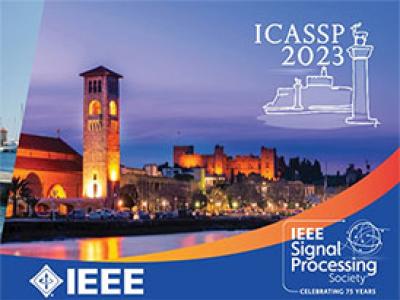
- Read more about Applying Symmetrical Component Transform for Industrial Appliance Classification in Non-Intrusive Load Monitoring
- Log in to post comments
Slides.pdf

- Categories:
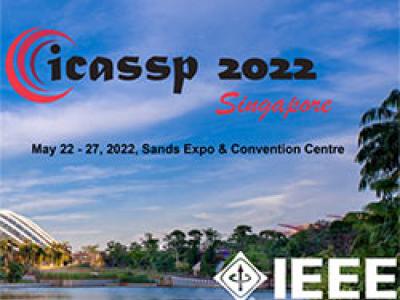
- Read more about Identification of Edge Disconnections in Networks Based on Graph Filter Outputs
- Log in to post comments
Graphs are fundamental mathematical structures used in various fields to model statistical and physical relationships between data, signals, and processes. In some applications, such as data processing in graphs that represent physical networks, the initial network topology is known. However, disconnections of edges in the network change the topology and may affect the signals and processes over the network. In this paper, we consider the problem of edge disconnection identification in networks by using concepts from graph signal processing.
- Categories:
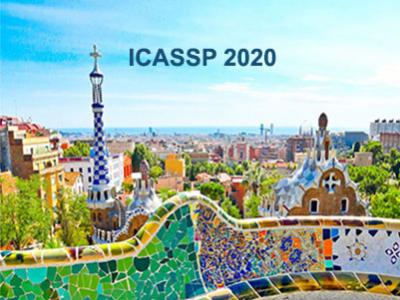
- Read more about Optimal Power Flow Using Graph Neural Networks
- Log in to post comments
Optimal power flow (OPF) is one of the most important optimization problems in the energy industry. In its simplest form, OPF attempts to find the optimal power that the generators within the grid have to produce to satisfy a given demand. Optimality is measured with respect to the cost that each generator incurs in producing this power. The OPF problem is non-convex due to the sinusoidal nature of electrical generation and thus is difficult to solve.
- Categories:
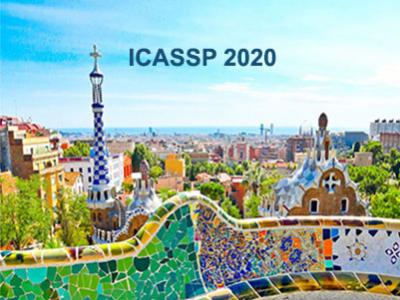
- Read more about EnerGAN: A Generative Adversarial Network for Energy Disaggregation
- Log in to post comments
An efficient, appliance-level approach for energy disaggregation, exploiting the benefits of Generative Adversarial Networks, is presented. The concept of adversarial training supports the creation of fine tuned disaggregators, which produce more detailed load estimations for a specific appliance, compared to state of the art deep learning models. The Generator and Discriminator of the model are appropriately adapted to fit the particularities of NILM problem, whereas a Seeder component is added to provide encoded compact input vectors to the Generator.
- Categories:
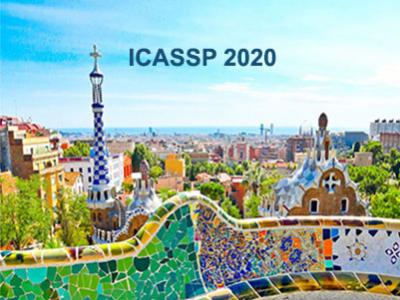
- Read more about STOCHASTIC GEOMETRY PLANNING OF ELECTRIC VEHICLES CHARGING STATIONS
- Log in to post comments
Smart grids are faced with the challenge of meeting the ever increasing load demands of electric vehicles (EVs). To provide acceptable charging services, operators need to be equipped with an efficient charging stations (CSs) planning strategy. Unfortunately, existing planning solutions are quite limited. They normally rely on standard IEEE bus systems or power grids that are specific to certain cities. In this paper, using stochastic geometry, we formulate the CSs planning on a stochastic geometry-based power grid model, that we previously showed to mimic real-world power grids.
- Categories:
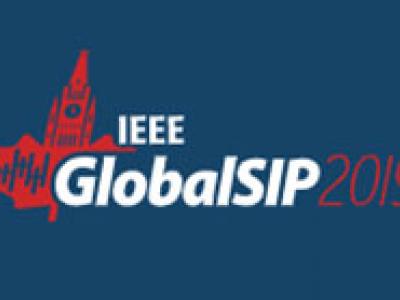
- Read more about Learning About Loads to Improve Power System Operation and Control
- Log in to post comments
- Categories:
- Read more about Integrated Power and D2D Communications Simulator for Future Power Systems
- Log in to post comments
- Categories:
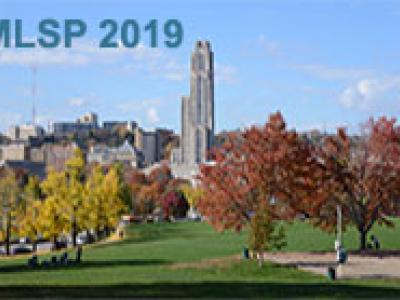
- Read more about COLLABORATIVE INFERENCE OF MISSING SMART ELECTRIC METER DATA FOR A BUILDING
- Log in to post comments
This paper proposes a novel approach to infer missing electricity meter data of a building using a seasonal autoregressive integrated moving average model with exogenous variables (SARIMAX). A cross-correlation function is utilized to identify buildings that have electricity usage patterns similar to the building with missing meter data.
- Categories:
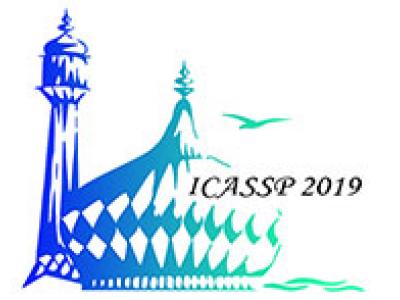
- Read more about Neural Variational Identification and Filtering
- Log in to post comments
- Categories: