Documents
Poster
Learning-Based Antenna Selection for Multicasting
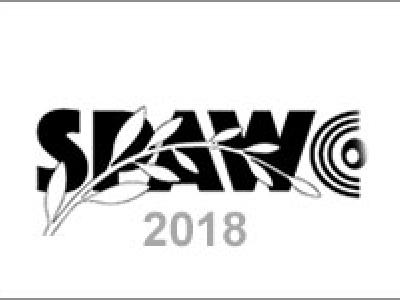
- Citation Author(s):
- Submitted by:
- Mohamed S. Ibrahim
- Last updated:
- 24 June 2018 - 12:17pm
- Document Type:
- Poster
- Document Year:
- 2018
- Event:
- Presenters:
- Mohamed S. Ibrahim
- Paper Code:
- NNAS-TA.S1
- Categories:
- Log in to post comments
In multi-antenna systems, it is preferred to activate only a subset of the available transmit antennas in order to save hardware and energy resources, without seriously degrading the system performance. However, antenna selection often poses very hard optimization problems. Joint multicast beamforming and antenna selection is one particular example, which is often approached by Semi-Definite Relaxation (SDR) type approximations. The drawback is that SDR lifts the problem to a much higher dimension, leading to considerably high memory and computational complexities. In this paper, we propose a machine learning based approach to circumvent the complexity issues. Specifically, we propose a neural network-based approach that aims at selecting a subset of antennas that maximizes the minimum signal to noise ratio at the receivers. The idea is to learn a mapping function (represented by a neural network) that maps channel realizations to antenna selection solutions from massive simulated data. This way, the computational burden of antenna selection can be shifted to off-line neural network training. Experiments demonstrate the efficacy of the proposed machine learning approach relative to the prior state-of-art.