Documents
Empirical studies
Food Image Generation on multi-noun categories
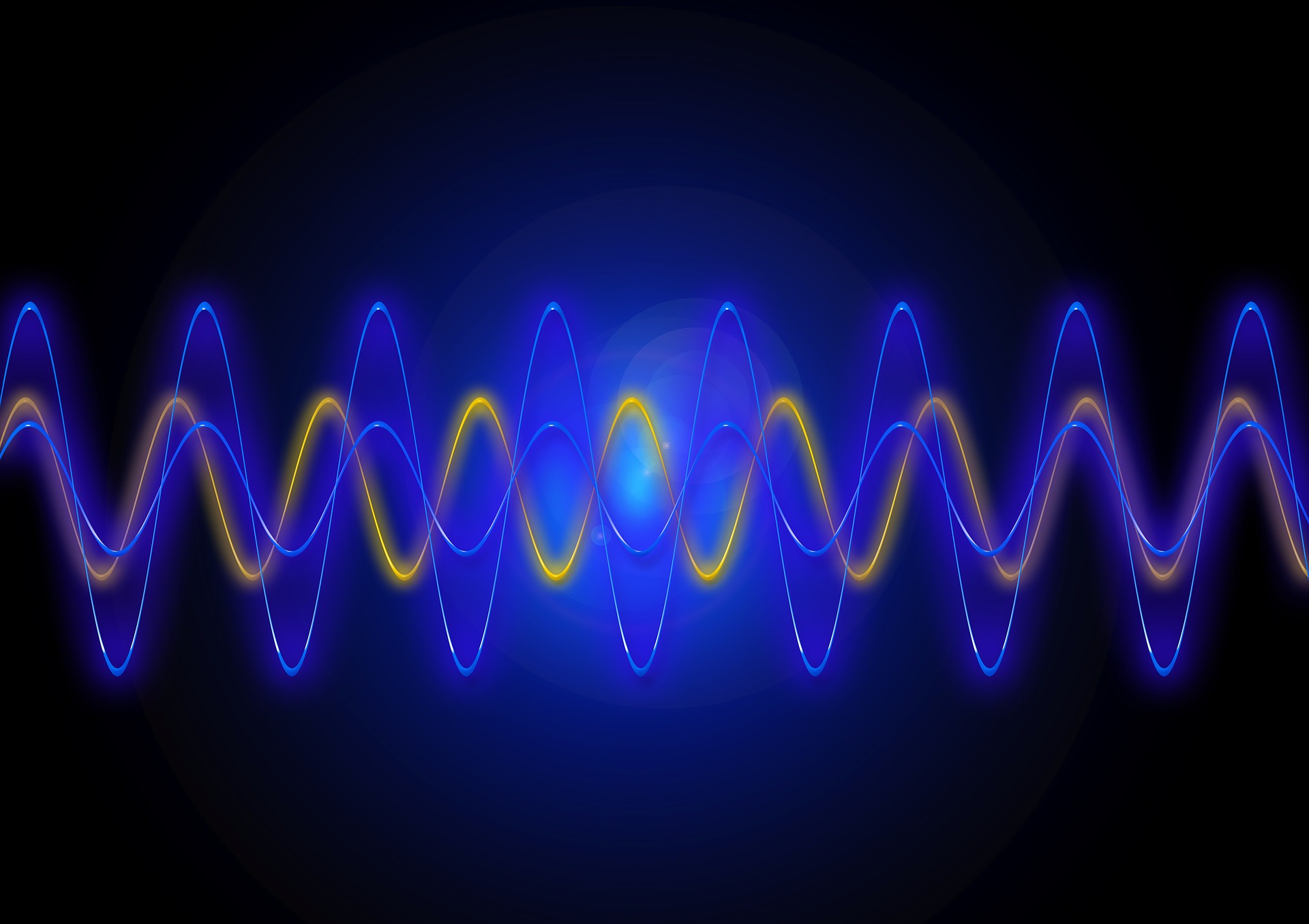
- DOI:
- 10.60864/g5e6-d580
- Citation Author(s):
- Submitted by:
- Xinyue Pan
- Last updated:
- 4 February 2025 - 9:38pm
- Document Type:
- Empirical studies
- Categories:
- Log in to post comments
Food image analysis is a challenging problem due to the diverse and complex appearance of food. Image generation provides a way to aid the data augmentation needed for dealing with this problem so that more data can be used for training a model. In this paper, we tackle a challenge in food image generation where categories consist of multiple nouns (e.g.,“egg sandwich”), often causing models to misinterpret individual components and generate unintended objects. This issue stems from insufficient food domain knowledge in the text encoder and misinterpretation of noun relationships, leading to incorrect spatial layouts. To overcome the issue, we propose FoCULR (Food Category Understanding and Layout Refinement) to fine-tune a text encoder to incorporate food domain knowledge, and improve the image generation process by introducing core concepts early on in the generation process. The proposed method enables the model to better understand the relationships between words within a category, while reducing the likelihood of generating irrelevant objects from individual nouns. Our experimental results demonstrate that the integration of these techniques improves image generation performance in the food domain