Documents
Poster
Improving the Capacity of Very Deep Networks with Maxout Units
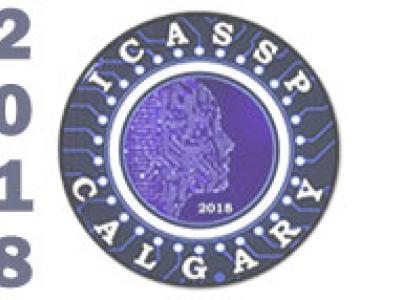
- Citation Author(s):
- Submitted by:
- Oyebade Oyedotun
- Last updated:
- 12 April 2018 - 11:43am
- Document Type:
- Poster
- Document Year:
- 2018
- Event:
- Presenters:
- Oyebade Oyedotun
- Paper Code:
- 2755
- Categories:
- Log in to post comments
Deep neural networks inherently have large representational power for approximating complex target functions. However,
models based on rectified linear units can suffer reduction in representation capacity due to dead units. Moreover, approximating very deep networks trained with dropout at test time can be more inexact due to the several layers of nonlinearities. To address the aforementioned problems, we propose to learn the activation functions of hidden units for very deep networks via maxout. However, maxout units increase the model parameters, and therefore model may suffer from
overfitting; we alleviate this problem by employing elastic net regularization. In this paper, we propose very deep networks with maxout units and elastic net regularization and show that the features learned are quite linearly separable. We perform extensive experiments and reach state-of-the-art results on the USPS and MNIST datasets. Particularly, we reach an error rate of 2.19% on the USPS dataset, surpassing the human performance error rate of 2.5% and all previously reported results, including those that employed training data augmentation. On the MNIST dataset, we reach an error rate of 0.36% which is competitive with the state-of-the-art results.