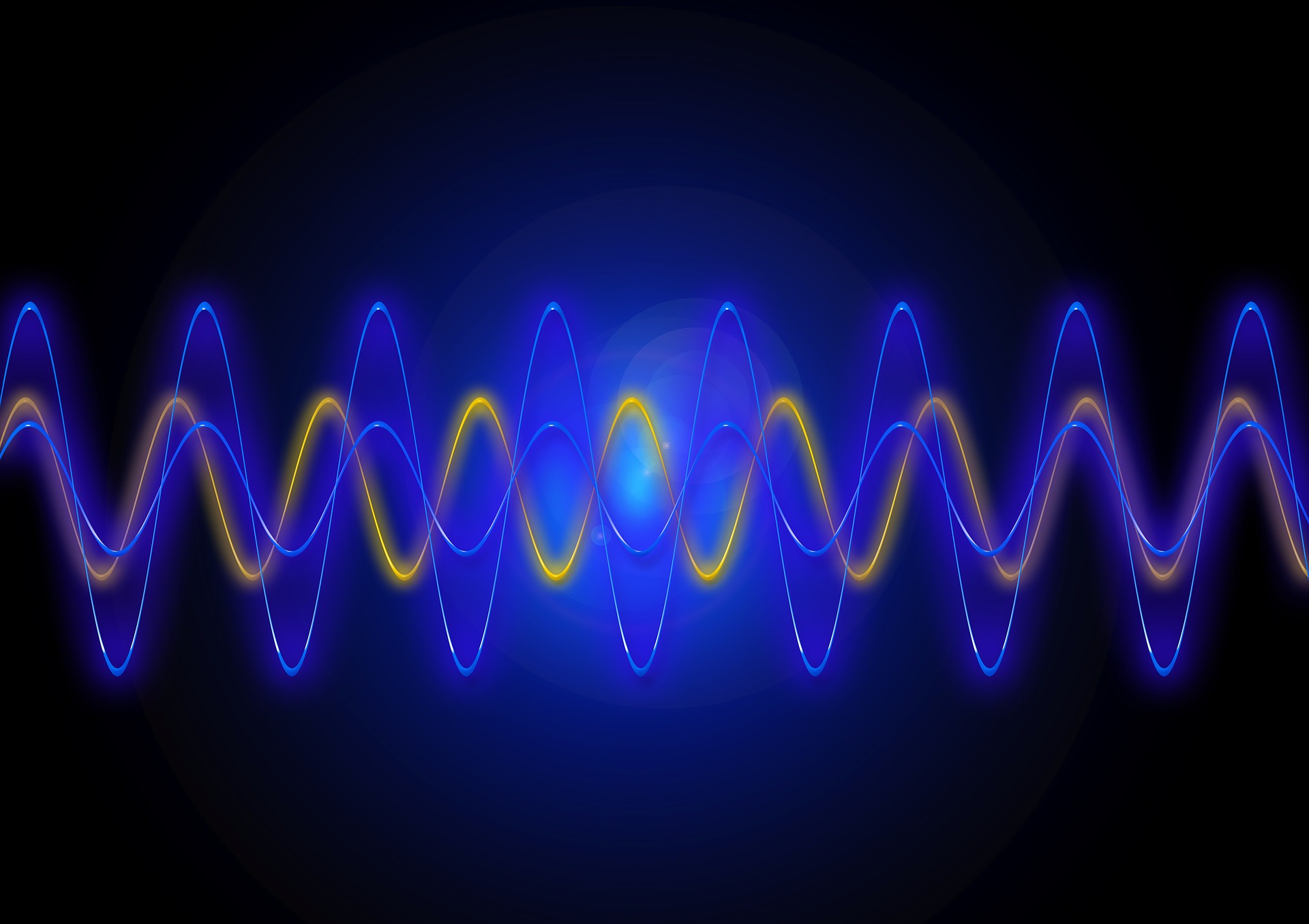
- DOI:
- 10.60864/k6td-3m24
- Citation Author(s):
- Submitted by:
- Chenzhong Yin
- Last updated:
- 6 June 2024 - 10:27am
- Document Type:
- Poster
- Paper Code:
- IFS-P4.10
- Categories:
- Log in to post comments
Malware represents a significant security concern in today's digital landscape, as it can destroy or disable operating systems, steal sensitive user information, and occupy valuable disk space.
However, current malware detection methods, such as static-based and dynamic-based approaches, struggle to identify newly developed (``zero-day") malware and are limited by customized virtual machine (VM) environments.
To overcome these limitations, we propose a novel malware detection approach that leverages deep learning, mathematical techniques, and network science.
Our approach focuses on static and dynamic analysis and utilizes the Low-Level Virtual Machine (LLVM) to profile applications within a complex network. The generated network topologies are input into the GraphSAGE architecture to efficiently distinguish between benign and malicious software applications, with the operation names denoted as node features.
Importantly, the GraphSAGE models analyze the network's topological geometry to make predictions, enabling them to detect state-of-the-art malware and prevent potential damage during execution in a VM.
To evaluate our approach, we conduct a study on a dataset comprising source code from 24,376 applications, specifically written in C/C++, sourced directly from widely-recognized malware and various types of benign software. The results show a high detection performance with an Area Under the Receiver Operating Characteristic Curve (AUROC) of 99.85\%. Our approach marks a substantial improvement in malware detection, providing a notably more accurate and efficient solution when compared to current state-of-the-art malware detection methods. The code is released at https://github.com/HantangZhang/MGN.