Documents
Presentation Slides
Emotion Neural Transducer for Fine-Grained Speech Emotion Recognition
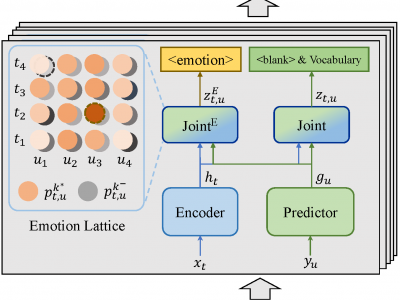
- DOI:
- 10.60864/4766-m532
- Citation Author(s):
- Submitted by:
- Siyuan Shen
- Last updated:
- 6 June 2024 - 10:32am
- Document Type:
- Presentation Slides
- Document Year:
- 2024
- Event:
- Presenters:
- Siyuan Shen
- Paper Code:
- SLP-L4.3
- Categories:
- Log in to post comments
The mainstream paradigm of speech emotion recognition (SER) is identifying the single emotion label of the entire utterance. This line of works neglect the emotion dynamics at fine temporal granularity and mostly fail to leverage linguistic information of speech signal explicitly. In this paper, we propose Emotion Neural Transducer for fine-grained speech emotion recognition with automatic speech recognition (ASR) joint training. We first extend typical neural transducer with emotion joint network to construct emotion lattice for fine-grained SER. Then we propose lattice max pooling on the alignment lattice to facilitate distinguishing emotional and non-emotional frames. To adapt fine-grained SER to transducer inference manner, we further make blank, the special symbol of ASR, serve as underlying emotion indicator as well, yielding Factorized Emotion Neural Transducer. For typical utterance-level SER, our ENT models outperform state-of-the-art methods on IEMOCAP in low word error rate. Experiments on IEMOCAP and the latest speech emotion diarization dataset ZED also demonstrate the superiority of fine-grained emotion modeling. Our code is available at https://github.com/ECNU-Cross-Innovation-Lab/ENT.