Documents
Presentation Slides
Joint Unsupervised and Supervised Training for Multilingual ASR
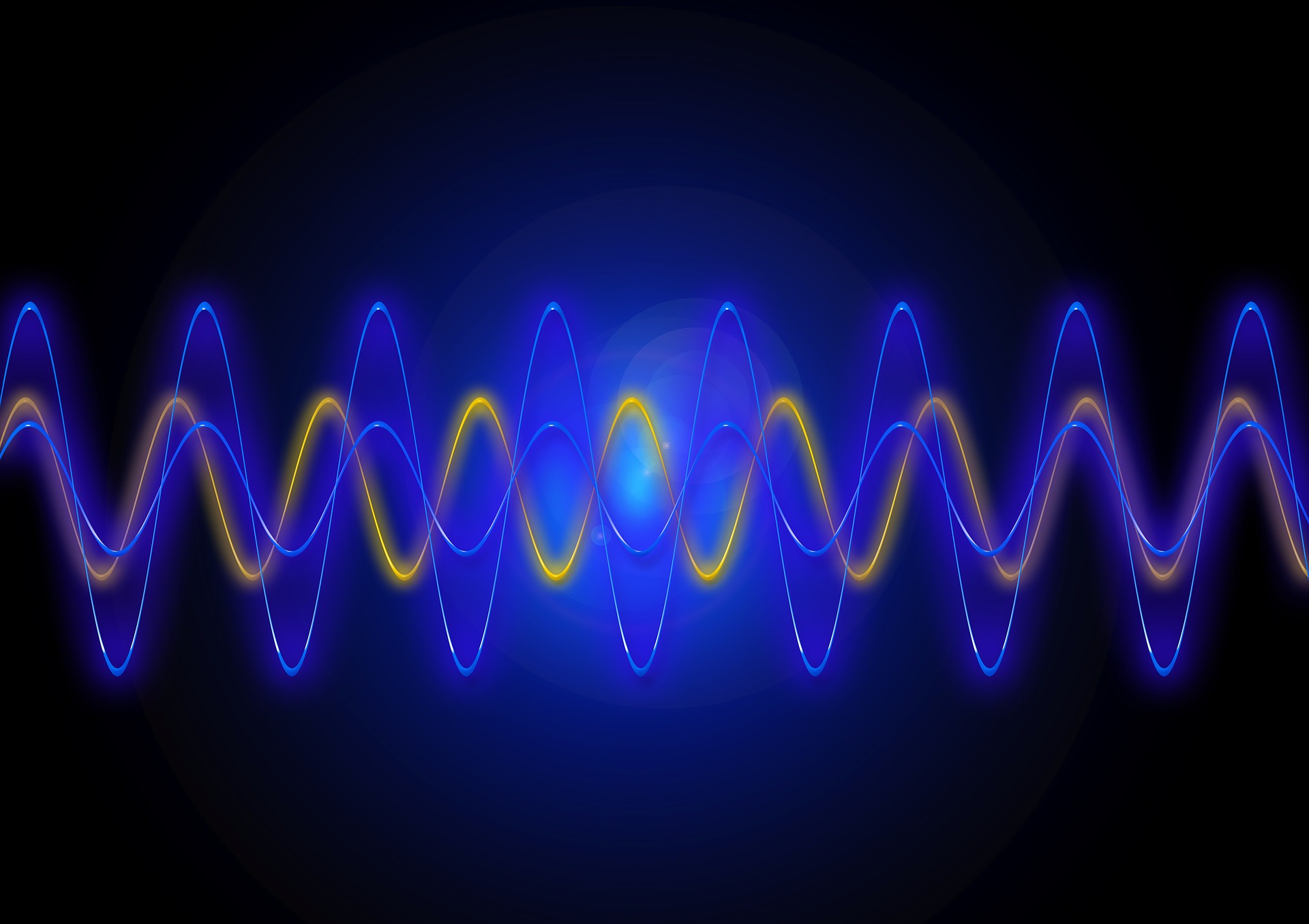
- Citation Author(s):
- Submitted by:
- Junwen Bai
- Last updated:
- 4 May 2022 - 4:54pm
- Document Type:
- Presentation Slides
- Document Year:
- 2022
- Presenters:
- Junwen Bai
- Categories:
- Log in to post comments
Self-supervised training has shown promising gains in pretraining models and facilitating the downstream finetuning for speech recognition, like multilingual ASR. Most existing methods adopt a 2-stage scheme where the self-supervised loss is optimized in the first pretraining stage, and the standard supervised finetuning resumes in the second stage. In this paper, we propose an end-to-end (E2E) Joint Unsupervised and Supervised Training (JUST) method to combine the supervised RNN-T loss and the self-supervised contrastive and masked language modeling (MLM) losses. We validate its performance on the public dataset Multilingual LibriSpeech (MLS), which includes 8 languages and is extremely imbalanced. On MLS, we explore (1) JUST trained from scratch, and (2) JUST finetuned from a pretrained checkpoint. Experiments show that JUST can consistently outperform other existing state-of-the-art methods, and beat the monolingual baseline by a significant margin, demonstrating JUST’s capability of handling low-resource languages in multilingual ASR. Our average WER of all languages outperforms average monolingual baseline by 33.3%, and the state-of-the-art 2-stage XLSR by 32%. On low-resource languages like Polish, our WER is less than half of the monolingual baseline and even beats the supervised transfer learning method which uses external supervision.
poster.pdf
