Documents
Supplementary material
ECAP-Supplementary
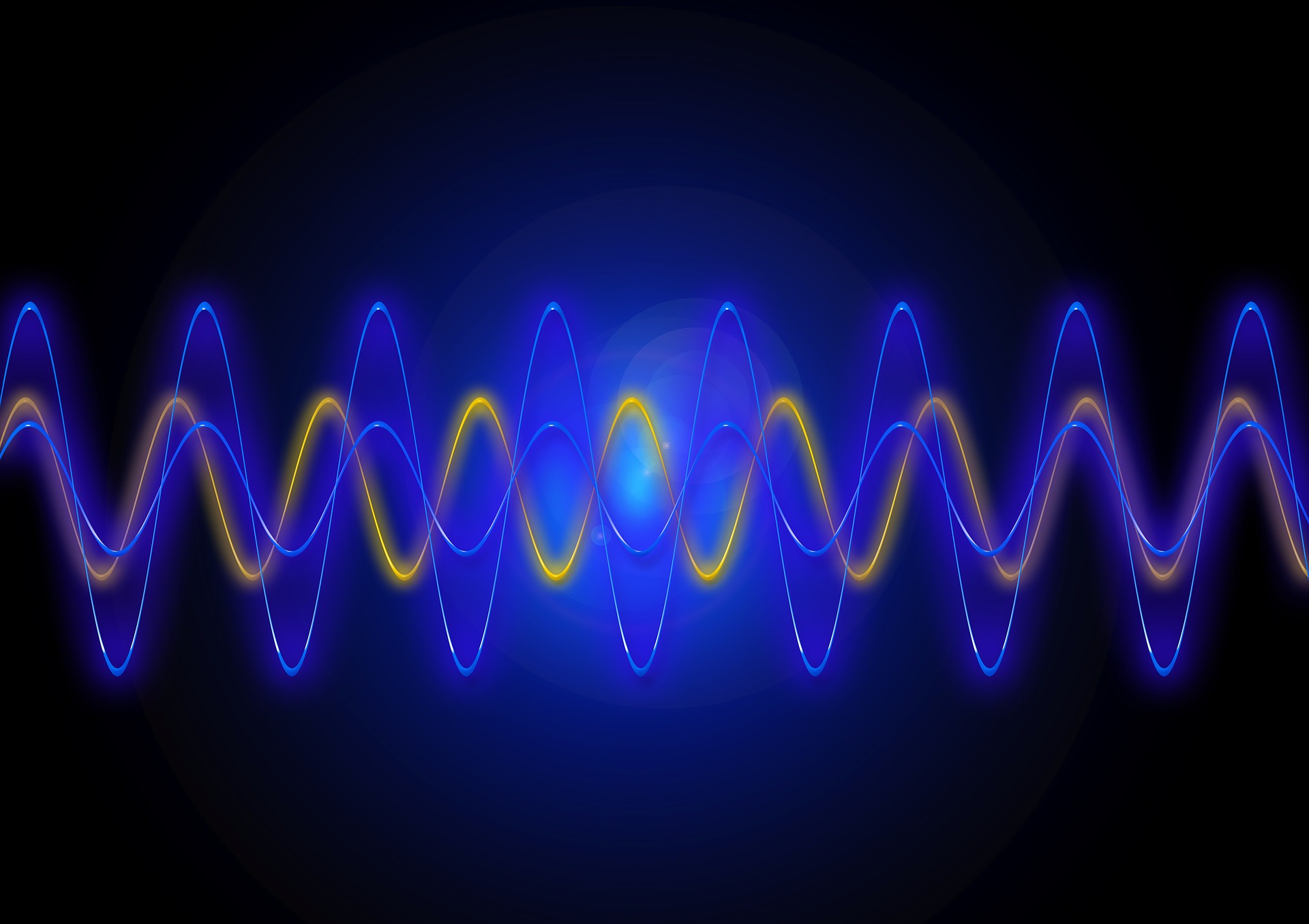
- DOI:
- 10.60864/pzzj-cx04
- Citation Author(s):
- Submitted by:
- Erik Brorsson
- Last updated:
- 2 February 2024 - 2:46am
- Document Type:
- Supplementary material
- Categories:
- Log in to post comments
We consider unsupervised domain adaptation (UDA) for semantic segmentation in which the model is trained on a labeled source dataset and adapted to an unlabeled target dataset. Unfortunately, current self-training methods are susceptible to misclassified pseudo-labels resulting from erroneous predictions. Since certain classes are typically associated with less reliable predictions in UDA, reducing the impact of such pseudo-labels without skewing the training towards some classes is notoriously difficult.
To this end, we propose an extensive cut-and-paste strategy (ECAP) to leverage reliable pseudo-labels through data augmentation.
Specifically, ECAP maintains a memory bank of pseudo-labeled target samples throughout training and cut-and-pastes the most confident ones onto the current training batch. We implement ECAP on top of the recent method MIC and boost its performance on two synthetic-to-real domain adaptation benchmarks. Notably, MIC+ECAP reaches an unprecedented performance of 69.1 mIoU on the Synthia→Cityscapes benchmark. Our code is available at https://github.com/ErikBrorsson/ECAP.