Documents
Poster
Feature Based Adaptation For Speaking Style Synthesis
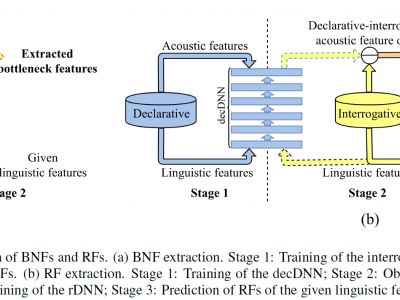
- Citation Author(s):
- Submitted by:
- Xixin Wu
- Last updated:
- 12 April 2018 - 10:14pm
- Document Type:
- Poster
- Document Year:
- 2018
- Event:
- Presenters:
- Xixin Wu
- Paper Code:
- SP-P6
- Categories:
- Log in to post comments
Speaking style plays an important role in the expressivity of speech for communication. Hence speaking style is very important for synthetic speech as well. Speaking style adaptation faces the difficulty that the data of specific styles may be limited and difficult to obtain in large amounts. A possible solution is to leverage data from speaking styles that are more available, to train the speech synthesizer and then adapt it to the target style for which the data is scarce. Conventional DNN adaptation approaches directly update the top layers of a well-trained, style-dependent model towards the target style. The detailed local context-level mismatch between the original and the target styles is not considered. In order to address this issue, two frame-level input feature-based style adaptation techniques are investigated in this paper. We will use style features extracted from (1) a target-style data trained bottleneck DNN, and (2) a novel cross-style residual feature regression DNN. These features are used for top-layer adaptation of a well-trained style-dependent synthesis network. Experimental results on adapting the declarative style to the interrogative style demonstrate the effectiveness of our proposed style features in improving the expressiveness of synthesizing speech for the interrogative style, while maintaining speech quality.