Documents
Presentation Slides
EFFICIENT CLOUD DETECTION IN REMOTE SENSING IMAGES USING EDGE-AWARE SEGMENTATION NETWORK AND EASY-TO-HARD TRAINING STRATEGY
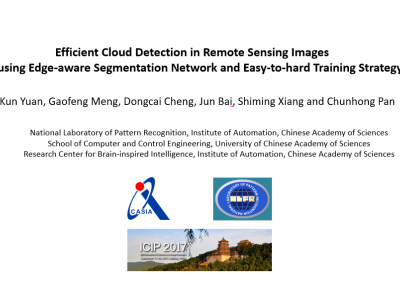
- Citation Author(s):
- Submitted by:
- Kun Yuan
- Last updated:
- 15 September 2017 - 5:07am
- Document Type:
- Presentation Slides
- Document Year:
- 2017
- Event:
- Presenters:
- Kun Yuan
- Paper Code:
- 1278
- Categories:
- Log in to post comments
Detecting cloud regions in remote sensing image (RSI) is very challenging yet of great importance to meteorological forecasting and other RSI-related applications. Technically, this task is typically implemented as a pixel-level segmentation. However, traditional methods based on handcrafted or low-level cloud features often fail to achieve satisfactory performances from images with bright non-cloud and/or semitransparent cloud regions. What is more, the performances could be further degraded due to the ambiguous boundaries caused by complicated textures and non-uniform distribution of intensities. In this paper, we propose a multi-task based deep neural network for cloud detection in RSIs. Architecturally, our network is designed to combine the two tasks of cloud segmentation and cloud edge detection together to encourage a better detection near cloud boundaries, resulting in an end-to-end approach for accurate cloud detection. Accordingly, an efficient sample selection strategy is proposed to train our network in an easy-to-hard manner, in which the number of the selected samples is governed by a weight that is annealed until the entire training samples have been considered. Both visual and quantitative comparisons are conducted on RSIs collected from Google Earth. The experimental results indicate that our method can yield superior performance over the state-of-the-art methods.