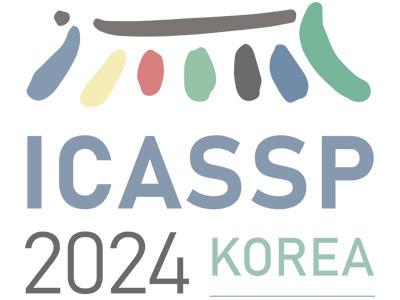
- Read more about VOXTLM: UNIFIED DECODER-ONLY MODELS FOR CONSOLIDATING SPEECH RECOGNITION, SYNTHESIS AND SPEECH, TEXT CONTINUATION TASKS
- Log in to post comments
We propose a decoder-only language model, VoxtLM, that can perform four tasks: speech recognition, speech synthesis, text generation, and speech continuation. VoxtLM integrates text vocabulary with discrete speech tokens from self-supervised speech features and uses special tokens to enable multitask learning. Compared to a single-task model, VoxtLM exhibits a significant improvement in speech synthesis, with improvements in both speech intelligibility from 28.9 to 5.6 and objective quality from 2.68 to 3.90.
- Categories:
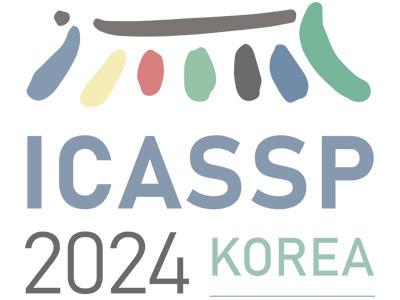
- Read more about VOXTLM: UNIFIED DECODER-ONLY MODELS FOR CONSOLIDATING SPEECH RECOGNITION, SYNTHESIS AND SPEECH, TEXT CONTINUATION TASKS
- Log in to post comments
We propose a decoder-only language model, VoxtLM, that can perform four tasks: speech recognition, speech synthesis, text generation, and speech continuation. VoxtLM integrates text vocabulary with discrete speech tokens from self-supervised speech features and uses special tokens to enable multitask learning. Compared to a single-task model, VoxtLM exhibits a significant improvement in speech synthesis, with improvements in both speech intelligibility from 28.9 to 5.6 and objective quality from 2.68 to 3.90.
- Categories:
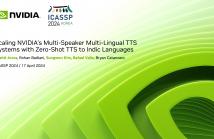
- Read more about Scaling NVIDIA’s Multi-Speaker Multi-Lingual TTS Systems with Zero-Shot TTS to Indic Languages
- Log in to post comments
In this paper, we describe the TTS models developed by NVIDIA for the MMITS-VC (Multi-speaker, Multi-lingual Indic TTS with Voice Cloning) 2024 Challenge. In Tracks 1 and 2, we utilize RAD-MMM to perform few-shot TTS by training additionally on 5 minutes of target speaker data. In Track 3, we utilize P-Flow to perform zero-shot TTS by training on the challenge dataset as well as external datasets. We use HiFi-GAN vocoders for all submissions.
- Categories:
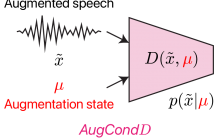
- Read more about Training Generative Adversarial Network-Based Vocoder with Limited Data Using Augmentation-Conditional Discriminator
- Log in to post comments
A generative adversarial network (GAN)-based vocoder trained with an adversarial discriminator is commonly used for speech synthesis because of its fast, lightweight, and high-quality characteristics. However, this data-driven model requires a large amount of training data incurring high data-collection costs. To address this issue, we propose an augmentation-conditional discriminator (AugCondD) that receives the augmentation state as input in addition to speech, thereby assessing input speech according to augmentation state, without inhibiting the learning of the original non-augmented distribution. Experimental results indicate that AugCondD improves speech quality under limited data conditions while achieving comparable speech quality under sufficient data conditions.
- Categories:
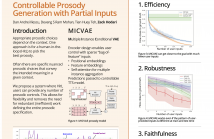
- Read more about Poster - Controllable Prosody Generation With Partial Inputs
- Log in to post comments
Appropriate prosodic choices depend on the context. One approach is for a human-in-the- loop (HitL) to pick the best prosody.
Often there are specific nuanced prosodic choices that convey the intended meaning in a given context.
We propose a system where HitL users can provide any number of prosodic controls. This allows for flexibility and removes the need for redundant (inefficient) work defining the entire prosodic specification.
