Documents
Poster
Learning from the best: A teacher-student multilingual framework for low-resource languages
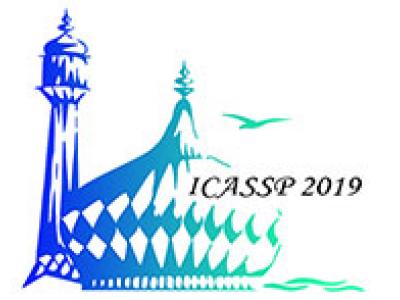
- Citation Author(s):
- Submitted by:
- Deblin Bagchi
- Last updated:
- 13 May 2019 - 5:43pm
- Document Type:
- Poster
- Document Year:
- 2019
- Event:
- Presenters:
- Deblin Bagchi
- Paper Code:
- SLP-P3.1
- Categories:
- Log in to post comments
The traditional method of pretraining neural acoustic models in low-resource languages consists of initializing the acoustic model parameters with a large, annotated multilingual corpus and can be a drain on time and resources. In an attempt to reuse TDNN-LSTMs already pre-trained using multilingual training, we have applied Teacher-Student (TS) learning as a method of pretraining to transfer knowledge from a multilingual TDNN-LSTM to a TDNN. The pretraining time is reduced by an order of magnitude with the use of language-specific data during the teacher-student training. Additionally, the TS architecture allows us to leverage untranscribed data, previously untouched during supervised training. The best student TDNN achieves a WER within 1% of the teacher TDNN-LSTM performance and shows consistent improvement in recognition over TDNNs trained using the traditional pipeline over all the evaluation languages. Switching to TDNN from TDNN-LSTM also allows sub-real time decoding.