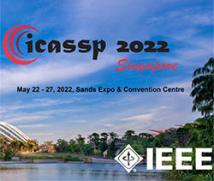
ICASSP 2022 - IEEE International Conference on Acoustics, Speech and Signal Processing is the world’s largest and most comprehensive technical conference focused on signal processing and its applications. The ICASSP 2022 conference will feature world-class presentations by internationally renowned speakers, cutting-edge session topics and provide a fantastic opportunity to network with like-minded professionals from around the world. Visit the website.
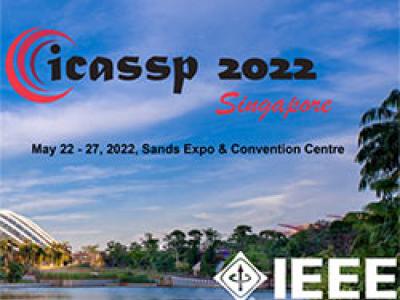
- Read more about SELECTIVE MUTUAL LEARNING: AN EFFICIENT APPROACH FOR SINGLE CHANNEL SPEECH SEPARATION
- Log in to post comments
Mutual learning, the related idea to knowledge distillation, is a group of untrained lightweight networks, which simultaneously learn and share knowledge to perform tasks together during training. In this paper, we propose a novel mutual learning approach, namely selective mutual learning. This is the simple yet effective approach to boost the performance of the networks for speech separation. There are two networks in the selective mutual learning method, they are like a pair of friends learning and sharing knowledge with each other.
- Categories:
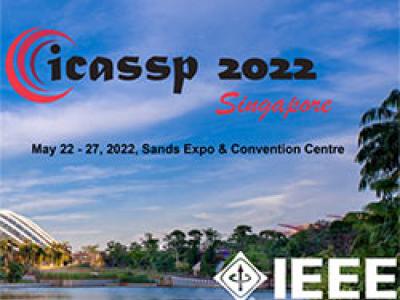
- Read more about IMPQ: Reduced Complexity Neural Networks via Granular Precision Assignment
- Log in to post comments
- Categories:
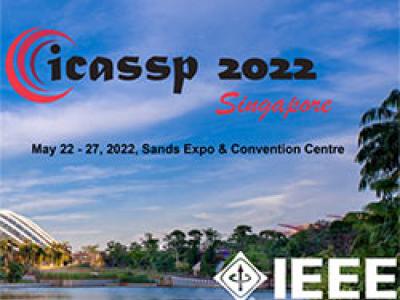
- Read more about saito22icassp_slide
- Log in to post comments
We propose novel deep speaker representation learning that considers perceptual similarity among speakers for multi-speaker generative modeling. Following its success in accurate discriminative modeling of speaker individuality, knowledge of deep speaker representation learning (i.e., speaker representation learning using deep neural networks) has been introduced to multi-speaker generative modeling.
- Categories:
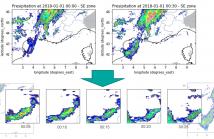
When providing the boundary conditions for hydrological flood models and estimating the associated risk, interpolating precipitation at very high temporal resolutions (e.g. 5 minutes) is essential not to miss the cause of flooding in local regions. In this paper, we study optical flow-based interpolation of globally available weather radar images from satellites.
- Categories:
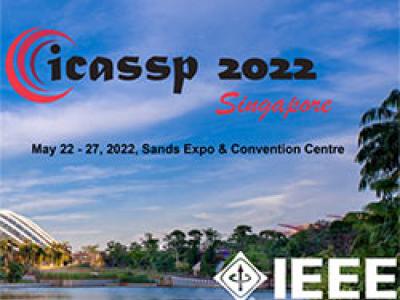
- Read more about On Language Model Integration for RNN Transducer based Speech Recognition
- Log in to post comments
The mismatch between an external language model (LM) and the implicitly learned internal LM (ILM) of RNN-Transducer (RNN-T) can limit the performance of LM integration such as simple shallow fusion. A Bayesian interpretation suggests to remove this sequence prior as ILM correction. In this work, we study various ILM correction-based LM integration methods formulated in a common RNN-T framework. We provide a decoding interpretation on two major reasons for performance improvement with ILM correction, which is further experimentally verified with detailed analysis.
