Documents
Poster
EARLY DIAGNOSING PARKINSON'S DISEASE VIA A DEEP LEARNING MODEL BASED ON AUGMENTED FACIAL EXPRESSION DATA
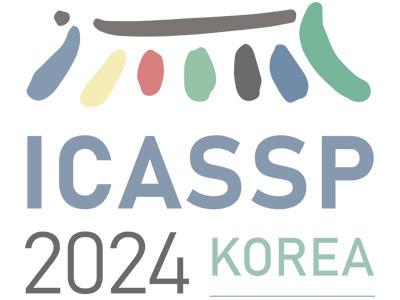
- DOI:
- 10.60864/hsjb-t033
- Citation Author(s):
- Submitted by:
- Yintao Zhou
- Last updated:
- 6 June 2024 - 10:54am
- Document Type:
- Poster
- Document Year:
- 2024
- Event:
- Categories:
- Log in to post comments
It is crucial to promptly diagnose potential Parkinson's disease (PD) patients in order to facilitate early treatment and prevent disease progression. In recent years, there has been growing interest in using facial expressions for in-vitro PD diagnosis due to the distinct "masked face" characteristics of PD patients and the cost-effectiveness of this approach. However, current facial expression-based PD diagnosis methods are hindered by limited training data on PD patients' facial expressions and weak prediction models. To address these issues, we propose a new PD diagnosis method that utilizes facial expression data augmentation and deep neural network prediction. Our approach involves two stages: 1) generating virtual facial expression images depicting six basic emotions (anger, disgust, fear, happiness, sadness, and surprise) through multi-domain adversarial learning to expand the original training data; 2) training a deep neural network prediction model using a combination of the augmented training data from PD patients and facial expression images of normal individuals from public datasets. Qualitative and quantitative experiments confirm the efficacy of our multi-domain adversarial learning-based facial expression synthesis and demonstrate the promising performance of our proposed approach for PD diagnosis.