Documents
Poster
Generating Adaptive and Robust Filter Sets using an Unsupervised Learning Framework
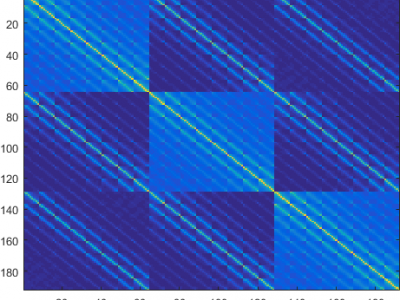
- Citation Author(s):
- Submitted by:
- Mohit Prabhushankar
- Last updated:
- 11 September 2017 - 6:47pm
- Document Type:
- Poster
- Document Year:
- 2017
- Event:
- Presenters:
- Ghassan AlRegib
- Paper Code:
- ICIP-TA-PD.3
- Categories:
- Log in to post comments
In this paper, we introduce an adaptive unsupervised learning framework, which utilizes natural images to train filter sets. The ap- plicability of these filter sets is demonstrated by evaluating their per- formance in two contrasting applications - image quality assessment and texture retrieval. While assessing image quality, the filters need to capture perceptual differences based on dissimilarities between a reference image and its distorted version. In texture retrieval, the filters need to assess similarity between texture images to retrieve closest matching textures. Based on experiments, we show that the filter responses span a set in which a monotonicity-based metric can measure both the perceptual dissimilarity of natural images and the similarity of texture images. In addition, we corrupt the images in the test set and demonstrate that the proposed method leads to robust and reliable retrieval performance compared to existing methods.