- Categories:
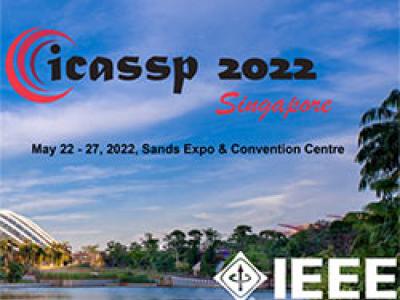
- Read more about ENABLING ON-DEVICE TRAINING OF SPEECH RECOGNITION MODELS WITH FEDERATED DROPOUT
- Log in to post comments
Federated learning can be used to train machine learning models on the edge on local data that never leave devices, providing privacy by default. This presents a challenge pertaining to the communication and computation costs associated with clients’ devices. These costs are strongly correlated with the size of the model being trained, and are significant for state-of-the-art automatic speech recognition models.We propose using federated dropout to reduce the size of client models while training a full-size model server-side.
- Categories:
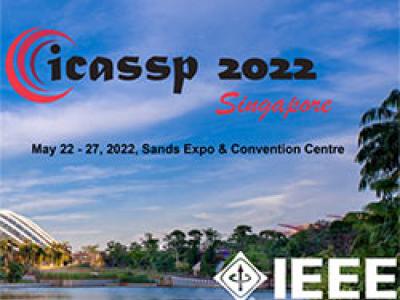
- Read more about ENABLING ON-DEVICE TRAINING OF SPEECH RECOGNITION MODELS WITH FEDERATED DROPOUT
- Log in to post comments
Federated learning can be used to train machine learning models on the edge on local data that never leave devices, providing privacy by default. This presents a challenge pertaining to the communication and computation costs associated with clients’ devices. These costs are strongly correlated with the size of the model being trained, and are significant for state-of-the-art automatic speech recognition models.We propose using federated dropout to reduce the size of client models while training a full-size model server-side.
- Categories:
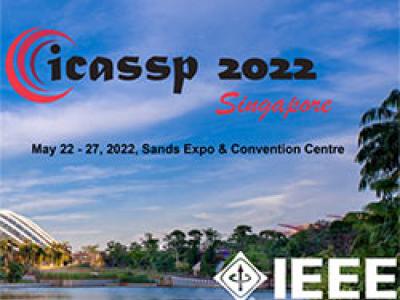
- Read more about ROBUST NONPARAMETRIC DISTRIBUTION FORECAST WITH BACKTEST-BASED BOOTSTRAP AND ADAPTIVE RESIDUAL SELECTION
- Log in to post comments
Distribution forecast can quantify forecast uncertainty and provide various forecast scenarios with their corresponding estimated probabilities. Accurate distribution forecast is crucial for planning - for example when making production capacity or inventory allocation decisions. We propose a practical and robust distribution forecast framework that relies on backtest-based bootstrap and adaptive residual selection.
- Categories:
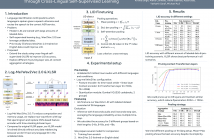
- Read more about ICASSP 2022 - Improved Language Identification Through Cross-Lingual Self-Supervised Learning
- 1 comment
- Log in to post comments
Language identification greatly impacts the success of downstream tasks such as automatic speech recognition. Recently, self-supervised speech representations learned by wav2vec 2.0 have been shown to be very effective for a range of speech tasks. We extend previous self-supervised work on language identification by experimenting with pre-trained models which were learned on real-world unconstrained speech in multiple languages and not just on English.
Slide_ICASSP_LIDW2V.pdf

- Categories: