Documents
Poster
INVESTIGATING LABEL NOISE SENSITIVITY OF CONVOLUTIONAL NEURAL NETWORKS FOR FINE GRAINED AUDIO SIGNAL LABELLING
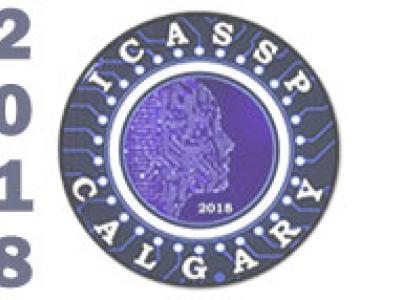
- Citation Author(s):
- Submitted by:
- Rainer Kelz
- Last updated:
- 23 April 2018 - 9:01pm
- Document Type:
- Poster
- Document Year:
- 2018
- Event:
- Presenters:
- Rainer Kelz
- Categories:
- Log in to post comments
We measure the effect of small amounts of systematic and
random label noise caused by slightly misaligned ground truth
labels in a fine grained audio signal labeling task. The task
we choose to demonstrate these effects on is also known as
framewise polyphonic transcription or note quantized multi-
f0 estimation, and transforms a monaural audio signal into a
sequence of note indicator labels. It will be shown that even
slight misalignments have clearly apparent effects, demonstrating a great sensitivity of convolutional neural networks
to label noise. The implications are clear: when using convolutional neural networks for fine grained audio signal label-
ing tasks, great care has to be taken to ensure that the annotations have precise timing, and are free from systematic or
random error as much as possible - even small misalignments
will have a noticeable impact.