Documents
Poster
IDEAL: Improved DEnse LocAL Contrastive Learning for Semi-Supervised Medical Image Segmentation
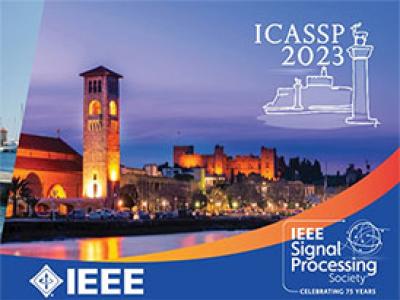
- Citation Author(s):
- Submitted by:
- Hritam Basak
- Last updated:
- 19 May 2023 - 3:04am
- Document Type:
- Poster
- Document Year:
- 2023
- Event:
- Presenters:
- Hritam Basak
- Paper Code:
- 538
- Categories:
- Log in to post comments
Due to the scarcity of labeled data, Contrastive Self-Supervised Learning (SSL) frameworks have lately shown great potential in several medical image analysis tasks. However, the existing contrastive mechanisms are sub-optimal for dense pixel-level segmentation tasks due to their inability to mine local features. To this end, we extend the concept of metric learning to the segmentation task, using a dense (dis)similarity learning for pre-training a deep encoder network, and employing a semi-supervised paradigm to fine-tune for the downstream task. Specifically, we propose a simple convolutional projection head for obtaining dense pixel-level features, and a new contrastive loss to utilize these dense projections thereby improving the local representations. A bidirectional consistency regularization mechanism involving two-stream model training is devised for the downstream task. Upon comparison, our IDEAL method outperforms the SoTA methods by fair margins on cardiac MRI segmentation. Code is available at: https://github.com/Rohit-Kundu/IDEAL-ICASSP23