Documents
ICIP2025_Supplementary Materials
ICIP2025_3D_360ExtremelySparseViews
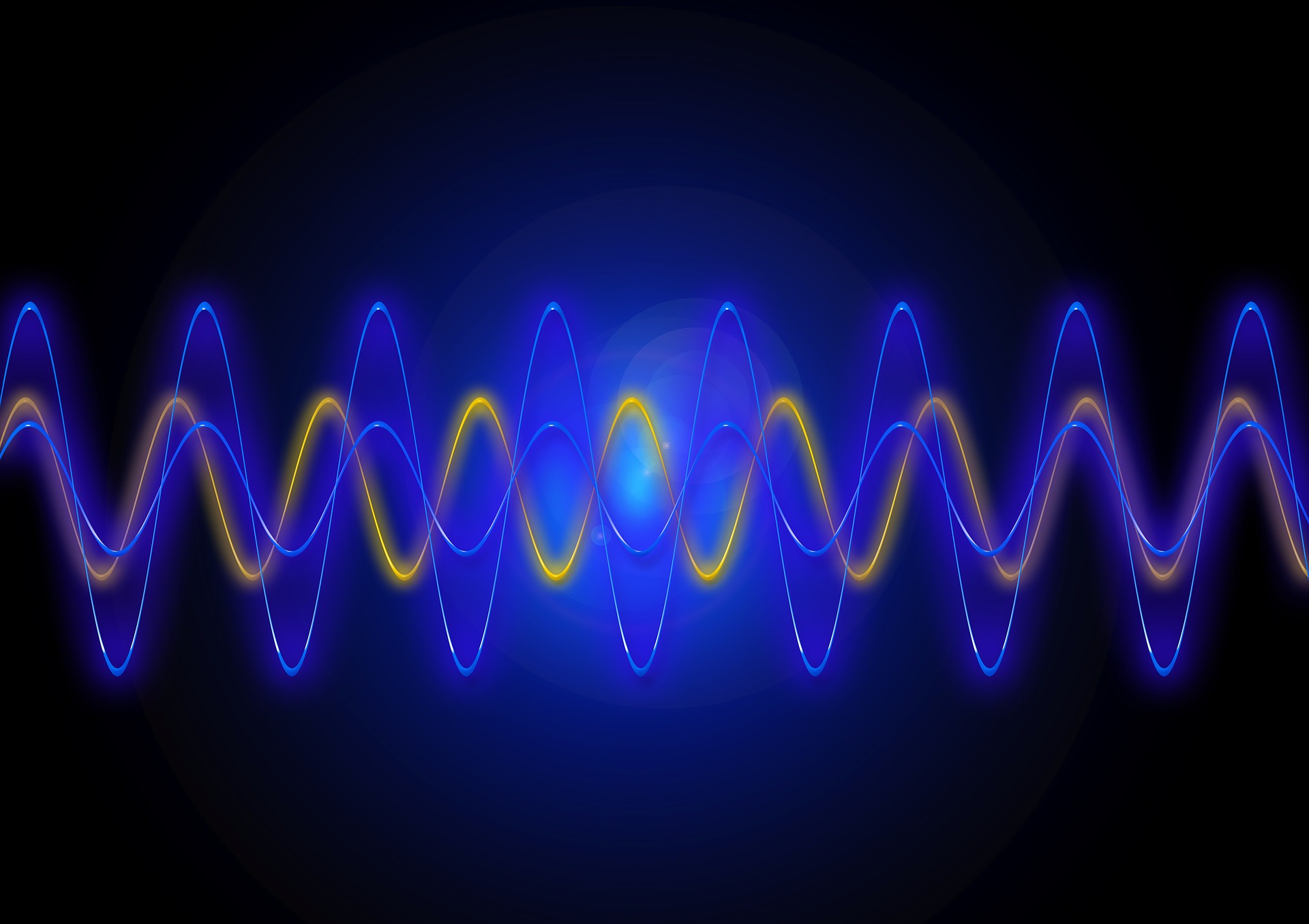
- DOI:
- 10.60864/sw3b-1w78
- Citation Author(s):
- Submitted by:
- Guangan Chen
- Last updated:
- 22 January 2025 - 6:13pm
- Document Type:
- ICIP2025_Supplementary Materials
- Categories:
- Log in to post comments
Novel view synthesis in 360$^\circ$ scenes from extremely sparse input views is essential for applications like virtual reality and augmented reality. This paper presents a novel framework for novel view synthesis in extremely sparse-view cases. As typical structure-from-motion methods are unable to estimate camera poses in extremely sparse-view cases, we apply DUSt3R to estimate camera poses and generate a dense point cloud. Using the poses of estimated cameras, we densely sample additional views from the upper hemisphere space of the scenes, from which we render synthetic images together with the point cloud. Training on a combination of reference images from sparse views and densely sampled synthetic images allows a larger scene coverage in 3D space, addressing the overfitting challenge due to the limited input in sparse-view cases. Retraining a diffusion-based image enhancement model on our created dataset, we further improve the quality of the point-cloud-rendered images by removing artifacts. We compare our framework with benchmark methods in cases of only four input views, demonstrating significant improvement in novel view synthesis under extremely sparse-view conditions for 360$^\circ$ scenes.