Documents
Poster
Enhancing Generalization in Medical Visual Question Answering Tasks via Gradient-Guided Model Perturbation
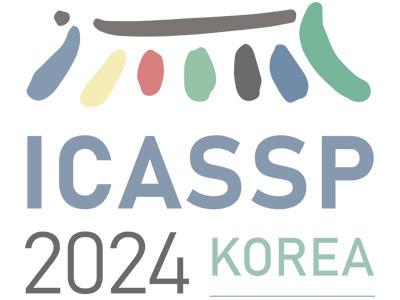
- DOI:
- 10.60864/h0fm-vf80
- Citation Author(s):
- Submitted by:
- Hongyang Li
- Last updated:
- 5 April 2024 - 3:34am
- Document Type:
- Poster
- Document Year:
- 2024
- Event:
- Categories:
- Log in to post comments
Leveraging pre-trained visual language models has become a widely adopted approach for improving performance in downstream visual question answering (VQA) applications. However, in the specialized field of medical VQA, the scarcity of available data poses a significant barrier to achieving reliable model generalization. Numerous methods have been proposed to enhance model generalization, addressing the issue from data-centric and model-centric perspectives. Data augmentation techniques are commonly employed to enrich the dataset, while various regularization approaches aim to prevent model overfitting, especially when training on limited data samples. In this paper, we introduce a method that incorporates gradient-guided parameter perturbations to the visual encoder of the multimodality model during both pre-training and fine-tuning phases, to improve model generalization for downstream medical VQA tasks. The small perturbation is adaptively generated by aligning with the direction of the moving average gradient in the optimization landscape, which is opposite to the directions of the optimizer's historical updates. It is subsequently injected into the model’s visual encoder. The results show that, even with a significantly smaller pre-training image caption dataset, our approach achieves competitive outcomes on both VQA-RAD and SLAKE datasets.
poster_lhy.pdf
