Documents
Presentation Slides
LEARNABLE CONTEXTUAL REGULARIZATION FOR SEMANTIC SEGMENTATION OF INDOOR SCENE IMAGES
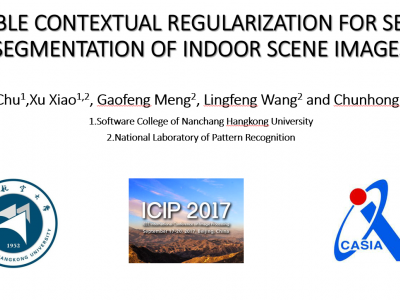
- Citation Author(s):
- Submitted by:
- xiao xu
- Last updated:
- 15 September 2017 - 7:33am
- Document Type:
- Presentation Slides
- Document Year:
- 2017
- Event:
- Presenters:
- Xu Xiao
- Paper Code:
- 1953
- Categories:
- Log in to post comments
Semantic segmentation of indoor scene images has a wide range of
applications. However, due to a large number of classes and uneven
distribution in indoor scenes, mislabels are often made when facing
small objects or boundary regions. Technically, contextual infor-
mation may benefit for segmentation results, but has not yet been
exploited sufficiently. In this paper, we propose a learnable contex-
tual regularization model for enhancing the semantic segmentation
results of color indoor scene images. This regularization model is
combined with a deep convolutional segmentation network without
significantly increasing the number of additional parameters. Our
model, derived from the inherent contextual regularization on the
indoor scene objects, benefits much from the learnable constrain-
t layers bridging the lower layers and the higher layers in the deep
convolutional network. The constraint layers are further integrated
with a weighted L1-norm based contextual regularization between
the neighboring pixels of RGB values to improve the segmenta-
tion results. Experimental results on NYUDv2 indoor scene dataset
demonstrate the effectiveness and efficiency of the proposed method.