Documents
Presentation Slides
Kernelized Dense Layers For Facial Expression Recognition
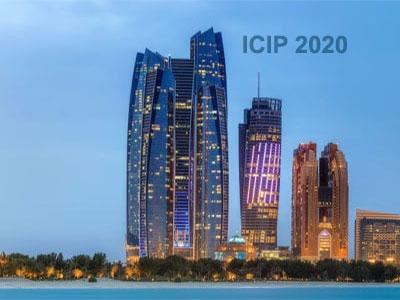
- Citation Author(s):
- Submitted by:
- Mohamed Amine M...
- Last updated:
- 2 November 2020 - 4:37pm
- Document Type:
- Presentation Slides
- Document Year:
- 2020
- Event:
- Presenters:
- Mohamed Amine Mahmoudi
- Paper Code:
- #2488
- Categories:
- Log in to post comments
Fully connected layer is an essential component of Convolutional Neural Networks (CNNs), which demonstrates its efficiency in computer vision tasks. The CNN process usually starts with convolution and pooling layers that first break down the input images into features, and then analyze them independently. The result of this process feeds into a fully connected neural network structure which drives the final classification decision. In this paper, we propose a Kernelized Dense Layer (KDL) which captures higher order feature interactions instead of conventional linear relations. We apply this method to Facial Expression Recognition (FER) and evaluate its performance on RAF, FER2013 and ExpW datasets. The experimental results demonstrate the benefits of such layer and show that our model achieves competitive results with respect to the state-of-the-art approaches.
Comments
Kernelized Dense Layers For Facial Expression Recognition
Fully connected layer is an essential component of Convolutional Neural Networks (CNNs), which demonstrates its efficiency in computer vision tasks. The CNN process usually starts with convolution and pooling layers that first break down the input images into features, and then analyze them independently. The result of this process feeds into a fully connected neural network structure which drives the final classification decision. In this paper, we propose a Kernelized Dense Layer (KDL) which captures higher order feature interactions instead of conventional linear relations. We apply this method to Facial Expression Recognition (FER) and evaluate its performance on RAF, FER2013 and ExpW datasets. The experimental results demonstrate the benefits of such layer and show that our model achieves competitive results with respect to the state-of-the-art approaches.