Documents
Poster
Invertible Resampling-based Layered Image Compression
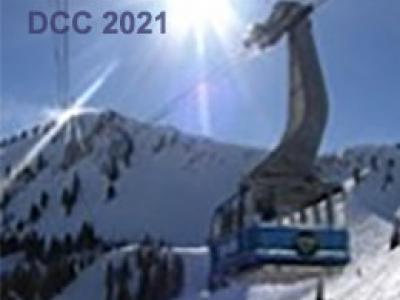
- Citation Author(s):
- Submitted by:
- YOUMIN XU
- Last updated:
- 11 March 2021 - 12:41pm
- Document Type:
- Poster
- Document Year:
- 2021
- Event:
- Presenters:
- Youmin Xu
- Paper Code:
- 154
- Categories:
- Log in to post comments
Flow-based generative models are successfully applied in image generation tasks, where an invertible neural network (INN) is built up based on flow steps. Learning-based compression commonly transforms the input into a compact space and then implements a reconstruction network in the decoder accordingly. By utilizing low-resolution images, traditional or adaptive downsamplers with their corresponding traditional or learned upsamplers usually achieve better coding quality at a low bit-rate. This paper proposes a novel image compression framework named Invertible Resampling-based Layered Image Compression (IRLIC). The rescaling network is built by splitting the input image into a downsampled image and a high-frequency part which is transformed into pre-defined distribution by INN, with symmetrical upsampling. Thus, reliable rescaling is applied in the total lossy compression framework, where only the downsampled image and the reconstruction residual are needed to recover a compressed image. Our IRLIC achieves superior performance to the current methods like BPG and other learning-based image compressions at the bit-rate below 1.8bpp.
script.pptx

script.pptx
