Documents
Poster
ECM-OPCC: Efficient Context Model for Octree-based Point Cloud Compression
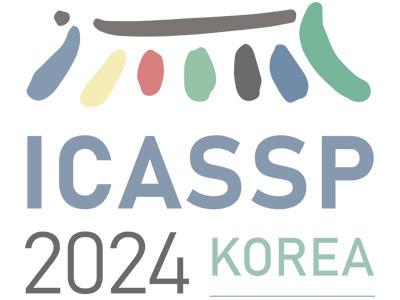
- DOI:
- 10.60864/mrxb-r952
- Citation Author(s):
- Submitted by:
- Yan Wang
- Last updated:
- 6 June 2024 - 10:55am
- Document Type:
- Poster
- Event:
- Presenters:
- Yan Wang
- Paper Code:
- MMSP-P1.6
- Categories:
- Log in to post comments
Recently, deep learning methods have shown promising results in point cloud compression. However, previous octree-based approaches either lack sufficient context or have high decoding complexity (e.g. > 900s). To address this problem, we propose a sufficient yet efficient context model and design an efficient deep learning codec for point clouds. Specifically, we first propose a segment-constrained multi-group coding strategy to exploit the autoregressive context while maintaining decoding efficiency. Then, we propose a dual transformer architecture to utilize the dependency of current node on its ancestors and siblings. We also propose a random-masking pre-train method to enhance our model. Experimental results show that our approach achieves state-of-the-art performance for both lossy and lossless point cloud compression, and saves a significant amount of decoding time compared with previous octree-based SOTA compression methods.