Documents
Poster
l0-norm Feature LMS Algorithms
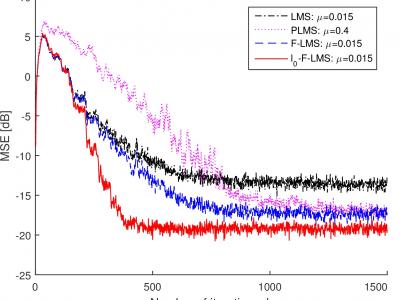
- Citation Author(s):
- Submitted by:
- Hamed Yazdanpanah
- Last updated:
- 16 November 2018 - 4:29pm
- Document Type:
- Poster
- Document Year:
- 2018
- Event:
- Presenters:
- José Antonio Apolinário Jr.
- Paper Code:
- GS-P.5.10
- Categories:
- Log in to post comments
A class of algorithms known as feature least-mean-square (FLMS) has been proposed recently to exploit hidden sparsity
in adaptive filter parameters. In contrast to common sparsityaware adaptive filtering algorithms, the F-LMS algorithm detects and exploits sparsity in linear combinations of filter coefficients. Indeed, by applying a feature matrix to the adaptive filter coefficients vector, the F-LMS algorithm can reveal and exploit their hidden sparsity. However, in many cases the unknown plant to be identified contains not only hidden but also plain sparsity and the F-LMS algorithm is unable to exploit it. Therefore, we can incorporate sparsity-promoting techniques into the F-LMS algorithm in order to allow the exploitation of plain sparsity. In this paper, by utilizing the l0-norm, we propose the l0-norm F-LMS (l0-F-LMS) algorithm for sparse lowpass and sparse highpass systems. Numerical results show that the proposed algorithm outperforms the F-LMS algorithm when dealing with hidden sparsity, particularly in highly sparse systems where the convergence rate is sped up significantly.