Documents
Poster
ENHANCING LOW-LATENCY SPEAKER DIARIZATION WITH SPATIAL DICTIONARY LEARNING
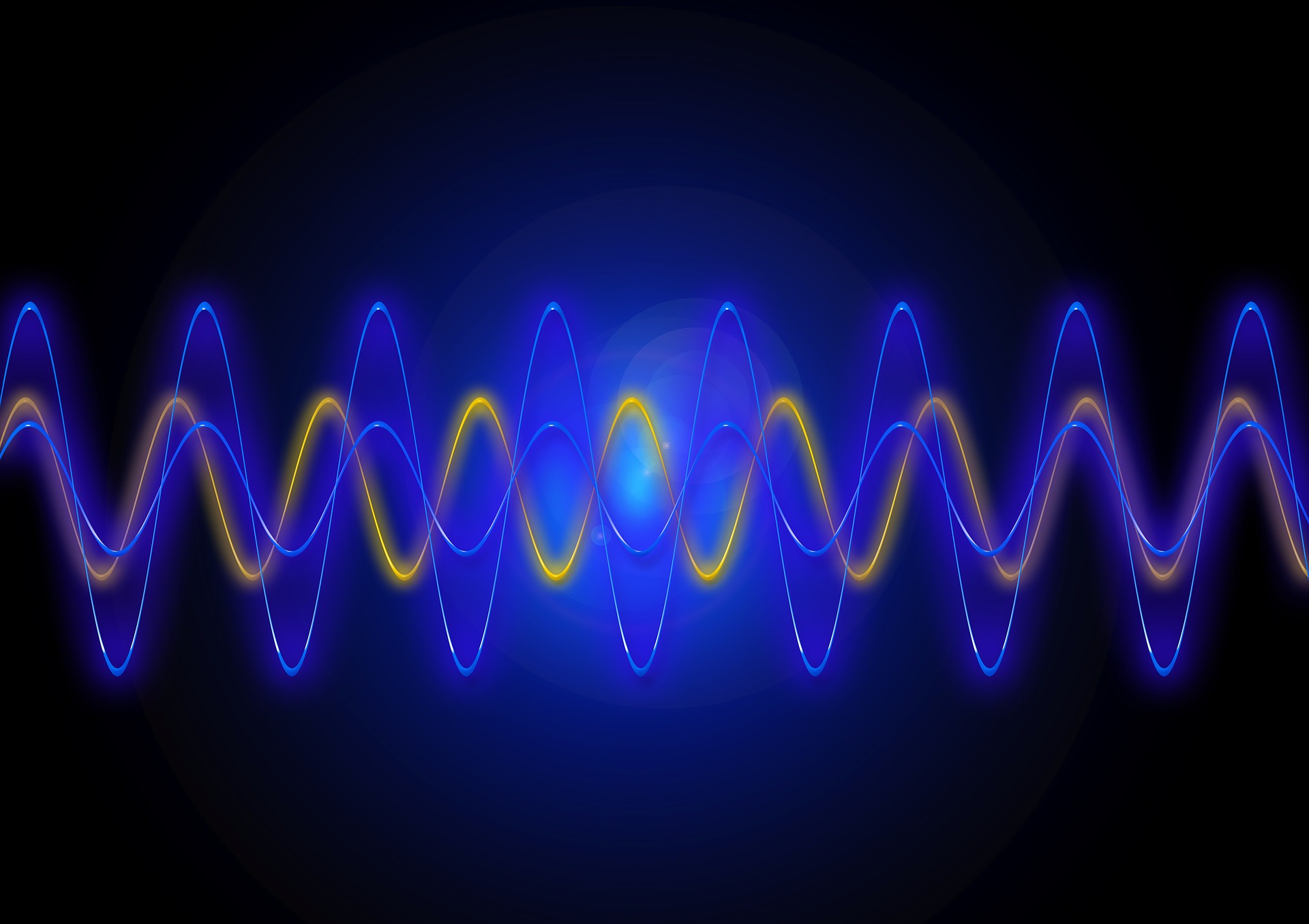
- DOI:
- 10.60864/6t7c-hh06
- Citation Author(s):
- Submitted by:
- Weiguang Chen
- Last updated:
- 6 June 2024 - 10:23am
- Document Type:
- Poster
- Categories:
- Log in to post comments
This study proposes a low-latency online speaker diarization framework.
Specifically, we design a spatial dictionary learning module shared across different frequency bands, enabling spatial feature learning at each frequency bin.
This contributes to reducing the latency constraints of the online diarization system.
Additionally, a magnitude-weighted fusion is devised to integrate spectral features. Consequently, the system can extract discriminative speaker embeddings by simultaneously considering spectral and spatial features.
Experimental results on the Alimeeting dataset demonstrate a significant improvement in diarization error rates across various latencies, with a relative improvement of 45.80\% compared to single-channel online diarization.
Moreover, our method surpasses offline direction-of-arrival-based diarization and achieves comparable performance to the second-ranked offline system of the Alimeeting challenge.