Documents
Presentation Slides
LEARNING FROM TAXONOMY: MULTI-LABEL FEW-SHOT CLASSIFICATION FOR EVERYDAY SOUND RECOGNITION
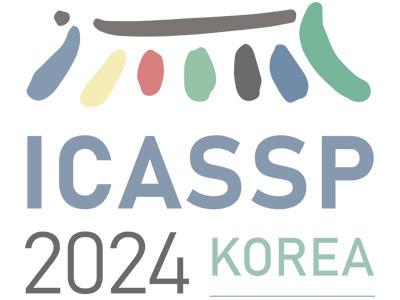
- DOI:
- 10.60864/kcqv-hz86
- Citation Author(s):
- Submitted by:
- Jinhua Liang
- Last updated:
- 6 June 2024 - 10:27am
- Document Type:
- Presentation Slides
- Event:
- Presenters:
- Jinhua Liang
- Paper Code:
- AASP-L4.1
- Categories:
- Log in to post comments
Humans categorise and structure perceived acoustic signals into hierarchies of auditory objects. The semantics of these objects are thus informative in sound classification, especially in few-shot scenarios. However, existing works have only represented audio semantics as binary labels (e.g., whether a recording contains \textit{dog barking} or not), and thus failed to learn a more generic semantic relationship among labels. In this work, we introduce an ontology-aware framework to train multi-label few-shot audio networks with both relative and absolute relationships in an audio taxonomy. Specifically, we propose label-dependent prototypical networks (LaD-ProtoNet) to learn coarse-to-fine acoustic patterns by exploiting direct connections between parent and children classes of sound events. We also present a label smoothing method to take into account the taxonomic knowledge by taking into account absolute distance between two labels w.r.t the taxonomy. For evaluation in a real-world setting, we curate a new dataset, namely FSD-FS, based on the FSD50K dataset and compare the proposed methods and other few-shot classifiers using this dataset. Experiments demonstrate that the proposed method outperforms non-ontology-based methods on the FSD-FS dataset.
Comments
presentation slides
presentation slides