Documents
Presentation Slides
A Generalized Framework for Domain Adaptation of PLDA in Speaker Recognition
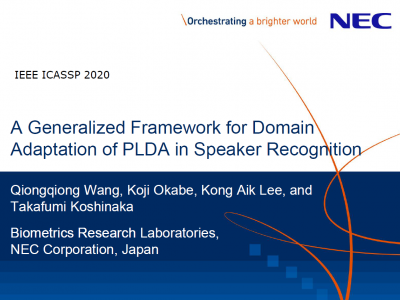
- Citation Author(s):
- Submitted by:
- Qiongqiong Wang
- Last updated:
- 20 May 2020 - 8:49pm
- Document Type:
- Presentation Slides
- Document Year:
- 2020
- Event:
- Categories:
- Keywords:
- Log in to post comments
This paper proposes a generalized framework for domain adaptation of Probabilistic Linear Discriminant Analysis (PLDA) in speaker recognition. It not only includes several existing supervised and unsupervised domain adaptation methods but also makes possible more flexible usage of available data in different domains. In particular, we introduce here the two new techniques described below. (1) Correlation-alignment-based interpolation and (2) covariance regularization. The proposed correlation-alignment-based interpolation method decreases minCprimary up to 30.5% as compared with that from an out-of-domain PLDA model
before adaptation, and minCprimary is also 5.5% lower than with a conventional linear interpolation method with optimal interpolation weights. Further, the proposed regularization technique ensures robustness in interpolations w.r.t. varying
interpolation weights, which in practice is essential.