Documents
supplementary figures and tables
INVESTIGATING ROBUSTNESS OF UNSUPERVISED STYLEGAN IMAGE RESTORATION
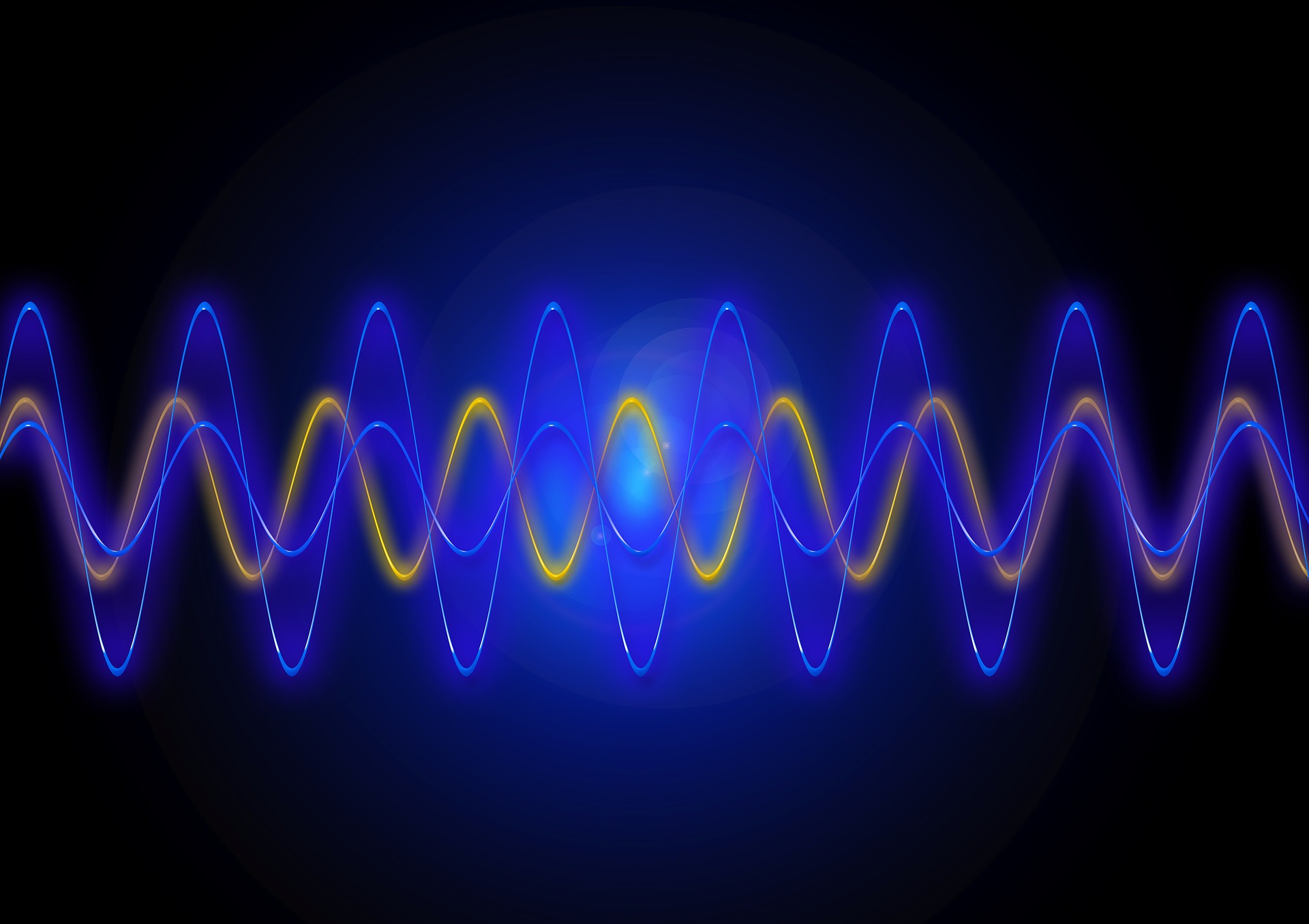
- Citation Author(s):
- Submitted by:
- AKBAR ALI
- Last updated:
- 5 February 2025 - 3:48pm
- Document Type:
- supplementary figures and tables
- Categories:
- Log in to post comments
Recently, generative priors have shown significant improvement for unsupervised image restoration. This study explores the incorporation of multiple loss functions that capture various perceptual and structural aspects of image quality. Our proposed method improves robustness across multiple tasks, including denoising, upsampling, inpainting, and deartifacting, by utilizing a comprehensive loss function based on Learned Perceptual Image Patch Similarity(LPIPS), Multi-Scale Structural Similarity Index Measure Loss(MS-SSIM), Consistency, Feature, and Gradient losses. The experimental results demonstrate marked improvements in accuracy, fidelity, and visual realism in unsupervised image restoration, showcasing the effectiveness of our approach in delivering high-quality results. The experimental results validate the superiority of our approach and offer a promising direction for future advancements in generative-based image restoration methods.