Documents
Poster
End-to-End Multimodal Speech Recognition
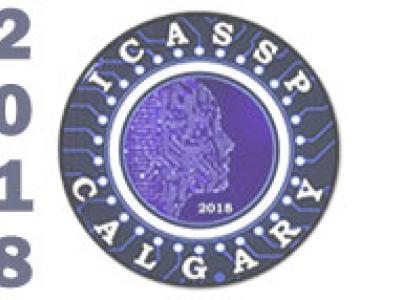
- Citation Author(s):
- Submitted by:
- Shruti Palaskar
- Last updated:
- 12 April 2018 - 8:02pm
- Document Type:
- Poster
- Document Year:
- 2018
- Event:
- Presenters:
- Shruti Palaskar, Ramon Sanabria and Florian Metze
- Paper Code:
- 4069
- Categories:
- Keywords:
- Log in to post comments
Transcription or sub-titling of open-domain videos is still a chal- lenging domain for Automatic Speech Recognition (ASR) due to the data’s challenging acoustics, variable signal processing and the essentially unrestricted domain of the data. In previous work, we have shown that the visual channel – specifically object and scene features – can help to adapt the acoustic model (AM) and language model (LM) of a recognizer, and we are now expanding this work to end-to-end approaches. In the case of a Connectionist Tempo- ral Classification (CTC)-based approach, we retain the separation of AM and LM, while for a sequence-to-sequence (S2S) approach, both information sources are adapted together, in a single model. This paper also analyzes the behavior of CTC and S2S models on noisy video data (How-To corpus), and compares it to results on the clean Wall Street Journal (WSJ) corpus, providing insight into the robustness of both approaches.
Index Terms— Audiovisual Speech Recognition, Connectionist Temporal Classification, Sequence-to-Sequence Model, Adaptation