Documents
Presentation Slides
Estimating Structural Missing Values via Low-tubal-rank Tensor Completion
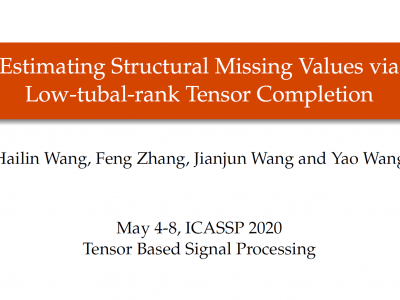
- Citation Author(s):
- Submitted by:
- Hailin Wang
- Last updated:
- 16 April 2020 - 3:15am
- Document Type:
- Presentation Slides
- Document Year:
- 2020
- Event:
- Presenters:
- Hailin Wang
- Categories:
- Keywords:
- Log in to post comments
The recently proposed Tensor Nuclear Norm (TNN) minimization has been widely used for tensor completion. However, previous works didn’t consider the structural difference between the observed data and missing data, which widely exists in many applications. In this paper, we propose to incorporate a constraint item on the missing values into low-tubal-rank tensor completion to promote the structural hypothesis
of the missing values such as sparsity. Theoretically, the proposed model has lower recovery error than classical model, and the target tensor can be recovered exactly with overwhelming probability provided low-tubal-rankness on whole area and sparsity on missing area. Algorithmically, an efficient algorithm by Alternating Direction Method of Multiplier (ADMM) is presented. Extensive experiments on both synthetic and real-world data demonstrate its superiority compared with several state-of-the-art methods.