Documents
Poster
FOVEA TRANSFORMER: EFFICIENT LONG-CONTEXT MODELING WITH STRUCTURED FINE-TO-COARSE ATTENTION
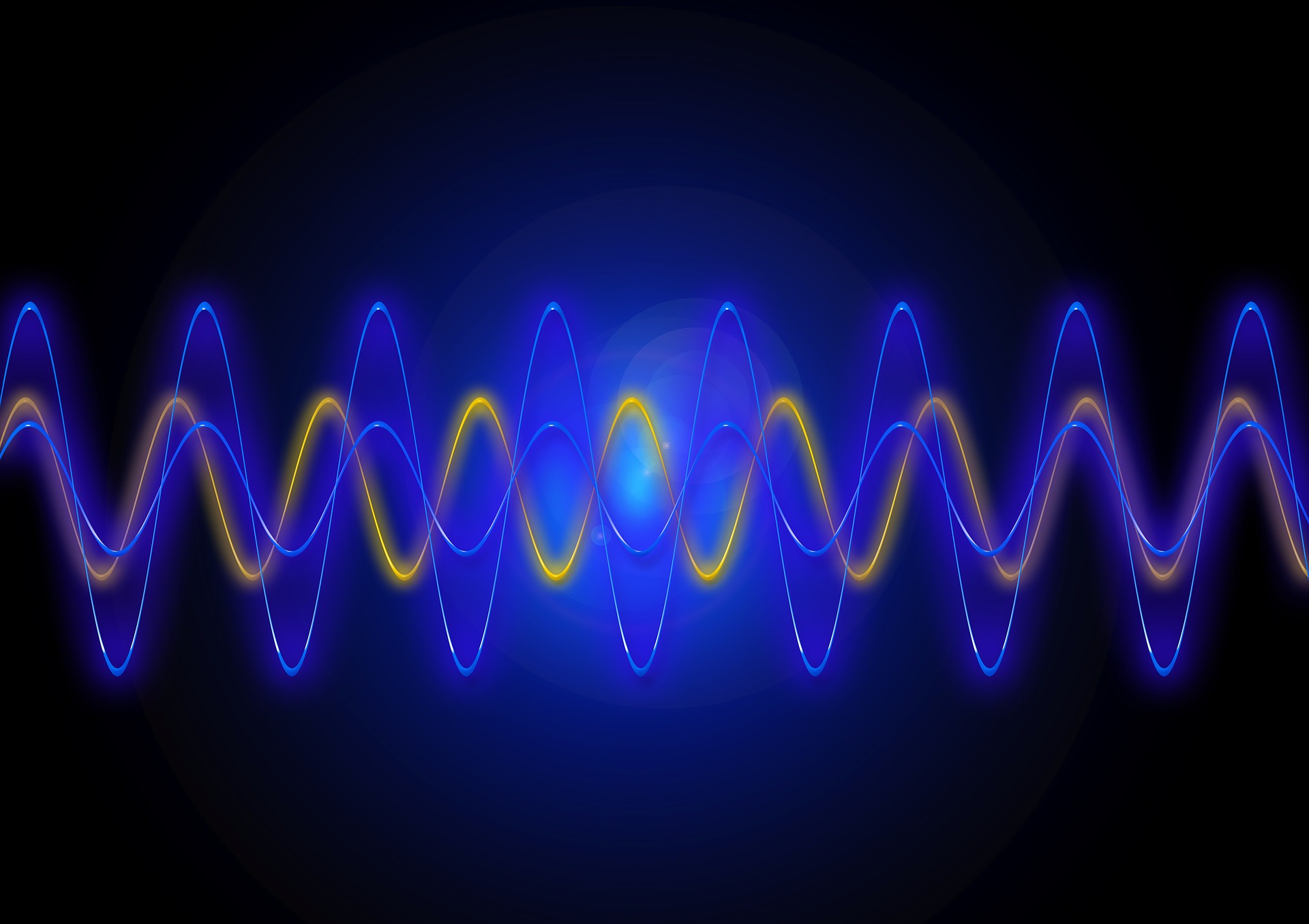
- DOI:
- 10.60864/0pj0-k111
- Citation Author(s):
- Submitted by:
- Ziwei He
- Last updated:
- 6 June 2024 - 10:50am
- Document Type:
- Poster
- Document Year:
- 2024
- Paper Code:
- SLP-P36.10
- Categories:
- Log in to post comments
The quadratic complexity of self-attention in Transformers has hindered the processing of long text. To alleviate this problem, previous works have proposed to sparsify the attention matrix, taking advantage of the observation that crucial information about a token can be derived from its neighbors. These methods typically combine one or another form of local attention and global attention. Such combinations introduce abrupt changes in contextual granularity when going from local to global, which may be undesirable. We believe that a smoother transition could potentially enhance model's ability to capture long-context dependencies. In this study, we introduce Fovea Transformer, a long-context focused transformer that addresses the challenges of capturing global dependencies while maintaining computational efficiency. To achieve this, we construct a multi-scale tree from the input sequence, and use representations of context tokens with a progressively coarser granularity in the tree, as their distance to the query token increases. We evaluate our model on three long-context summarization tasks\footnote{Our code is publicly available at: \textit{https://github.com/ZiweiHe/Fovea-Transformer}}. It achieves state-of-the-art performance on two of them, and competitive results on the third with mixed improvement and setback of the evaluation metrics.