Documents
Presentation Slides
End-to-end deep-learning-based image compression for machine tasks, a study
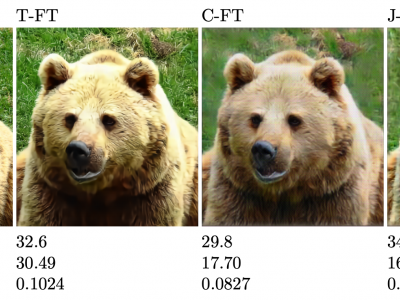
- Citation Author(s):
- Submitted by:
- Fabien Racape
- Last updated:
- 28 February 2021 - 5:53pm
- Document Type:
- Presentation Slides
- Document Year:
- 2021
- Event:
- Presenters:
- Lahiru D. Chamain
- Paper Code:
- 174
- Categories:
- Log in to post comments
This paper provides an in-depth study of a framework consisting of combining trainable im- age and video codecs with machine task algorithms. The field of video coding optimization for machines is gaining traction, due to the increasing share of image and video content that is dedicated to be analyzed by machines, rather than viewed by humans. Recent works in image compression have demonstrated the potential of end-to-end deep-learning-based auto-encoders that can be trained to optimally compress images and videos with respect to a target rate and any given differentiable quality metric. In parallel, deep neural networks, which are also made differentiable for training, have shown state-of-the-art performance for a variety of computer vision tasks. As it remains challenging to specialize a traditional hy- brid encoder, which considers images block by block, for such task, we explore the promises of auto-encoders to address content compression for machines. After a comparison between traditional codecs and the proposed framework, we study and discuss the performances of several configurations in terms of joint training of the codec and the task algorithm in the context of object detection.