Documents
Presentation Slides
HMNet: Hierarchical Microscale-aware Network for Infrared Small Target Detection
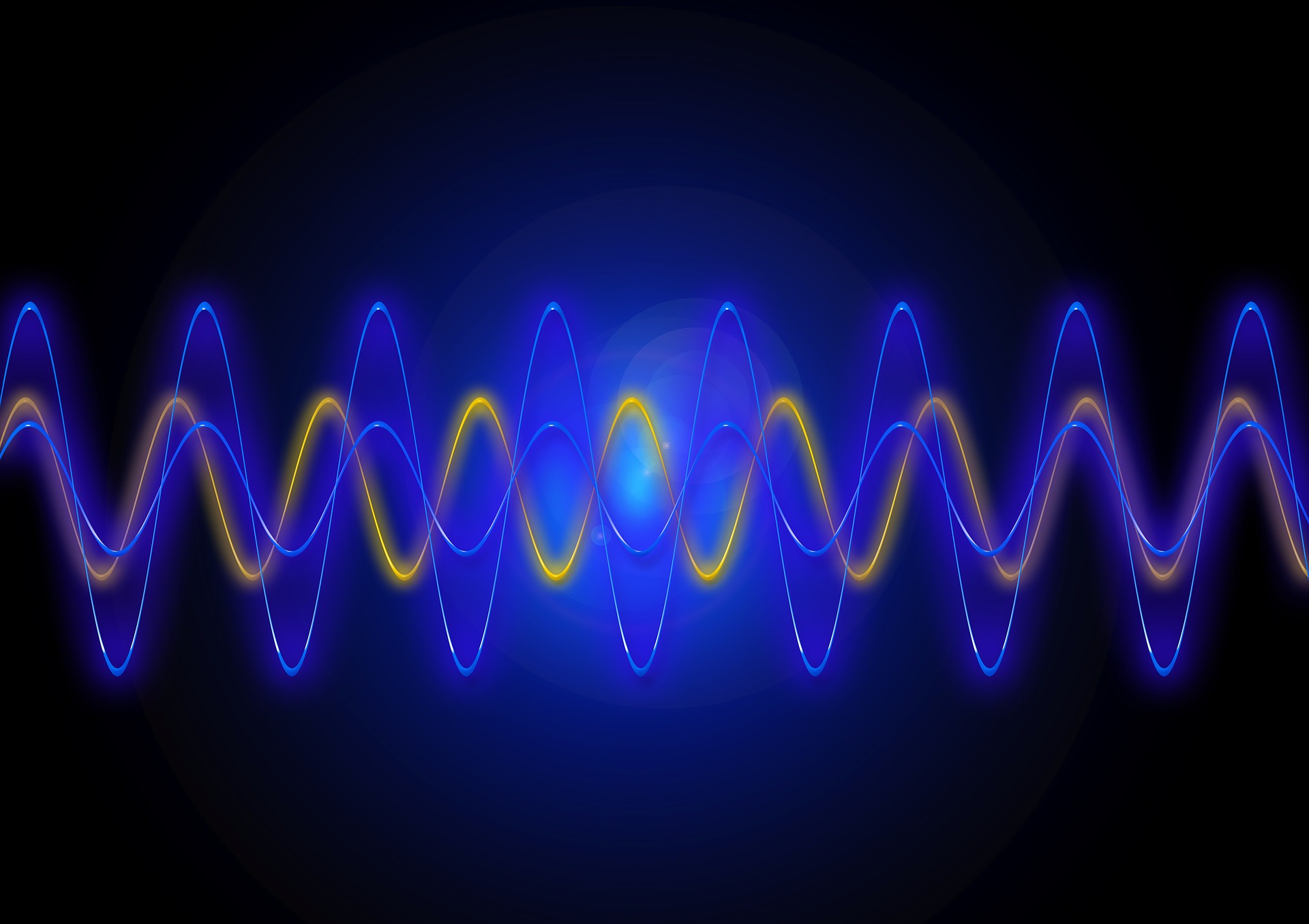
- Citation Author(s):
- Submitted by:
- Yueqian Quan
- Last updated:
- 17 April 2024 - 9:51am
- Document Type:
- Presentation Slides
- Categories:
- Log in to post comments
Compared to the natural image community, infrared target detection suffers more challenges due to the severely tiny and low-contrast objects, especially in cases with obscuration from clutter and noise. The traditional solutions are susceptible to noise interference, which yields suboptimal performance lacking of contour and texture details. Meanwhile, due to the spatial invariance of convolutional layers, most deep learning-based methods locate small targets loosely during feature extraction, leading to serious omissions. To address these limitations, we propose a hierarchical microscale-aware network (HMNet) following an encoder-decoder structure that is mainly equipped with two novel modules: the holistic attention-aware (HAA) module and the scale-aware adaptive extraction (SAE) module. HAA integrates local and global cues via self-attention, depthwise separable convolutions, and dilated convolutions, which hammers at enhancing target features and ensuring accurate localization. As a complement, SAE employs multi-scale features and spatial-channel attention to acquire richer texture details while reducing background noise. The experiments on public datasets demonstrate that our method achieves state-of-the-art performance.