- Categories:
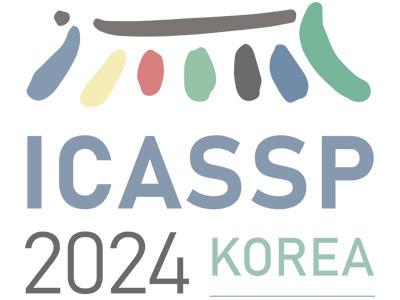
- Read more about TNFORMER: SINGLE-PASS MULTILINGUAL TEXT NORMALIZATION WITH A TRANSFORMER DECODER MODEL
- Log in to post comments
Text Normalization (TN) is a pivotal pre-processing procedure in speech synthesis systems, which converts diverse forms of text into a canonical form suitable for correct synthesis. This work introduces a novel model, TNFormer, which innovatively transforms the TN task into a next token prediction problem, leveraging the structure of GPT with only Transformer decoders for efficient, single-pass TN. The strength of TNFormer lies not only in its ability to identify Non-Standard Words that require normalization but also in its aptitude for context-driven normalization in a single pass.
- Categories:
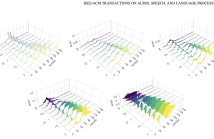
- Read more about Interpreting Intermediate Convolutional Layers of Generative CNNs Trained on Waveforms
- Log in to post comments
This paper presents a technique to interpret and visualize intermediate layers in generative CNNs trained on raw speech data in an unsupervised manner. We argue that averaging over feature maps after ReLU activation in each transpose convolutional layer yields interpretable time-series data. This technique allows for acoustic analysis of intermediate layers that parallels the acoustic analysis of human speech data: we can extract F0, intensity, duration, formants, and other acoustic properties from intermediate layers in order to test where and how CNNs encode various types of information.
- Categories:
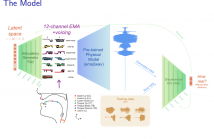
- Read more about Articulation GAN: Unsupervised Modeling of Articulatory Learning
- Log in to post comments
Generative deep neural networks are widely used for speech synthesis, but most existing models directly generate waveforms or spectral outputs. Humans, however, produce speech by controlling articulators, which results in the production of speech sounds through physical properties of sound propagation. We introduce the Articulatory Generator to the Generative Adversarial Network paradigm, a new unsupervised generative model of speech production/synthesis.
- Categories:
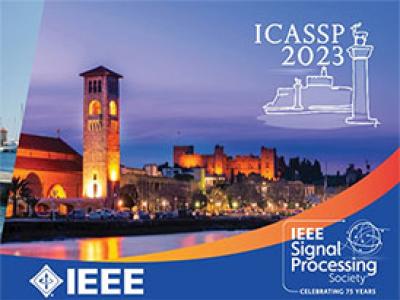
- Read more about Poster
- Log in to post comments
Listening to spoken content often requires modifying the speech rate while preserving the timbre and pitch of the speaker. To date, advanced signal processing techniques are used to address this task, but it still remains a challenge to maintain a high speech quality at all time-scales. Inspired by the success of speech generation using Generative Adversarial Networks (GANs), we propose a novel unsupervised learning algorithm for time-scale modification (TSM) of speech, called ScalerGAN. The model is trained using a set of speech utterances, where no time-scales are provided.
- Categories:
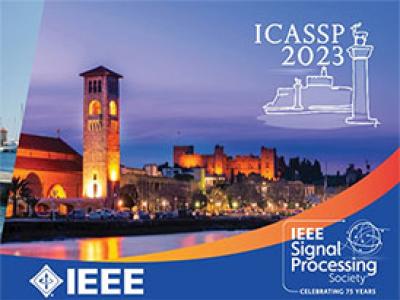
- Read more about Wave-U-Net Discriminator: Fast and Lightweight Discriminator for Generative Adversarial Network-Based Speech Synthesis
- Log in to post comments
This study proposes a Wave-U-Net discriminator, which is a single but expressive discriminator that assesses a waveform in a sample-wise manner with the same resolution as the input signal while extracting multilevel features via an encoder and decoder with skip connections. The experimental results demonstrate that a Wave-U-Net discriminator can be used as an alternative to a typical ensemble of discriminators while maintaining speech quality, reducing the model size, and accelerating the training speed.
- Categories: