Documents
Poster
ENTROPY BASED PRUNING OF BACKOFF MAXENT LANGUAGE MODELS WITH CONTEXTUAL FEATURES
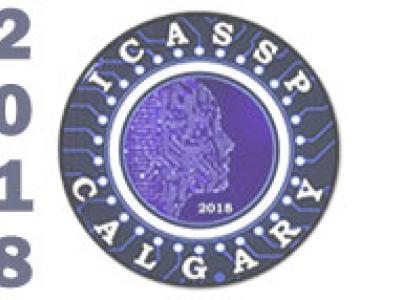
- Citation Author(s):
- Submitted by:
- Tongzhou Chen
- Last updated:
- 19 April 2018 - 2:12pm
- Document Type:
- Poster
- Document Year:
- 2018
- Event:
- Presenters:
- Tongzhou Chen
- Paper Code:
- 3442
- Categories:
- Log in to post comments
In this paper, we present a pruning technique for maximum en- tropy (MaxEnt) language models. It is based on computing the exact entropy loss when removing each feature from the model, and it ex- plicitly supports backoff features by replacing each removed feature with its backoff. The algorithm computes the loss on the training data, so it is not restricted to models with n-gram like features, al- lowing models with any feature, including long range skips, triggers, and contextual features such as device location.
Results on the 1-billion word corpus show large perplexity im- provements relative for frequency pruned models of comparable size. Automatic speech recognition (ASR) experiments show absolute word error rate improvements in a large-scale cloud based mobile ASR system for Italian.
poster.pdf
