Documents
Presentation Slides
Presentation Slides
EnerGAN: A Generative Adversarial Network for Energy Disaggregation
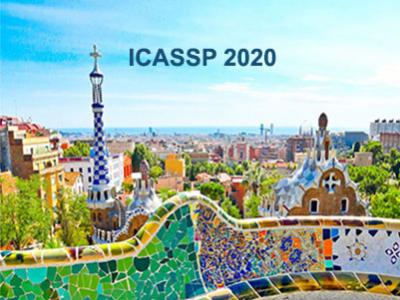
- Citation Author(s):
- Submitted by:
- Maria Kaselimi
- Last updated:
- 27 June 2020 - 2:06pm
- Document Type:
- Presentation Slides
- Document Year:
- 2020
- Event:
- Presenters:
- Maria Kaselimi
- Paper Code:
- DIS-P1.3
- Categories:
- Log in to post comments
An efficient, appliance-level approach for energy disaggregation, exploiting the benefits of Generative Adversarial Networks, is presented. The concept of adversarial training supports the creation of fine tuned disaggregators, which produce more detailed load estimations for a specific appliance, compared to state of the art deep learning models. The Generator and Discriminator of the model are appropriately adapted to fit the particularities of NILM problem, whereas a Seeder component is added to provide encoded compact input vectors to the Generator. The experimental evaluation against state of the art techniques indicates promising results.