Documents
Presentation Slides
Learning Product Graphs from Multidomain Signals
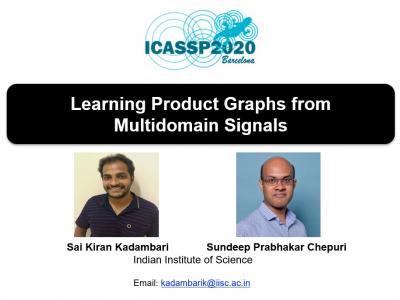
- Citation Author(s):
- Submitted by:
- Sai Kiran Kadambari
- Last updated:
- 14 May 2020 - 7:38pm
- Document Type:
- Presentation Slides
- Document Year:
- 2020
- Event:
- Presenters:
- Kadambari Sai Kiran
- Paper Code:
- SPTM-P3.8
- Categories:
- Log in to post comments
In this paper, we focus on learning the underlying product graph structure from multidomain training data. We assume that the product graph is formed from a Cartesian graph product of two smaller factor graphs. We then pose the product graph learning problem as the factor graph Laplacian matrix estimation problem. To estimate the factor graph Laplacian matrices, we assume that the data is smooth with respect to the underlying product graph. When the training data is noise free or complete, learning factor graphs can be formulated as a convex optimization problem, which has an explicit solution based on the water-filling algorithm. The developed framework is illustrated using numerical experiments on synthetic data as well as real data related to air quality monitoring in India.