Documents
Research Manuscript
HSBS: COMPREHENSIVE BOOSTING OF FACIAL EXPRESSION RECOGNITION VIA HIERARCHICAL SEMANTIC AND BATCH-WISE SIMILARITY
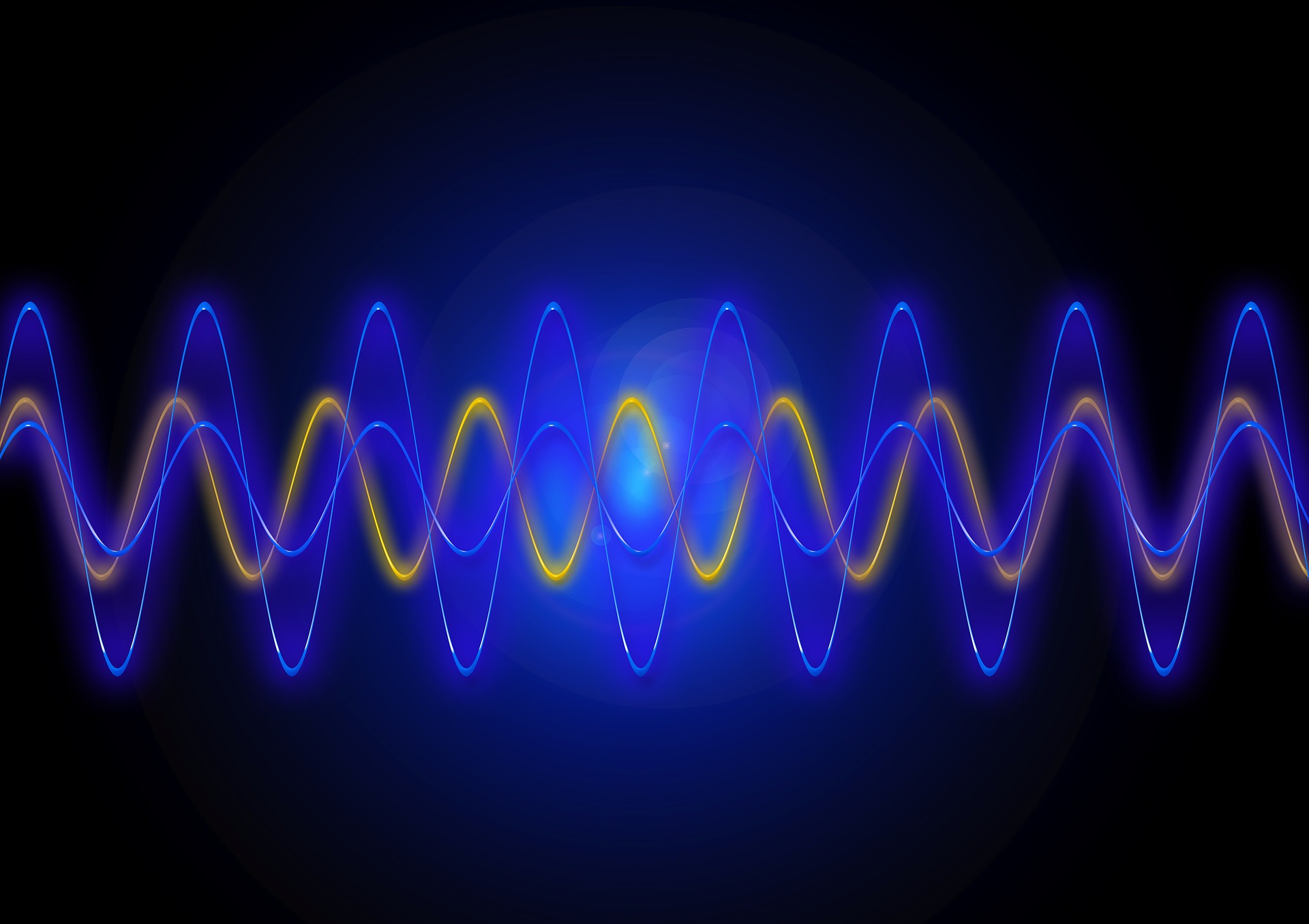
- Citation Author(s):
- Submitted by:
- wang wang
- Last updated:
- 22 January 2025 - 7:54am
- Document Type:
- Research Manuscript
- Categories:
- Log in to post comments
Facial Expression Recognition (FER) has achieved significant success in recent years due to the rise of deep learning. Meanwhile, latent semantic information is crucial for recognizing facial expressions with subtle differences. Inspired by inconsistencies in learning intensity across different layers of deep learning networks — where shallow-layer features lack generalization and task relevance compared to deep-layer features — we propose a novel Hierarchical Semantic Transfer (HST) method. This method uses attention maps from deep layer features to regularize the model, enabling it to effectively capture the necessary semantic information and align the latent semantics between shallow and deep features, while ignoring irrelevant noise. Furthermore, to address the issue of class imbalance present in FER datasets, we introduce a Batch-wise Similarity Attention (BSA) mechanism to learn the similarity relationships between features of different samples. Extensive experiments on different datasets and backbones demonstrate that HSBS can work together to significantly improve model performance and achieve state-of-the art results on those FER datasets.