Documents
Presentation Slides
Language and Noise Transfer in Speech Enhancement Generative Adversarial Network
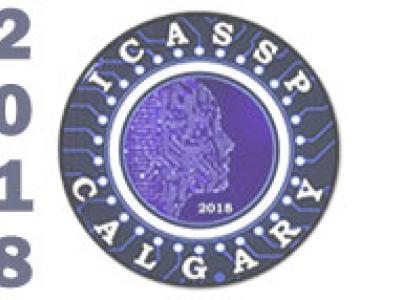
- Citation Author(s):
- Submitted by:
- Santi Pascual
- Last updated:
- 19 April 2018 - 4:40pm
- Document Type:
- Presentation Slides
- Document Year:
- 2018
- Event:
- Presenters:
- Santiago Pascual
- Paper Code:
- 4272
- Categories:
- Log in to post comments
Speech enhancement deep learning systems usually require large amounts of training data to operate in broad conditions or real applications. This makes the adaptability of those systems into new, low resource environments an important topic. In this work, we present the results of adapting a speech enhancement generative adversarial network by fine-tuning the generator with small amounts of data. We investigate the minimum requirements to obtain a stable behavior in terms of several objective metrics in two very different languages: Catalan and Korean. We also study the variability of test performance to unseen noise as a function of the amount of different types of noise available for training. Results show that adapting a pre-trained English model with 10\,min of data already achieves a comparable performance to having two orders of magnitude more data. They also demonstrate the relative stability in test performance with respect to the number of training noise types.