Documents
Poster
Generalized autocorrelation analysis for multi-target detection
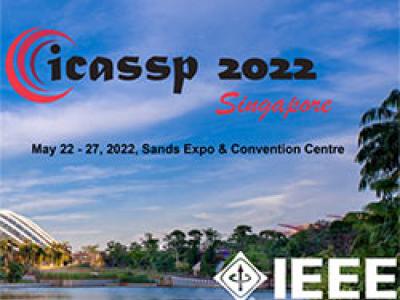
- Citation Author(s):
- Submitted by:
- Shay Kreymer
- Last updated:
- 4 May 2022 - 4:56pm
- Document Type:
- Poster
- Document Year:
- 2022
- Event:
- Presenters:
- Shay Kreymer
- Paper Code:
- SPTM-20.5
- Categories:
- Log in to post comments
We study the multi-target detection problem of recovering a target signal from a noisy measurement that contains multiple copies of the signal at unknown locations. Motivated by the structure reconstruction problem in cryo-electron microscopy, we focus on the high noise regime, where noise hampers accurate detection of signal occurrences. Previous works proposed an autocorrelation analysis framework to estimate the signal directly from the measurement, without detecting signal occurrences. Specifically, autocorrelation analysis entails finding a signal that best matches the observable autocorrelations by minimizing a least squares objective. This paper extends this line of research by developing a generalized autocorrelation analysis framework that replaces the least squares by a weighted least squares. The optimal weights can be computed directly from the data and guarantee favorable statistical properties. We demonstrate signal recovery from highly noisy measurements, and show that the proposed framework outperforms autocorrelation analysis in a wide range of parameters.